Surrogate Neural Network for Rapid Flight Performance Evaluation of Hybrid Rocket Engines
JOURNAL OF SPACECRAFT AND ROCKETS(2022)
摘要
This paper investigates the use of neural networks as surrogate models for fast and accurate performance prediction of hybrid rocket engines (HREs). To this aim, a deep feedforward neural network is trained to estimate the thrust and mass flow rate laws of a liquid oxygen/paraffin-wax HRE (as well as other motor characteristics, including the burning time as well as dry and propellant masses), starting from a limited number of motor design parameters, which define the fuel grain, the nozzle geometry, and the initial oxidizer mass flow rate. A medium-fidelity HRE ballistic model, accounting for nozzle throat erosion and two alternative oxidizer feed systems (a pump-fed and a pressure-fed system), is used to generate the sample data the network is trained on. A comprehensive analysis is carried out to assess the neural network accuracy and its generalization capability using several network topologies and varying the size of the training set. Finally, to demonstrate the effectiveness of the proposed approach, the trained network is used as a surrogate HRE model within a multidisciplinary optimization process, which is aimed at determining the optimal HRE design and ascent trajectory for a Vega-derived three-stage launch vehicle, where a HRE replaces the last two stages of the original four-stage rocket.
更多查看译文
关键词
rapid flight performance evaluation,neural network,surrogate
AI 理解论文
溯源树
样例
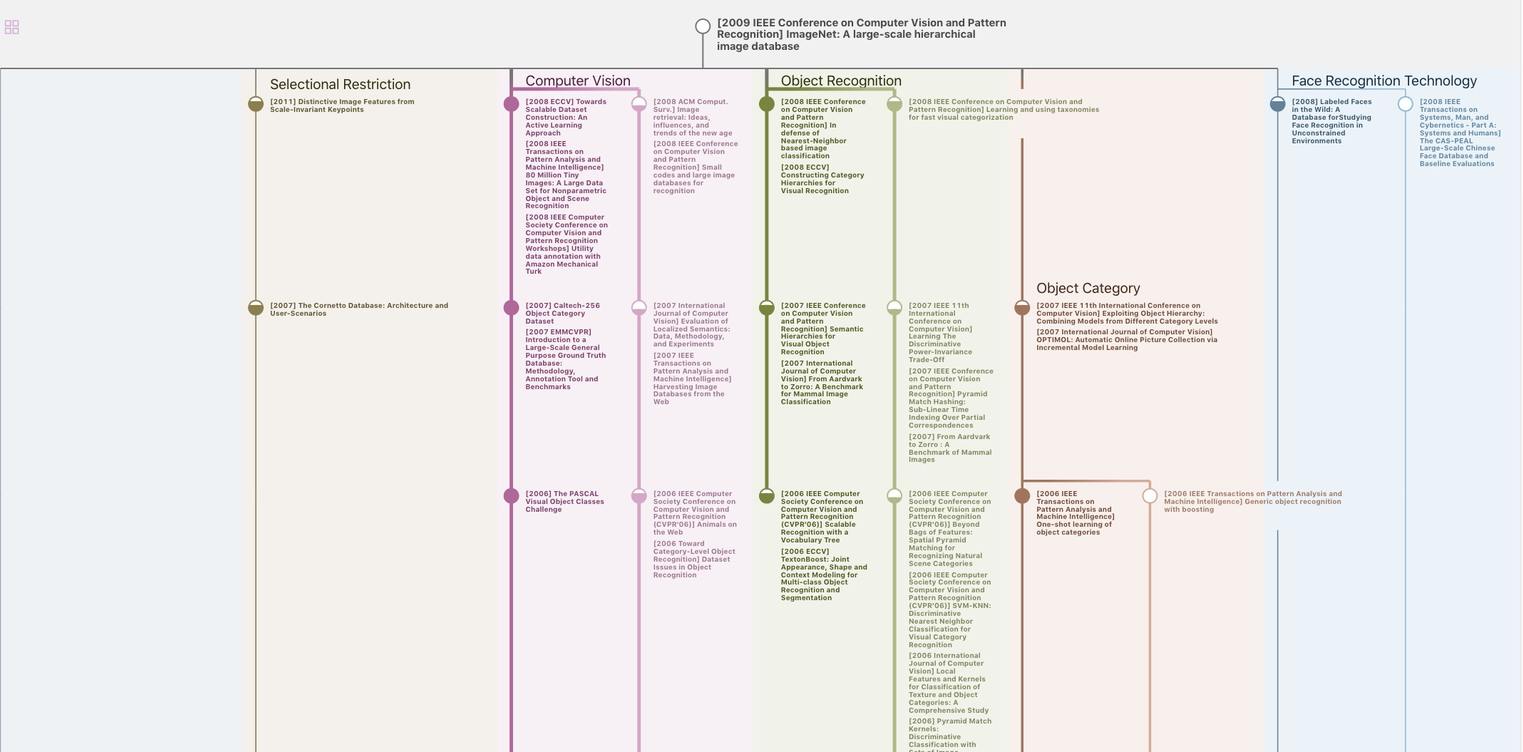
生成溯源树,研究论文发展脉络
Chat Paper
正在生成论文摘要