Pseudo Strong Labels for Large Scale Weakly Supervised Audio Tagging
ICASSP 2022 - 2022 IEEE International Conference on Acoustics, Speech and Signal Processing (ICASSP)(2022)
摘要
Large-scale audio tagging datasets inevitably contain imperfect labels, such as clip-wise annotated (temporally weak) tags with no exact on- and offsets, due to a high manual labeling cost. This work proposes pseudo strong labels (PSL), a simple label augmentation framework that enhances the supervision quality for large-scale weakly supervised audio tagging. A machine annotator is first trained on a large weakly supervised dataset, which then provides finer supervision for a student model. Using PSL we achieve an mAP of 35.95 balanced train subsets of Audioset using a MobileNetV2 backend, significantly outperforming approaches without PSL. An analysis is provided which reveals that PSL mitigates missing labels. Lastly, we show that models trained with PSL are also superior at generalizing to the Freesound datasets (FSD) than their weakly trained counterparts.
更多查看译文
关键词
Relabeling,Audio tagging,Convolutional neural networks,Label augmentation
AI 理解论文
溯源树
样例
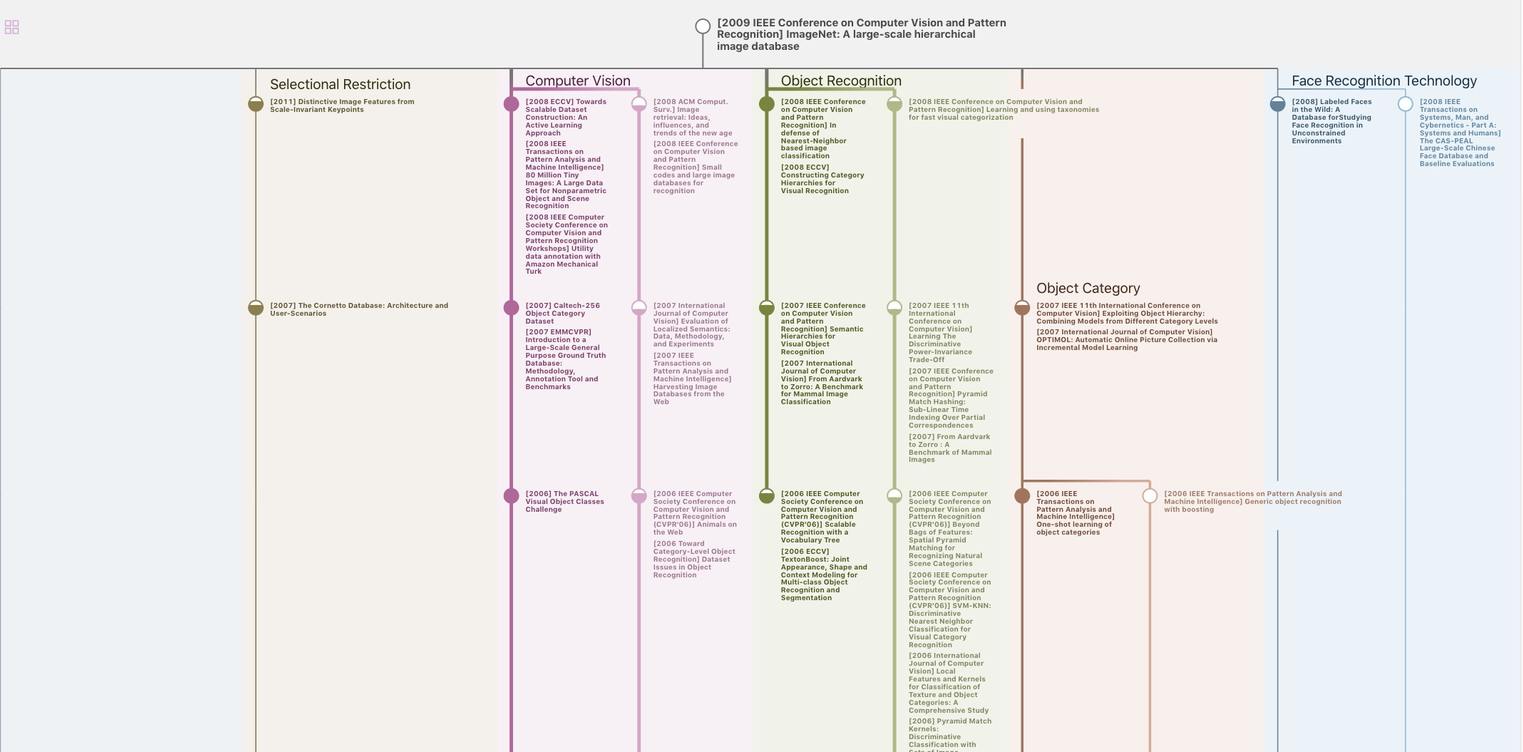
生成溯源树,研究论文发展脉络
Chat Paper
正在生成论文摘要