Data-Based Predictive Control Via Multistep Policy Gradient Reinforcement Learning.
IEEE transactions on cybernetics(2023)
Abstract
In this article, a model-free predictive control algorithm for the real-time system is presented. The algorithm is data driven and is able to improve system performance based on multistep policy gradient reinforcement learning. By learning from the offline dataset and real-time data, the knowledge of system dynamics is avoided in algorithm design and application. Cooperative games of the multiplayer in time horizon are presented to model the predictive control as optimization problems of multiagent and guarantee the optimality of the predictive control policy. In order to implement the algorithm, neural networks are used to approximate the action-state value function and predictive control policy, respectively. The weights are determined by using the methods of weighted residual. Numerical results show the effectiveness of the proposed algorithm.
MoreTranslated text
Key words
Predictive control,Real-time systems,Predictive models,Prediction algorithms,Reinforcement learning,Games,Cost function,Cooperative games,multistep reinforcement learning (RL),policy gradient methods,predictive control
AI Read Science
Must-Reading Tree
Example
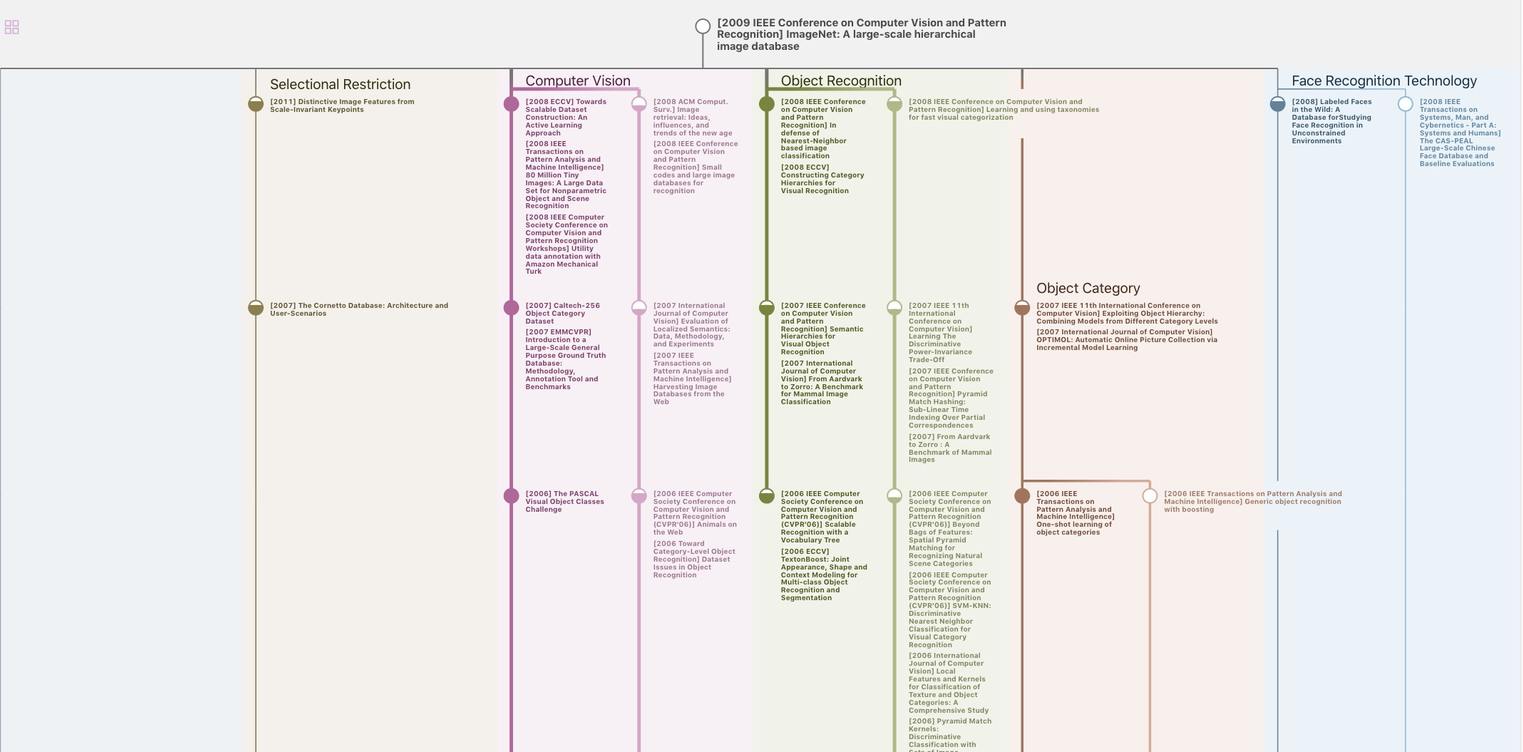
Generate MRT to find the research sequence of this paper
Chat Paper
Summary is being generated by the instructions you defined