SMIV: A 16-nm 25-mm² SoC for IoT With Arm Cortex-A53, eFPGA, and Coherent Accelerators
IEEE Journal of Solid-State Circuits(2022)
摘要
Emerging Internet of Things (IoT) devices necessitate system-on-chips (SoCs) that can scale from ultralow power always-on (AON) operation, all the way up to less frequent high-performance tasks at high energy efficiency. Specialized accelerators are essential to help meet these needs at both ends of the scale, but maintaining workload flexibility remains an important goal. This article presents a 25-mm
2
SoC in 16-nm FinFET technology which demonstrates targeted, flexible acceleration of key compute-intensive kernels spanning machine learning (ML), DSP, and cryptography. The
SMIV
SoC includes a dedicated AON sub-system, a dual-core Arm Cortex-A53 CPU cluster, an SoC-attached embedded field-programmable gate array (eFPGA) array, and a quad-core cache-coherent accelerator (CCA) cluster. Measurement results demonstrate: 1) 1236
$\times $
power envelope, from 1.1 mW (only AON cluster), up to 1.36 W (whole SoC at maximum throughput); 2) 5.5–28.9
$\times $
energy efficiency gain from offloading compute kernels from A53 to eFPGA; 3) 2.94
$\times $
latency improvement using coherent memory access (CCA cluster); and 4) 55
$\times $
MobileNetV1 energy per inference improvement on CCA compared to the CPU baseline. The overall flexibility-efficiency range on SMIV spans measured energy efficiencies of 1
$\times $
(dual-core A53), 3.1
$\times $
(A53 with SIMD), 16.5
$\times $
(eFPGA), 54.9
$\times $
(CCA), and 256
$\times $
(AON) at a peak efficiency of 4.8 TOPS/W.
更多查看译文
关键词
Deep neural networks (DNNs),embedded field-programmable gate array (eFPGA),hardware accelerators,Internet of Things (IoT),machine learning (ML),system-on-chip (SoC)
AI 理解论文
溯源树
样例
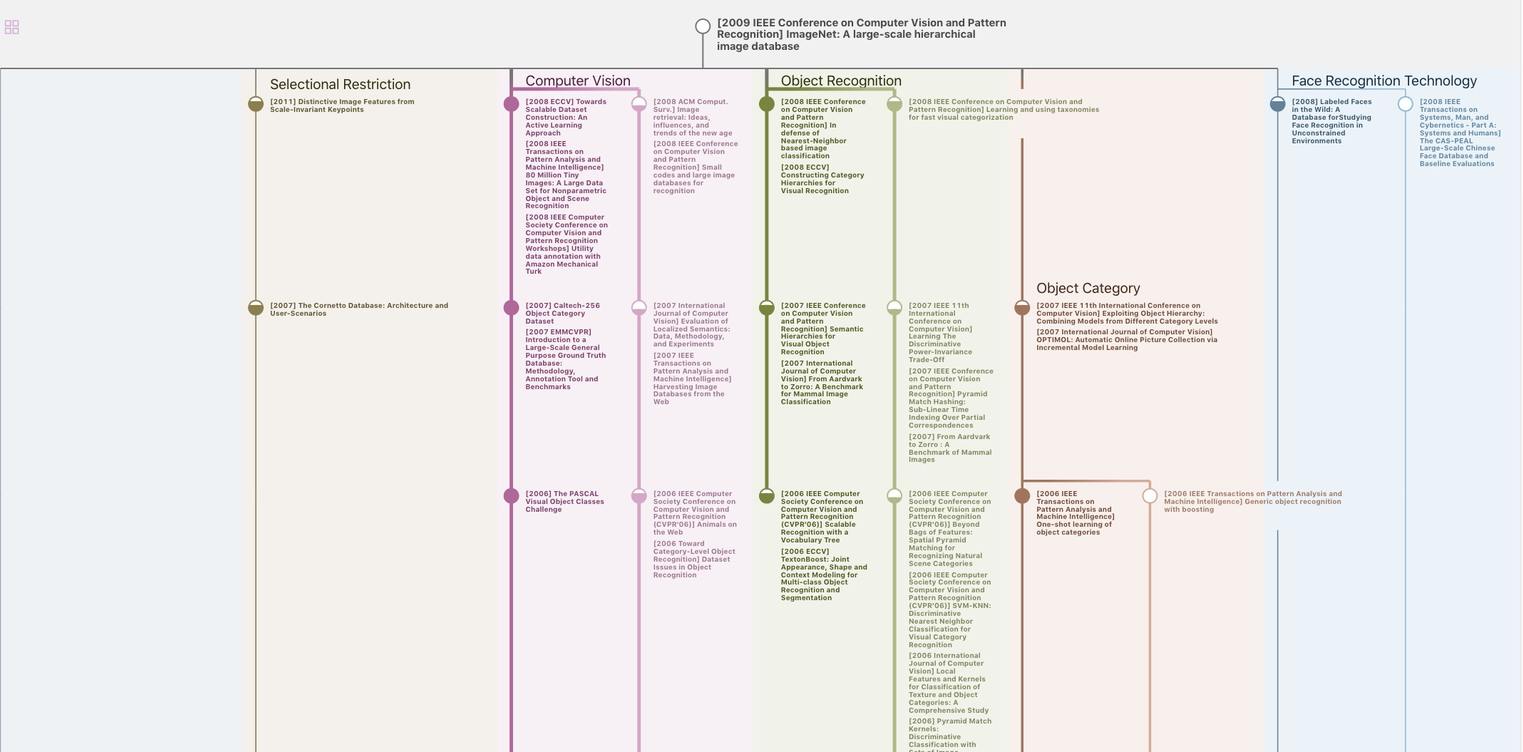
生成溯源树,研究论文发展脉络
Chat Paper
正在生成论文摘要