Detection Of Cellular Spikes And Classification Of Cells From Raw Nanoscale Biosensor Data
PROCEEDINGS OF ELM-2016(2018)
摘要
Nanoscale devices have provided promising endeavors for detecting crucial biomarkers such as DNA, proteins, and human cells at a finer scale. These biomarkers can improve prognosis by detecting dreadful disease such as cancer at an early stage than the current approaches. Analyzing raw data from these nanoscale devices for disease detection is tedious as the raw data suffers from noise. Furthermore, disease detection decisions are made based on manual or semi-automated analysis-which are time-consuming, monotonous and error-prone process. Recent trends show an unprecedented growth in the advancement of nanotechnology for medical diagnosis. These devices generate huge amount of raw data and analyzing raw data in order to classify biomarkers in a fully automated and robust way is a challenge. In this paper, we present an algorithm for identifying cellular spikes, we have adapted extreme learning machines and dynamic time warping for the classification of cancer in raw data collected from nanoscale biosensors, such as solid-state micropores. Our approach can classify cancer cells with an accuracy of 95.6%, and with a precision and recall of 85.7% and 80.0%, respectively.
更多查看译文
关键词
Cancer cells, Spike detection, Extreme learning machines, Dynamic time warping, Biomarkers, Nanoscale biosensing
AI 理解论文
溯源树
样例
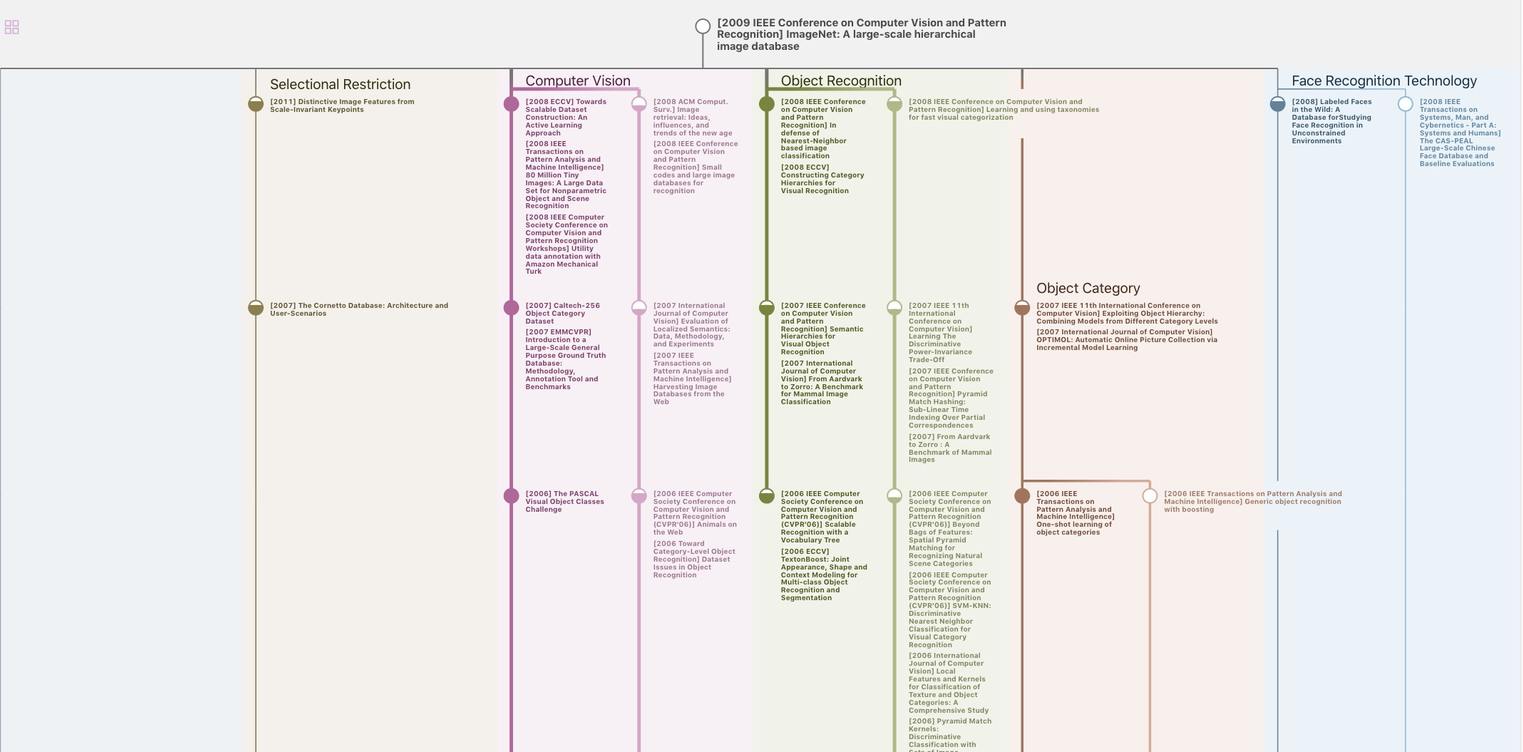
生成溯源树,研究论文发展脉络
Chat Paper
正在生成论文摘要