SLAP: A Supervised Learning Approach for Priority Cuts Technology Mapping
2021 58TH ACM/IEEE DESIGN AUTOMATION CONFERENCE (DAC)(2021)
摘要
Recently we have seen many works that leverage Machine Learning (ML) techniques in optimizing Electronic Design Automation (EDA) process. However, the uses of ML techniques are limited to learning forecasting models of existing EDA algorithms, instead of developing novel algorithms. In this work, we focus on designing an novel cut-based technology mapping algorithms assisted by ML techniques, which matches results of exhaustive cut exploration but preserving a small footprint of utilized cuts. The proposed approach has been demonstrated with a wide range of benchmarks with 24% reductions in number of cuts utilized compared to the state-of-the-art, while improving the circuit delay, and Area-Delay-Product (ADP), by average about 10%, 7%, respectively, with a 2% area penalty. Compared to the exhaustive approach, i.e., considering all the cuts, we achieve similar or better results while saving over than 2x the number of considered cuts (runtime) on average. Finally, we provide a comprehensive explanation of heuristics learned by the ML model by feature ranking.
更多查看译文
关键词
Cut-pruning, Technology Mapping, ASIC design, Machine Learning
AI 理解论文
溯源树
样例
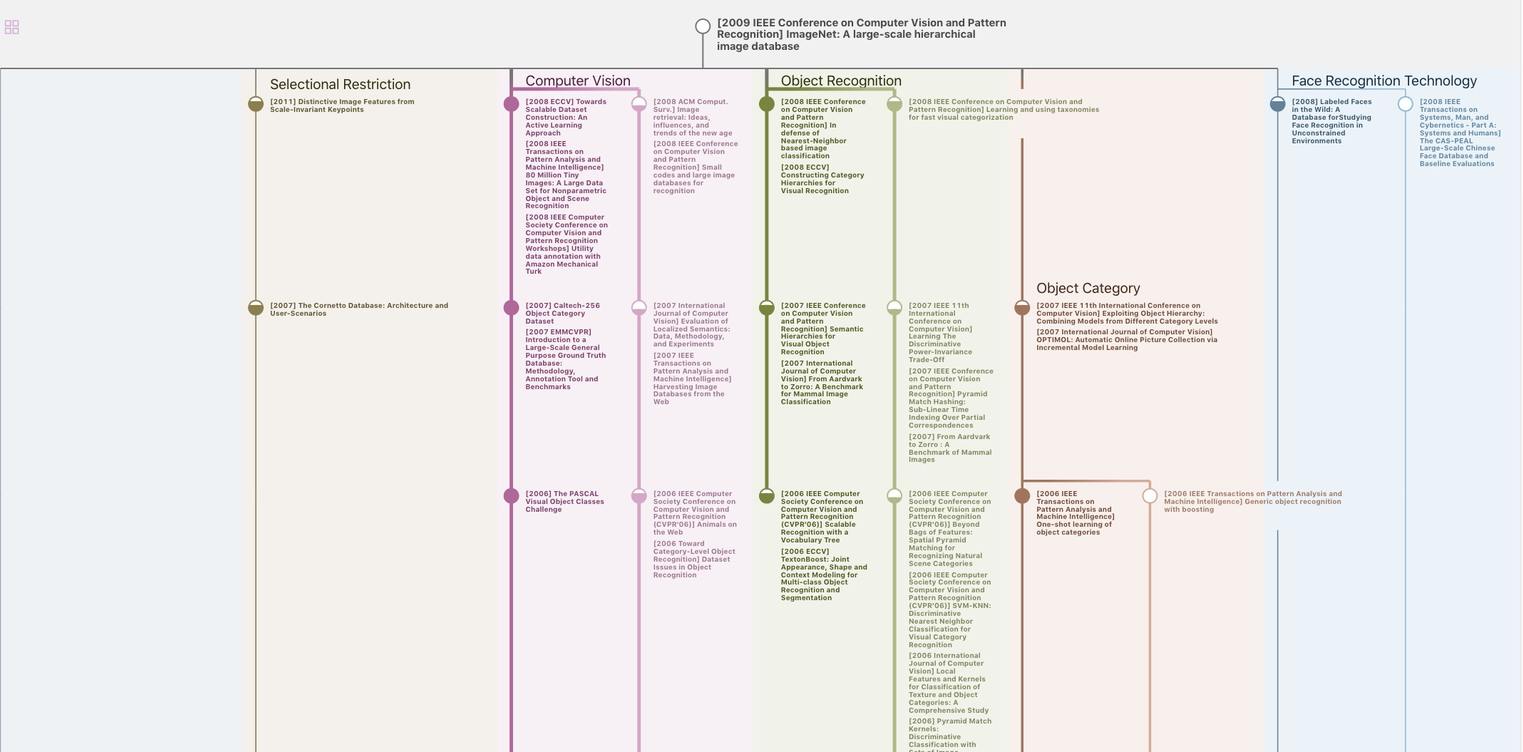
生成溯源树,研究论文发展脉络
Chat Paper
正在生成论文摘要