Unsupervised EEG Cybersickness Prediction with Deep Embedded Self Organizing Map
2020 IEEE 20th International Conference on Bioinformatics and Bioengineering (BIBE)(2020)
摘要
Cybersickness is a situation that may result from the overexpose to computer devices by a person. Symptoms associated with this sickness are dizziness, headache, nausea, or any kind of disoriented motion.Prediction of cybersickness is essential to avoid the aforementioned symptoms and subsequently secure people's health. In this work a Deep Embedded Self Organizing Map that is an unsupervised learning tool is jointly combined with an Auto-encoder implementing a clustering method. The Auto encoder is designed from EEGNET, which is a specialized CNN for EEG prediction, and is tailored to predict EEG based cybersickness. The proposed method is compared to KNN and SOM clustering methods and prediction accuracy was measured with the purity index and NMI. Test results give that KNN predicted cyberscikness by providing Purity equal to 0.9375 and NMI to 0.386519, while SOM had 0.9432 Purity and 0.403901 NMI. Lastly, DESOM provided the most efficient index values and more specifically: 0.96875 purity and 0.42561 NMI.
更多查看译文
关键词
Unsupervised-Learning,Self Organizing Map,Auto-encoder,Cybersickness,EEG
AI 理解论文
溯源树
样例
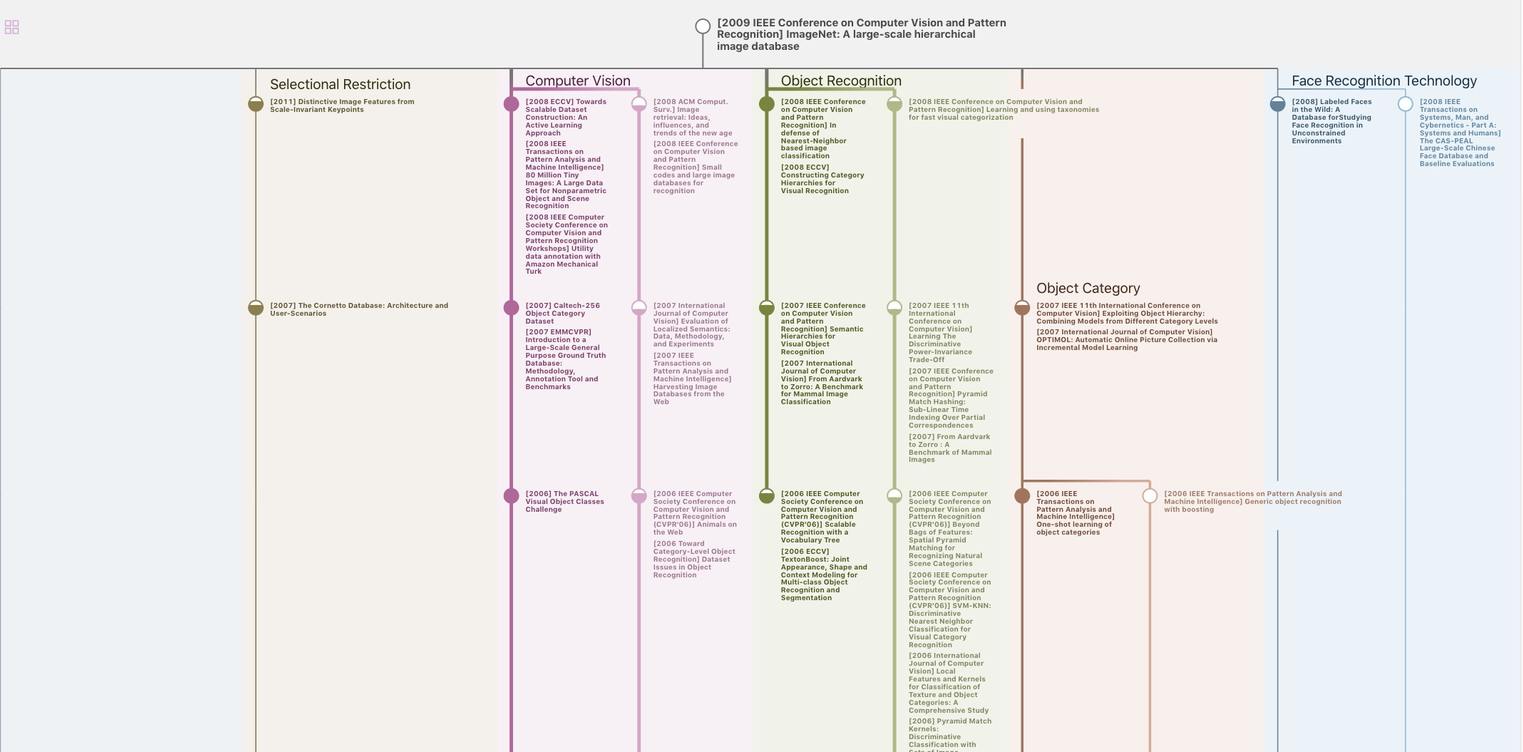
生成溯源树,研究论文发展脉络
Chat Paper
正在生成论文摘要