Machine learning for knowledge acquisition and accelerated inverse-design for non-Hermitian systems
arxiv(2023)
摘要
Non-Hermitian systems offer new platforms for unusual physical properties that can be flexibly manipulated by redistribution of the real and imaginary parts of refractive indices, whose presence breaks conventional wave propagation symmetries, leading to asymmetric reflection and symmetric transmission with respect to the wave propagation direction. Here, we use supervised and unsupervised learning techniques for knowledge acquisition in non-Hermitian systems which accelerate the inverse design process. In particular, we construct a deep learning model that relates the transmission and asymmetric reflection in non-conservative settings and propose sub-manifold learning to recognize non-Hermitian features from transmission spectra. The developed deep learning framework determines the feasibility of a desired spectral response for a given structure and uncovers the role of effective gain-loss parameters to tailor the spectral response. These findings offer a route for intelligent inverse design and contribute to the understanding of physical mechanism in general non-Hermitian systems.
更多查看译文
关键词
Micro-optics,Nanophotonics and plasmonics,Physics,general
AI 理解论文
溯源树
样例
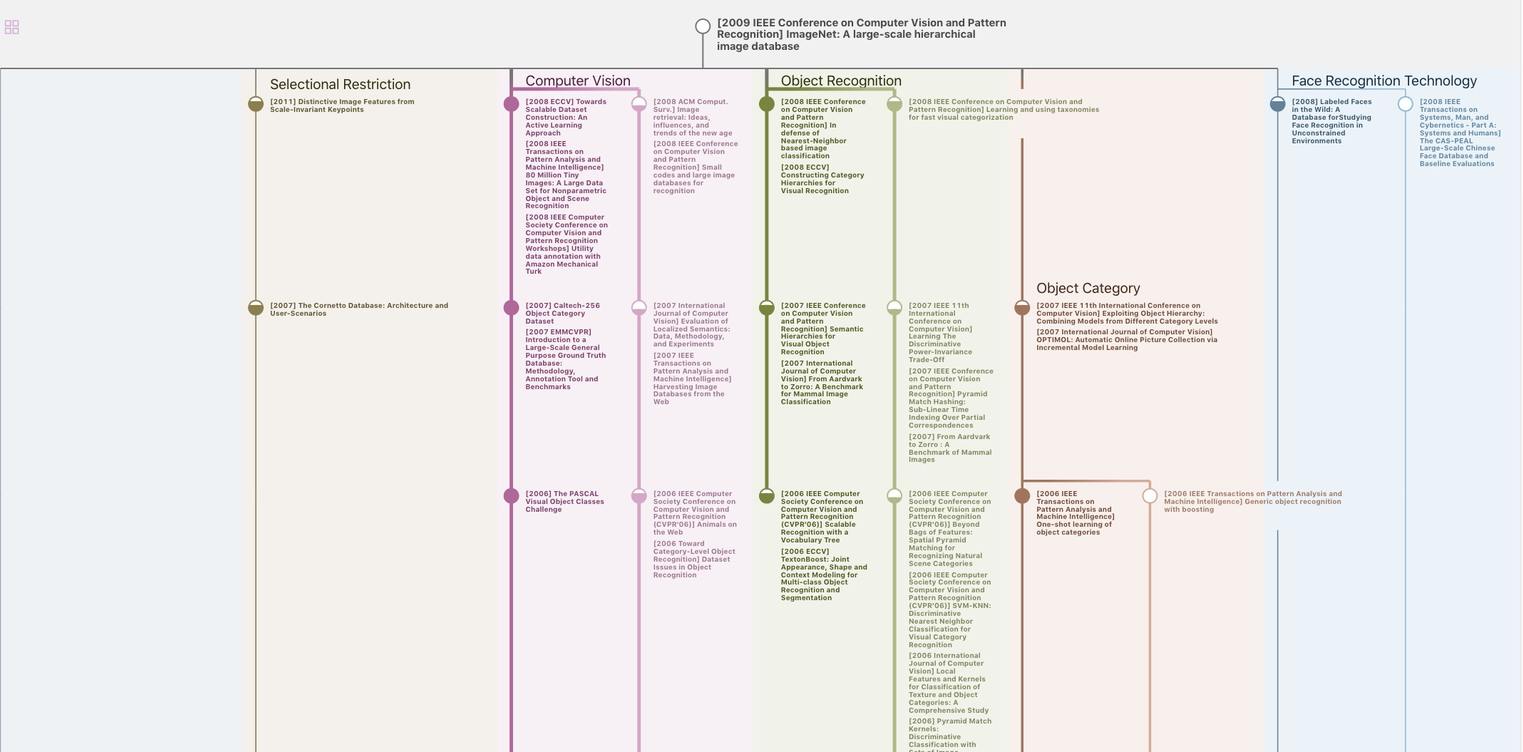
生成溯源树,研究论文发展脉络
Chat Paper
正在生成论文摘要