Human-Guided Motion Planning in Partially Observable Environments
IEEE International Conference on Robotics and Automation(2022)
摘要
Motion planning is a core problem in robotics, with a range of existing methods aimed to address its diverse set of challenges. However, most existing methods rely on complete knowledge of the robot environment; an assumption that seldom holds true due to inherent limitations of robot perception. To enable tractable motion planning for high-DOF robots under partial observability, we introduce BLIND, an algorithm that leverages human guidance. BLIND utilizes inverse reinforcement learning to derive motion-level guidance from human critiques. The algorithm overcomes the computational challenge of reward learning for high-DOF robots by projecting the robot's continuous configuration space to a motion-planner-guided discrete task model. The learned reward is in turn used as guidance to generate robot motion using a novel motion planner. We demonstrate BLIND using the Fetch robot and perform two simulation experiments with partial observability. Our experiments demonstrate that, despite the challenge of partial observability and high dimensionality, BLIND is capable of generating safe robot motion and outperforms baselines on metrics of teaching efficiency, success rate, and path quality.
更多查看译文
关键词
motion-planner-guided discrete task model,learned reward,motion planner,BLIND,Fetch robot,partial observability,safe robot motion,partially observable environments,core problem,robotics,robot environment,robot perception,tractable motion planning,human guidance,motion-level guidance,human critiques,computational challenge,reward learning
AI 理解论文
溯源树
样例
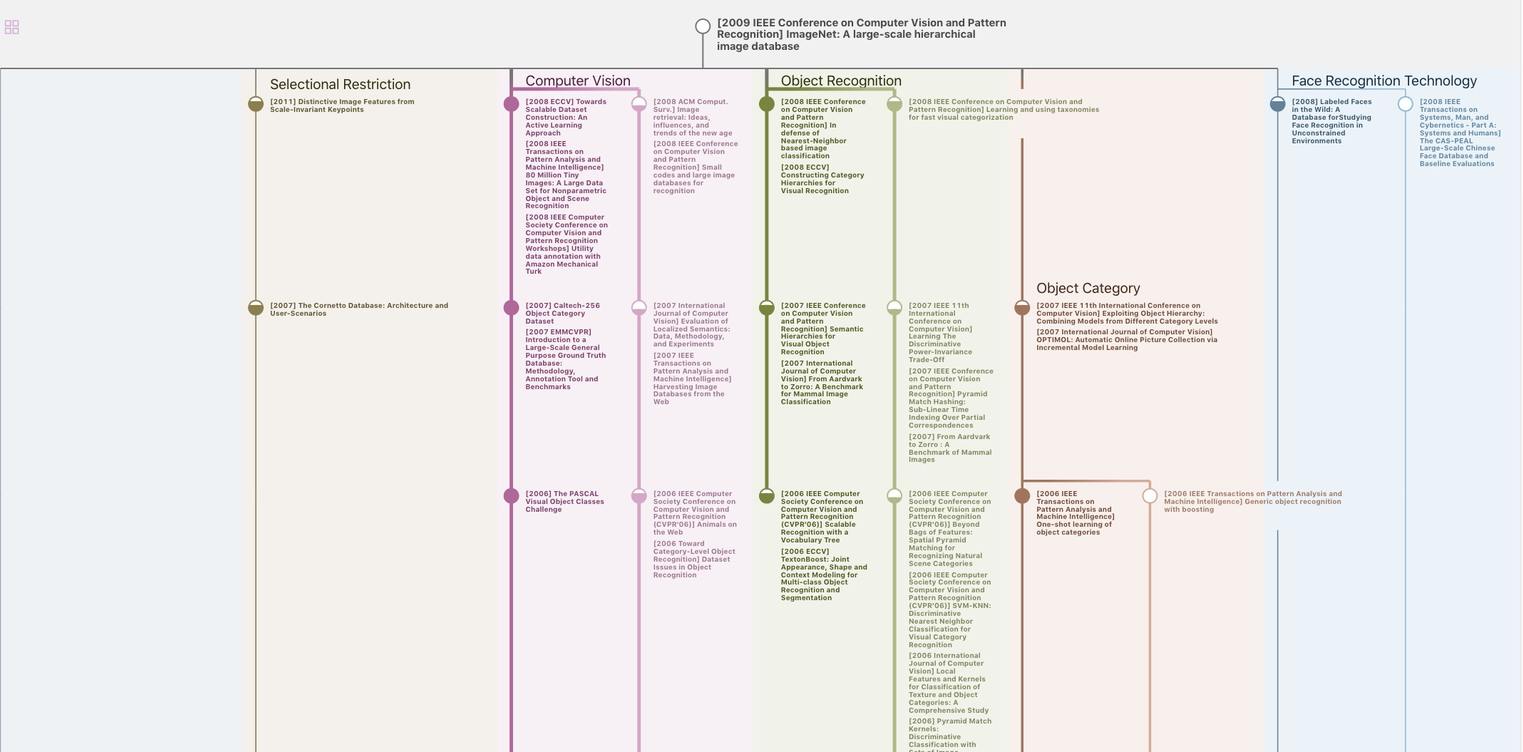
生成溯源树,研究论文发展脉络
Chat Paper
正在生成论文摘要