Neural networks with late-phase weights
ICLR(2021)
摘要
The largely successful method of training neural networks is to learn their weights using some variant of stochastic gradient descent (SGD). Here, we show that the solutions found by SGD can be further improved by ensembling a subset of the weights in late stages of learning. At the end of learning, we obtain back a single model by taking a spatial average in weight space. To avoid incurring increased computational costs, we investigate a family of low-dimensional late-phase weight models which interact multiplicatively with the remaining parameters. Our results show that augmenting standard models with late-phase weights improves generalization in established benchmarks such as CIFAR-10/100, ImageNet and enwik8. These findings are complemented with a theoretical analysis of a noisy quadratic problem which provides a simplified picture of the late phases of neural network learning.
更多查看译文
关键词
neural networks,late-phase
AI 理解论文
溯源树
样例
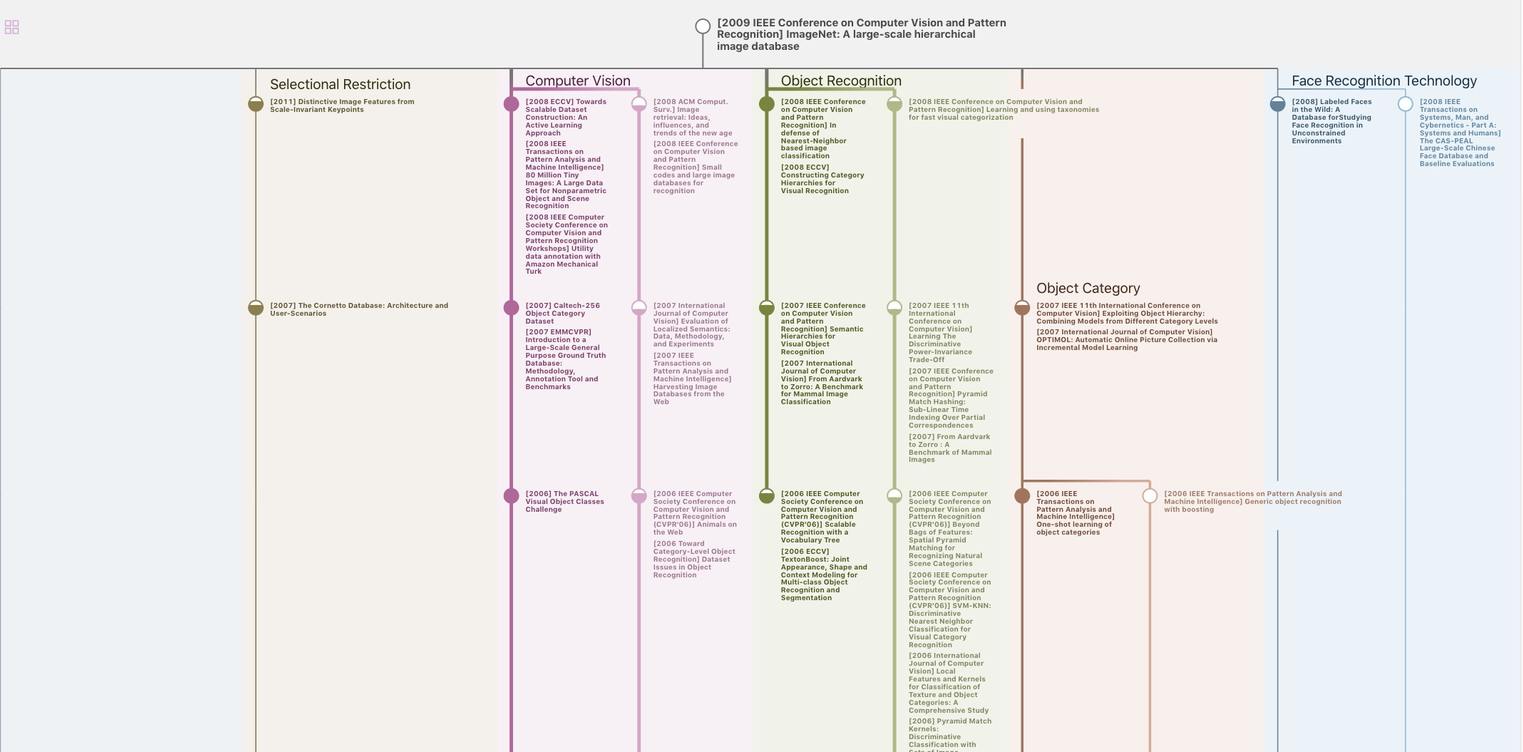
生成溯源树,研究论文发展脉络
Chat Paper
正在生成论文摘要