Hyperspectral And Lidar Data Applied To The Urban Land Cover Machine Learning And Neural-Network-Based Classification: A Review
REMOTE SENSING(2021)
摘要
Rapid technological advances in airborne hyperspectral and lidar systems paved the way for using machine learning algorithms to map urban environments. Both hyperspectral and lidar systems can discriminate among many significant urban structures and materials properties, which are not recognizable by applying conventional RGB cameras. In most recent years, the fusion of hyperspectral and lidar sensors has overcome challenges related to the limits of active and passive remote sensing systems, providing promising results in urban land cover classification. This paper presents principles and key features for airborne hyperspectral imaging, lidar, and the fusion of those, as well as applications of these for urban land cover classification. In addition, machine learning and deep learning classification algorithms suitable for classifying individual urban classes such as buildings, vegetation, and roads have been reviewed, focusing on extracted features critical for classification of urban surfaces, transferability, dimensionality, and computational expense.
更多查看译文
关键词
machine learning, deep learning, lidar, hyperspectral, remote sensing, urban environment, data fusion, sensor fusion, urban mapping, land cover classification
AI 理解论文
溯源树
样例
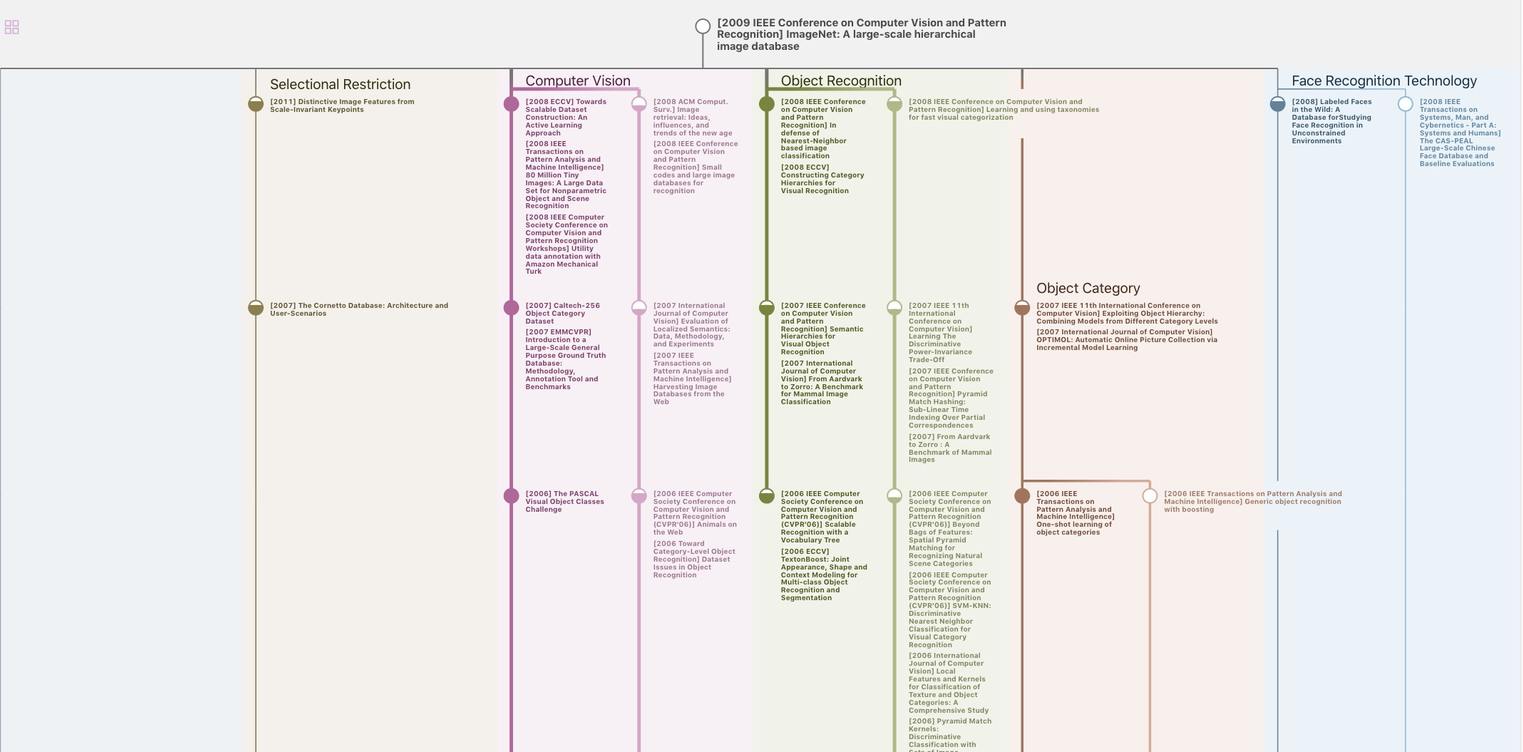
生成溯源树,研究论文发展脉络
Chat Paper
正在生成论文摘要