Rethinking Fall Detection With Wi-Fi
IEEE Transactions on Mobile Computing(2023)
摘要
The past decades have witnessed a surge in human fall detection with sensors, cameras, and wireless signals. Among them, Wi-Fi-based fall detection has been one of the most attractive solutions due to the ubiquitous and pervasive deployment of Wi-Fi infrastructures. However, these approaches are still difficult to be put into practical use. To push forward Wi-Fi-based fall detection for wide deployment, three major limitations concerning
environmental diversity
,
motion diversity
, and
user diversity
are required to be resolved. In this paper, we propose FallDar, a Wi-Fi-based deep learning-assisted fall detection system that outperforms state-of-the-art works on the three criteria simultaneously. First, to deal with environmental diversity, FallDar characterizes falls with the speed of the body, which is the most relevant and inherent feature of falling activities, making the system resilient to environmental changes. Second, to deal with motion diversity, FallDar simulates a large amount of fall data of various falling types with a DNN-based generative model. Training with these data, FallDar is endowed the capability of detecting more types of falls. Third, to deal with user diversity, FallDar proposes to incorporate the fall detection network with a user identification network. The network is designed to extract user-independent features, requiring no fall data from new users for system adjustment. We implement FallDar on commercial Wi-Fi devices and conduct experiments in home and office environments for six months. The evaluation results show that FallDar achieves a false alarm rate of 5.7% and a missed alarm rate of 3.4% across all factors, making a fundamental step towards ubiquitous fall detection with Wi-Fi.
更多查看译文
关键词
Fall detection, Wireless fidelity, Feature extraction, Mobile computing, Training, Radar, Performance evaluation, channel state information (CSI), commodity Wi-Fi
AI 理解论文
溯源树
样例
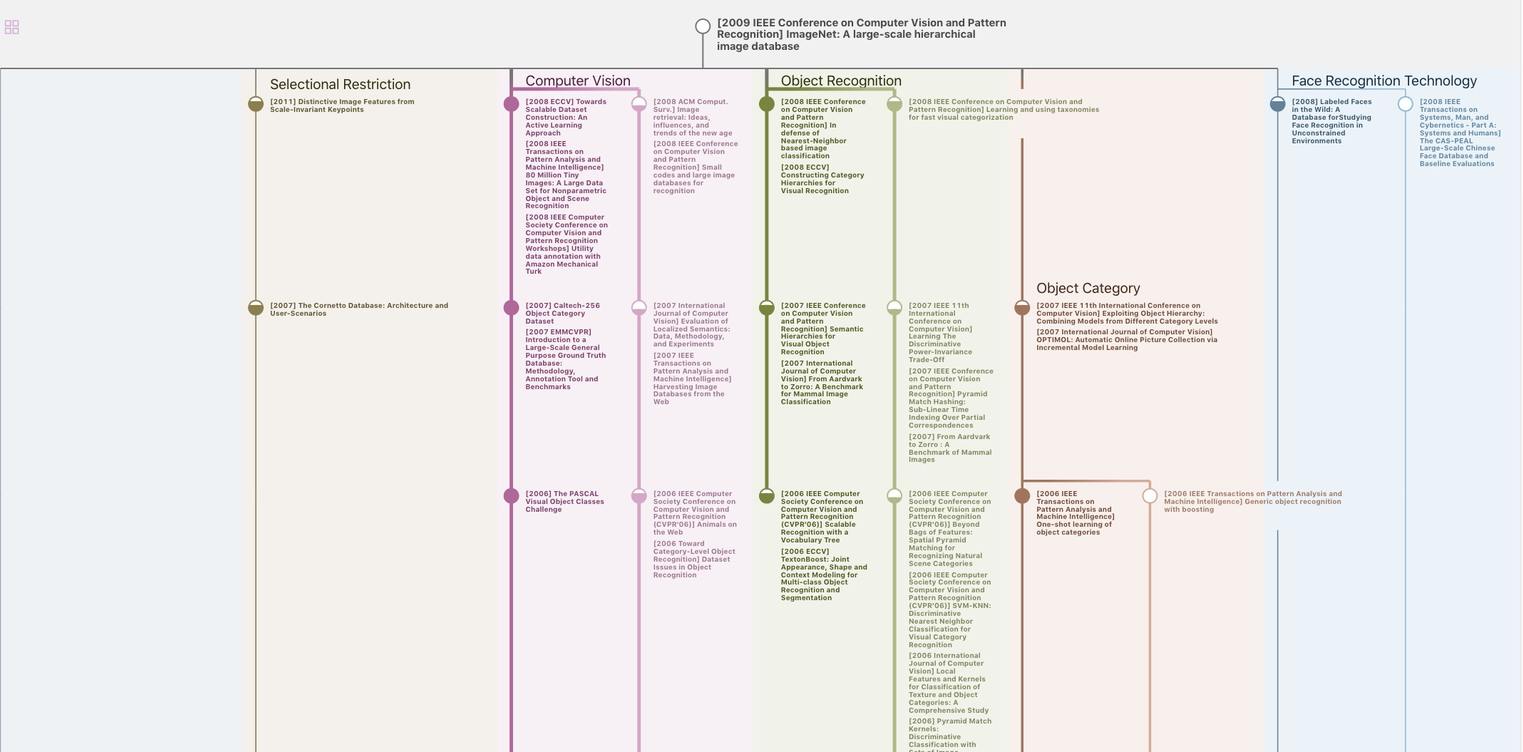
生成溯源树,研究论文发展脉络
Chat Paper
正在生成论文摘要