CoRelatE: Learning the correlation in multi-fold relations for knowledge graph embedding.
Knowl. Based Syst.(2021)
摘要
Existing approaches for knowledge graph embedding usually represent knowledge as triples of binary relations between entities and embed them into continuous vector space, which are not practical for the complex facts in the form of multi-fold relations in real life. In this work, we address the problem of multi-fold relation embedding in knowledge graph and propose a new framework CoRelatE that learns correlations between entities, facts and relations from the instances. We first model the entity-relation correlation directly via combinational operator, then utilize a graph convolutional network to model the correlation between entities and their related facts. Finally, the facts are forced to embed close to their corresponding relations to learn the fact-relation correlation. We formulate the objective as a joint optimization problem and introduce an efficient algorithm to solve it. We compare the proposed CoRelatE model with several state-of-the-art models including HypE, NaLP and RAE, m-TransH. Experimental results on four datasets of multi-fold relations and two datasets of binary relations for link prediction and instance classification tasks validate the effectiveness and merits of our model.
更多查看译文
关键词
Knowledge graph embedding,Representation learning,Multi-fold relation,Correlation learning
AI 理解论文
溯源树
样例
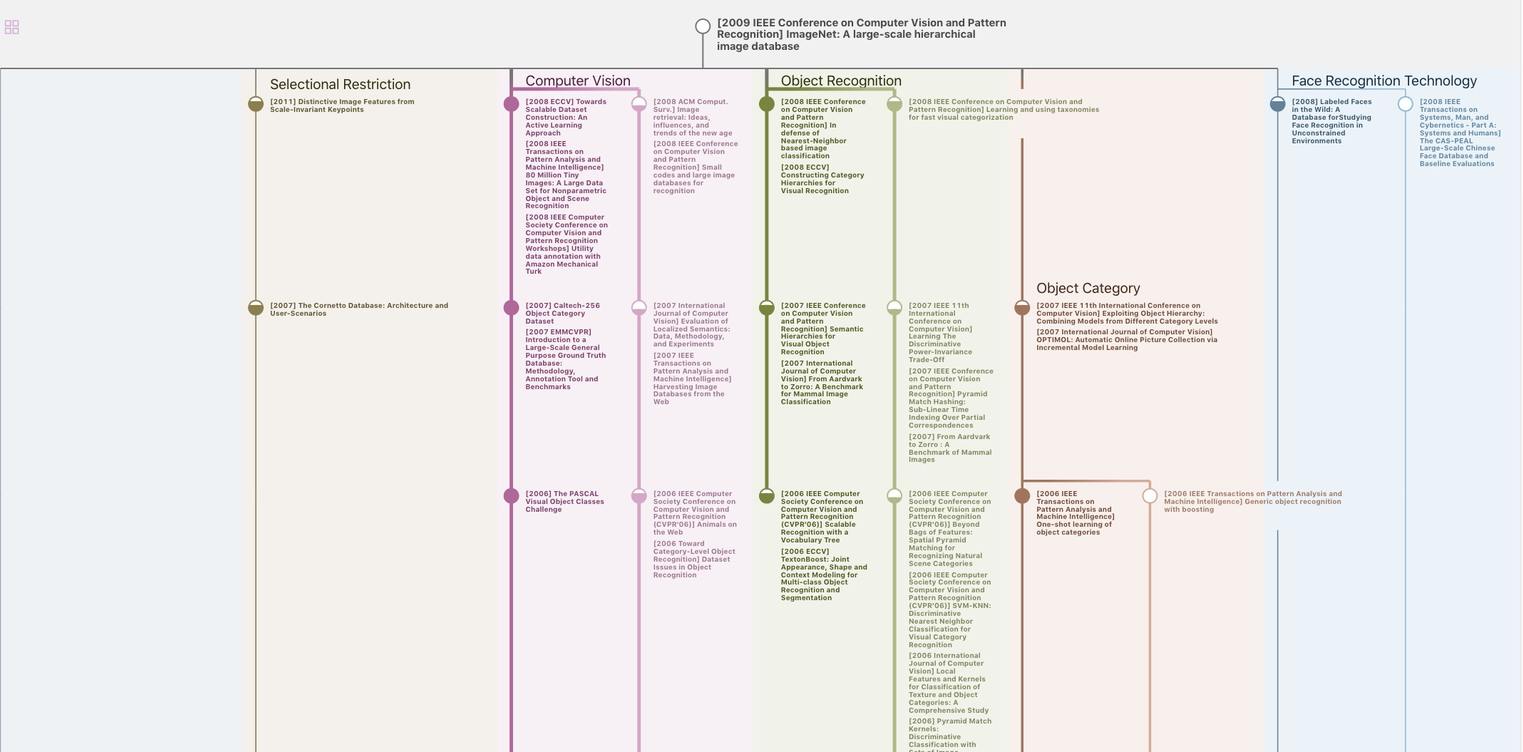
生成溯源树,研究论文发展脉络
Chat Paper
正在生成论文摘要