Privacy-Preserving Localization For Underwater Sensor Networks Via Deep Reinforcement Learning
IEEE TRANSACTIONS ON INFORMATION FORENSICS AND SECURITY(2021)
摘要
Underwater sensor networks (USNs) are envisioned to enable a large variety of marine applications. Such applications require accurate position information of sensor nodes. However, the openness and inhomogeneity characteristics of underwater medium make it much more challenging to solve the localization issue. This paper is concerned with a privacy-preserving localization issue for USNs in inhomogeneous underwater medium. An honest-but-curious model is considered to develop a privacy-preserving localization protocol. Based on this, a localization problem is constructed for sensor nodes to minimize the sum of all measurement errors, where a ray compensation strategy is incorporated to remove the localization bias from assuming the straight-line transmission. To make the above problem tractable, we consider the unsupervised, supervised and semisupervised scenarios, through which deep reinforcement learning (DRL) based localization estimators are utilized to estimate the positions of sensor nodes. It is noted that, the proposed localization solution in this paper can hide the private position information of USNs, and more importantly, it is robust to local optimum for nonconvex and nonsmooth localization problem in inhomogeneous underwater medium. Finally, simulation studies are given to show the position privacy can be preserved, while the localization accuracy can be enhanced as compared with the other existing works.
更多查看译文
关键词
Protocols, Nonhomogeneous media, Encryption, Privacy, Reinforcement learning, Receivers, Water, Privacy preserving, localization, deep reinforcement learning, underwater sensor networks, inhomogeneous
AI 理解论文
溯源树
样例
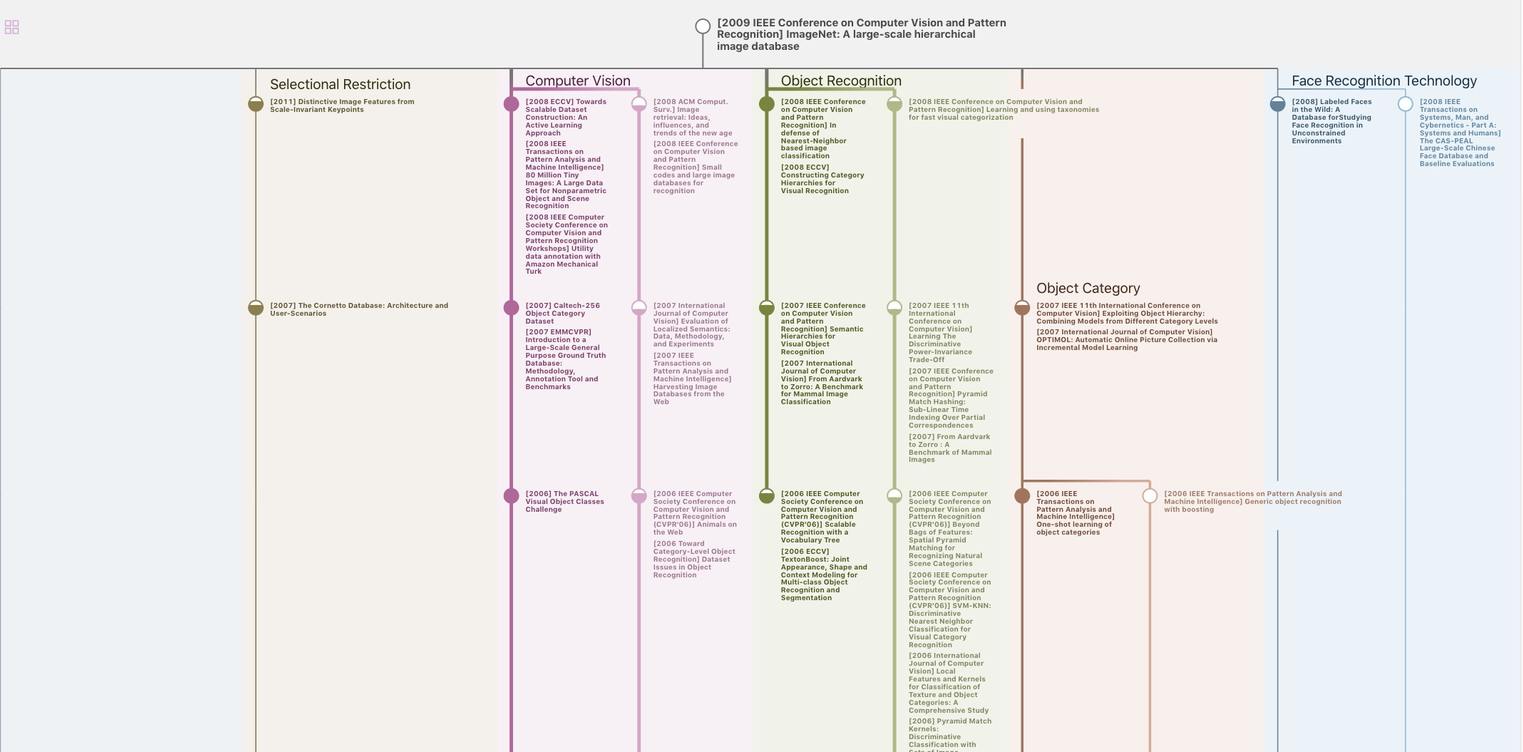
生成溯源树,研究论文发展脉络
Chat Paper
正在生成论文摘要