T-NET: LEARNING FEATURE REPRESENTATION WITH TASK-SPECIFIC SUPERVISION FOR BIOMEDICAL IMAGE ANALYSIS
2021 IEEE 18TH INTERNATIONAL SYMPOSIUM ON BIOMEDICAL IMAGING (ISBI)(2021)
摘要
The encoder-decoder network is widely used to learn deep feature representations from pixel-wise annotations in biomedical image analysis. Under this structure, the performance profoundly relies on the effectiveness of feature extraction achieved by the encoding network. However, few models have considered adapting the attention of the feature extractor even in different kinds of tasks. In this paper, we propose a novel training strategy by adapting the attention of the feature extractor according to different tasks for effective representation learning. Specifically, the framework, named T-Net, consists of an encoding network supervised by task-specific attention maps and a posterior network that takes in the learned features to predict the corresponding results. The attention map is obtained by the transformation from pixel-wise annotations according to the specific task, which is used as the supervision to regularize the feature extractor to focus on different locations of the recognition object. To show the effectiveness of our method, we evaluate T-Net on two different tasks, i.e., segmentation and localization. Extensive results on three public datasets (BraTS-17, MoNuSeg and IDRiD) have indicated the effectiveness and efficiency of our proposed supervision method, especially over the conventional encoding-decoding network.
更多查看译文
关键词
task-specific supervision,biomedical image analysis,encoder-decoder network,deep feature representations,pixel-wise annotations,feature extraction,encoding network,feature extractor,effective representation learning,task-specific attention maps,posterior network,attention map,supervision method,conventional encoding-decoding network,feature representation
AI 理解论文
溯源树
样例
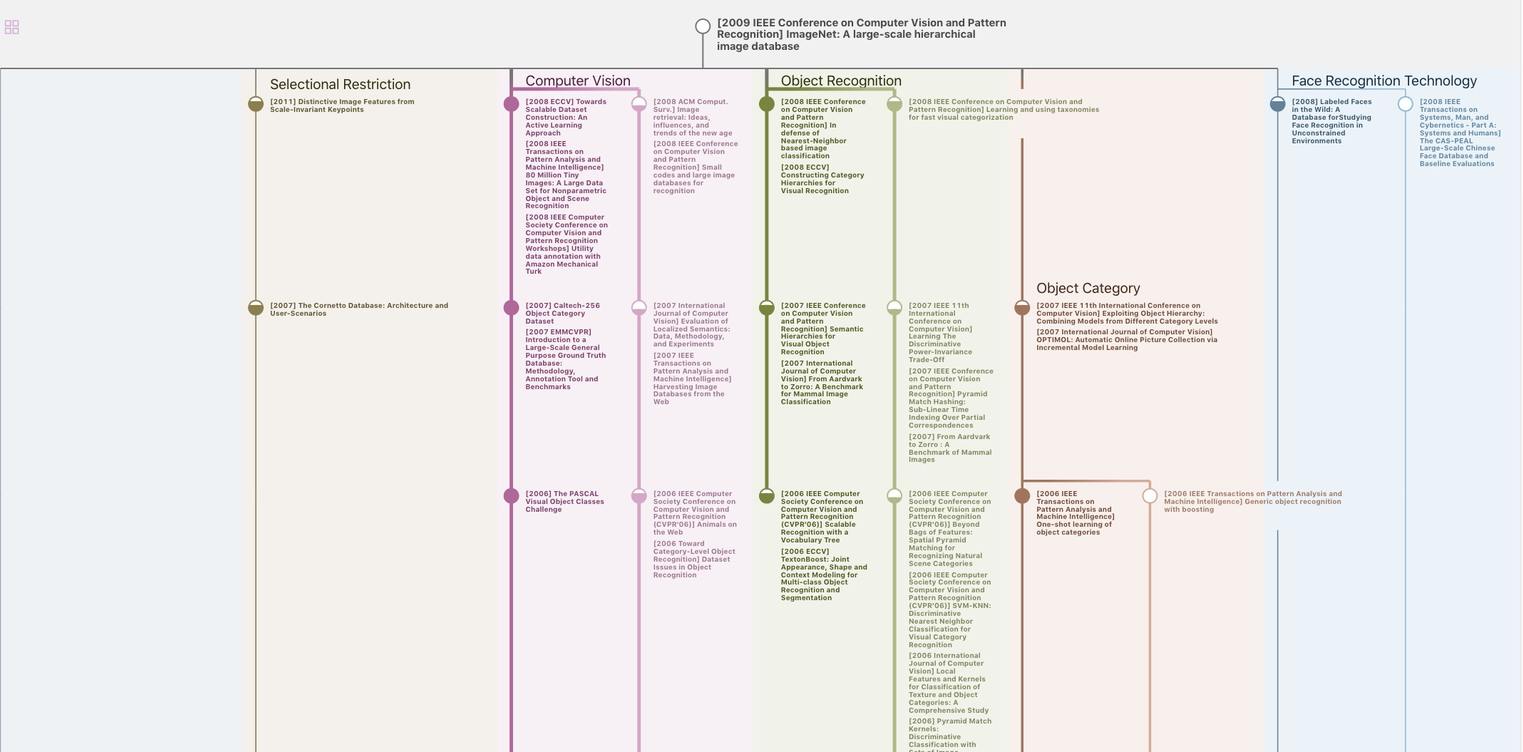
生成溯源树,研究论文发展脉络
Chat Paper
正在生成论文摘要