DuSAG: An Anomaly Detection Method in Dynamic Graph Based on Dual Self-attention
ARTIFICIAL NEURAL NETWORKS AND MACHINE LEARNING - ICANN 2022, PT I(2022)
摘要
Anomaly detection of dynamic graphs, graph stream composed of different graphs, has a wide range of applications. The existing anomaly detection methods of dynamic graph based on random walk did not focus on the important vertices in random walks and did not utilize previous states of vertices, and hence, the extracted structural and temporal features are limited. This paper introduces DuSAG which is a dual self-attention anomaly detection algorithm. DuSAG uses structural self-attention to focus on important vertices, and uses temporal self-attention to utilize the previous and last states of vertices, which improves the ability of structural and temporal features extraction and the ability of anomaly detection. We conducted experiments on three real-world datasets, and the results show that DuSAG outperform the state-of-the-art method.
更多查看译文
关键词
Anomaly detection, Dynamic graph, Dual self-attention, Deep learning
AI 理解论文
溯源树
样例
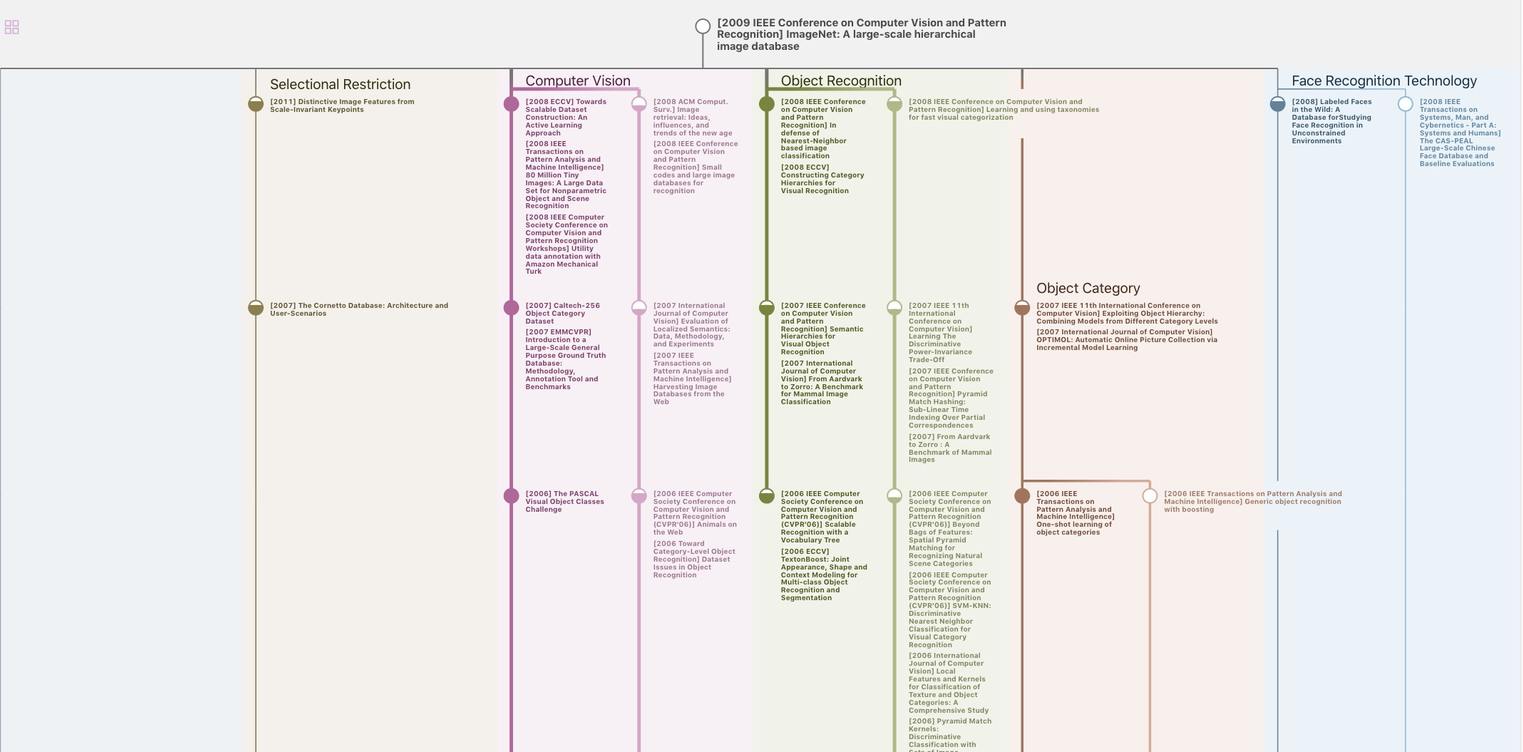
生成溯源树,研究论文发展脉络
Chat Paper
正在生成论文摘要