Perception robustness testing at different levels of generality
Field Robotics(2021)
摘要
Ensuring the success and safety of deployed robotic systems often requires predicting their behavior in a wide range of conditions, precluding practical testing of the physical system itself. We introduce an approach to predict the robustness of a perception system in a broad range of conditions through simulation of challenging real-world conditions achieved by modifying real images according to physical phenomena, producing physically-realistic, mutated images. Typical perception-system performance metrics are difficult to relate to system-level application- or safety-requirements. We propose performance-evaluating metrics that are more closely tied to these targets, a relationship dependent on the amount of application-specific context available and assumptions imposed. We also introduce a novel robustness metric, Robustness ROC, that can be applied to any of these performance metrics. We demonstrate this method on NREC-AgPD, a large dataset focused on person detection for off-road driving in orchard environments, assessing several recent person detectors, and showing how considerations of robustness can often change the best-choice algorithm.
更多查看译文
关键词
perception,testing
AI 理解论文
溯源树
样例
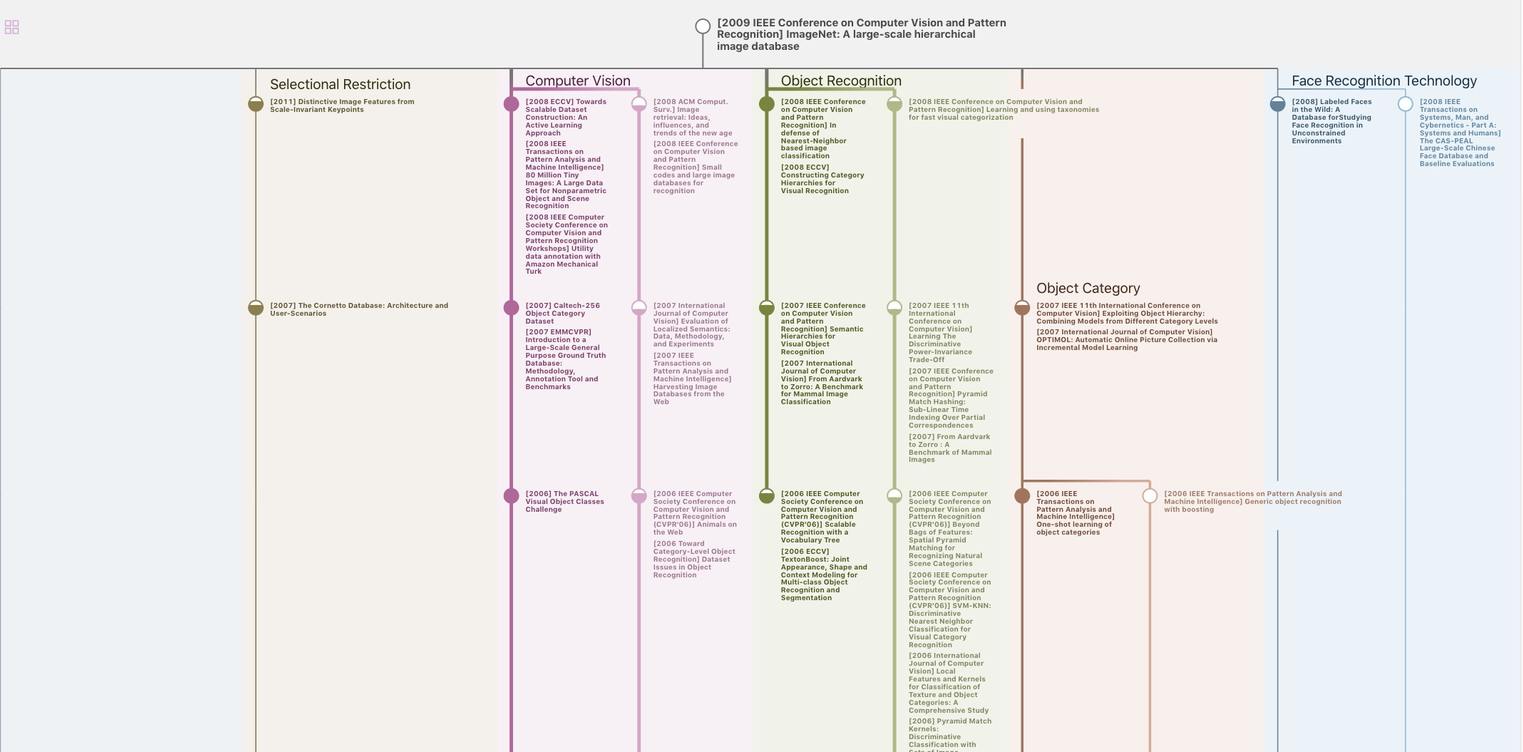
生成溯源树,研究论文发展脉络
Chat Paper
正在生成论文摘要