Feature aggregation graph convolutional network based on imaging genetic data for diagnosis and pathogeny identification of Alzheimer's disease
BRIEFINGS IN BIOINFORMATICS(2022)
Abstract
The roles of brain regions activities and gene expressions in the development of Alzheimer's disease (AD) remain unclear. Existing imaging genetic studies usually has the problem of inefficiency and inadequate fusion of data. This study proposes a novel deep learning method to efficiently capture the development pattern of AD. First, we model the interaction between brain regions and genes as node-to-node feature aggregation in a brain region-gene network. Second, we propose a feature aggregation graph convolutional network (FAGCN) to transmit and update the node feature. Compared with the trivial graph convolutional procedure, we replace the input from the adjacency matrix with a weight matrix based on correlation analysis and consider common neighbor similarity to discover broader associations of nodes. Finally, we use a full-gradient saliency graph mechanism to score and extract the pathogenetic brain regions and risk genes. According to the results, FAGCN achieved the best performance among both traditional and cutting-edge methods and extracted AD-related brain regions and genes, providing theoretical and methodological support for the research of related diseases.
MoreTranslated text
Key words
imaging genetics, Alzheimer's disease, deep learning, feature aggregation graph convolutional network
AI Read Science
Must-Reading Tree
Example
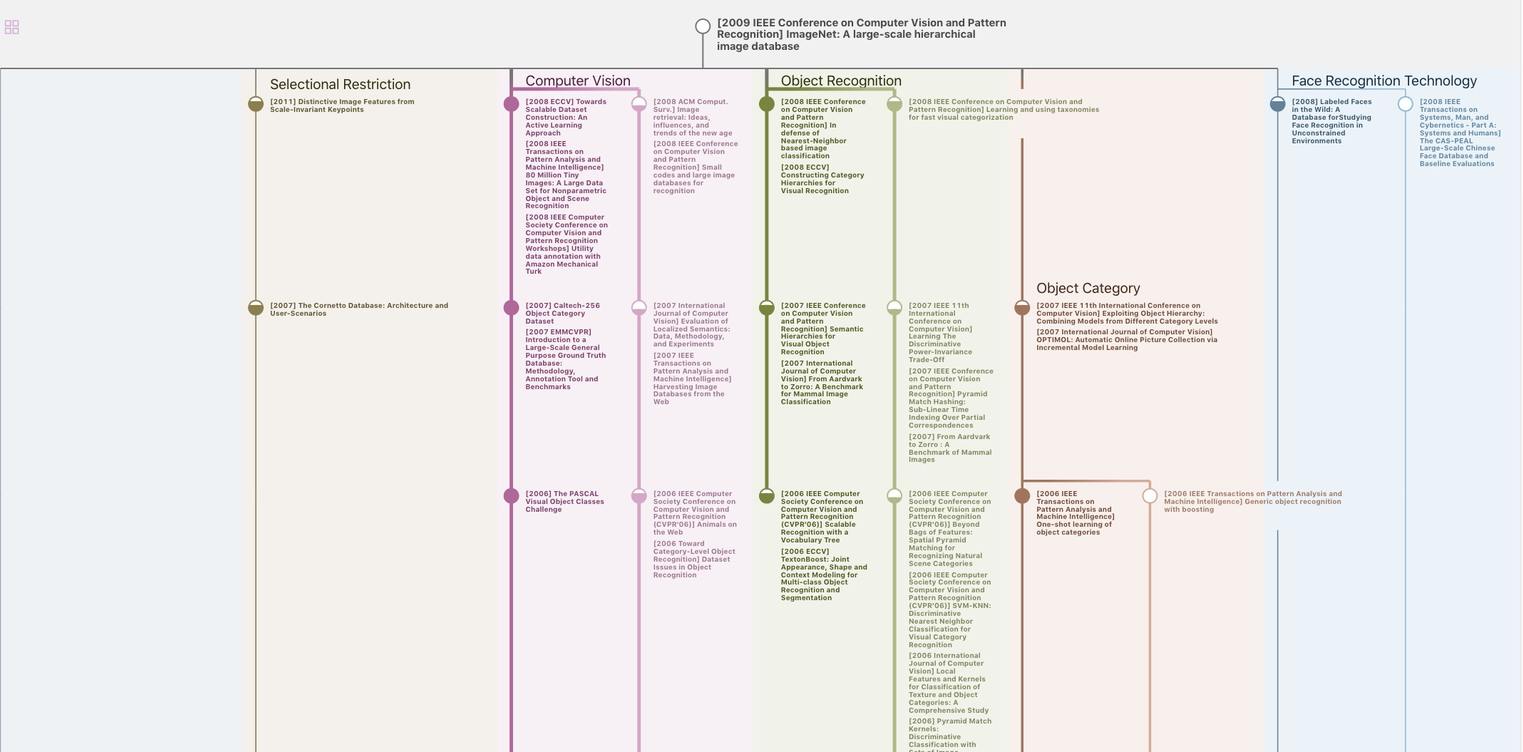
Generate MRT to find the research sequence of this paper
Chat Paper
Summary is being generated by the instructions you defined