Toward Systems Models for Obesity Prevention: A Big Role for Big Data
CURRENT DEVELOPMENTS IN NUTRITION(2022)
摘要
Teaser Text: Using large volumes and varieties of data-"big data"-can help to study the complex factors influencing obesity. This article explores the roles for big data in obesity research. The relation among the various causal factors of obesity is not well understood, and there remains a lack of viable data to advance integrated, systems models of its etiology. The collection of big data has begun to allow the exploration of causal associations between behavior, built environment, and obesity-relevant health outcomes. Here, the traditional epidemiologic and emerging big data approaches used in obesity research are compared, describing the research questions, needs, and outcomes of 3 broad research domains: eating behavior, social food environments, and the built environment. Taking tangible steps at the intersection of these domains, the recent European Union project "BigO: Big data against childhood obesity" used a mobile health tool to link objective measurements of health, physical activity, and the built environment. BigO provided learning on the limitations of big data, such as privacy concerns, study sampling, and the balancing of epidemiologic domain expertise with the required technical expertise. Adopting big data approaches will facilitate the exploitation of data concerning obesity-relevant behaviors of a greater variety, which are also processed at speed, facilitated by mobile-based data collection and monitoring systems, citizen science, and artificial intelligence. These approaches will allow the field to expand from causal inference to more complex, systems-level predictive models, stimulating ambitious and effective policy interventions.
更多查看译文
关键词
behavior, built environment, big data, childhood obesity, monitoring systems, physical activity, systems models
AI 理解论文
溯源树
样例
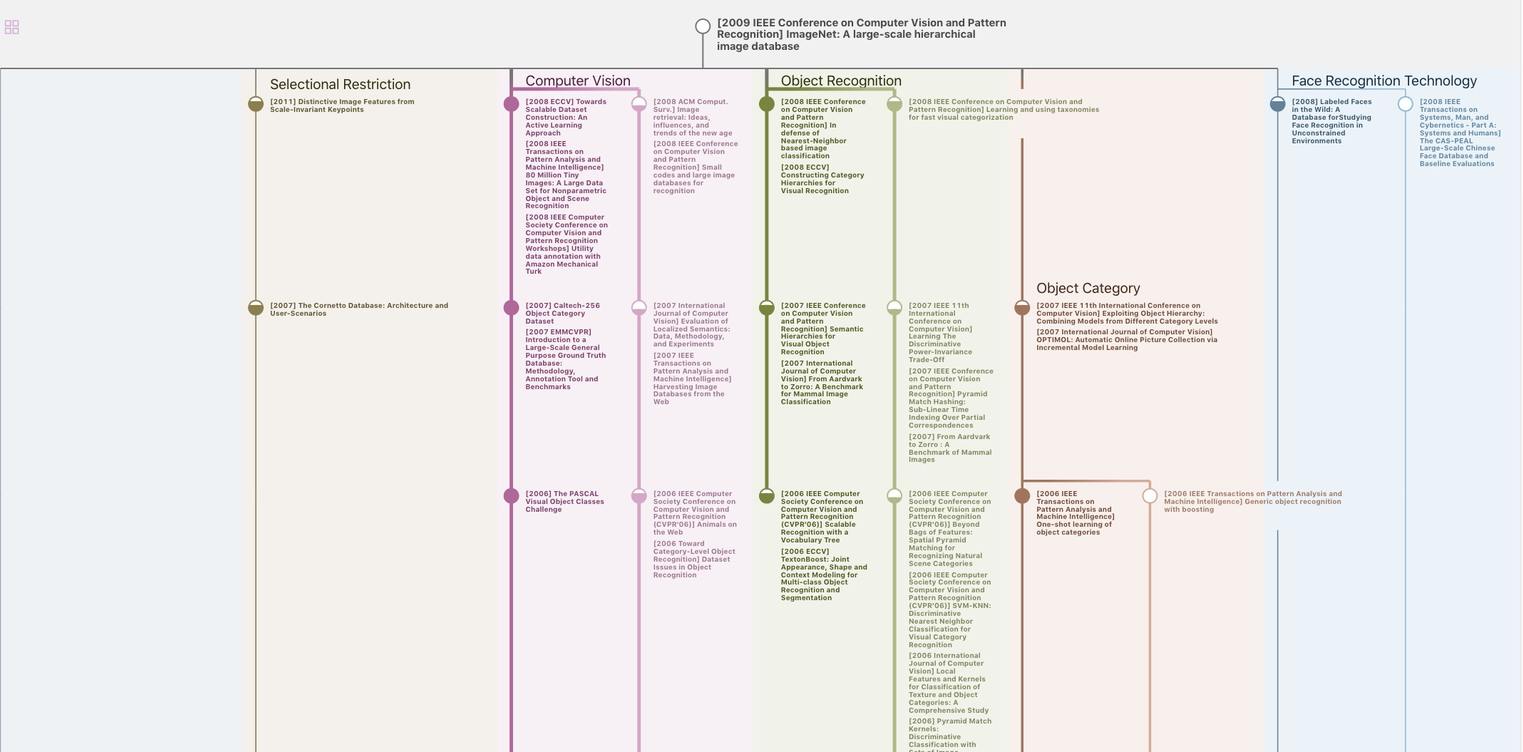
生成溯源树,研究论文发展脉络
Chat Paper
正在生成论文摘要