Single Degree Of Freedom Model Predictive Control With Variable Horizon
2020 AMERICAN CONTROL CONFERENCE (ACC)(2020)
摘要
This paper proposes a sampling-based model predictive control scheme with a single degree of freedom in control. A variable horizon and stabilizing terminal conditions ensure recursive feasibility, asymptotic stability, and improvement of the closed-loop performance. The initial derivation leads to a computationally demanding mixed-integer nonlinear programming problem. To address this issue, the paper presents an algorithm that seeks the optimal horizon while rolling out a finite number of control input candidates. The proposed derivative-free optimization even finds the control invariant terminal set online at reasonable computational overhead. The approach is straightforward to implement and aims for fast, albeit small-scale systems. A comparative analysis with a fixed-horizon scheme and standard model predictive control on a nonlinear benchmark system shows high closed-loop performance with low computational effort.
更多查看译文
关键词
variable horizon,sampling-based model predictive control scheme,stabilizing terminal conditions,recursive feasibility,asymptotic stability,closed-loop performance,initial derivation,mixed-integer nonlinear programming problem,optimal horizon,control input candidates,derivative-free optimization,control invariant terminal,reasonable computational overhead,fixed-horizon scheme,standard model predictive control
AI 理解论文
溯源树
样例
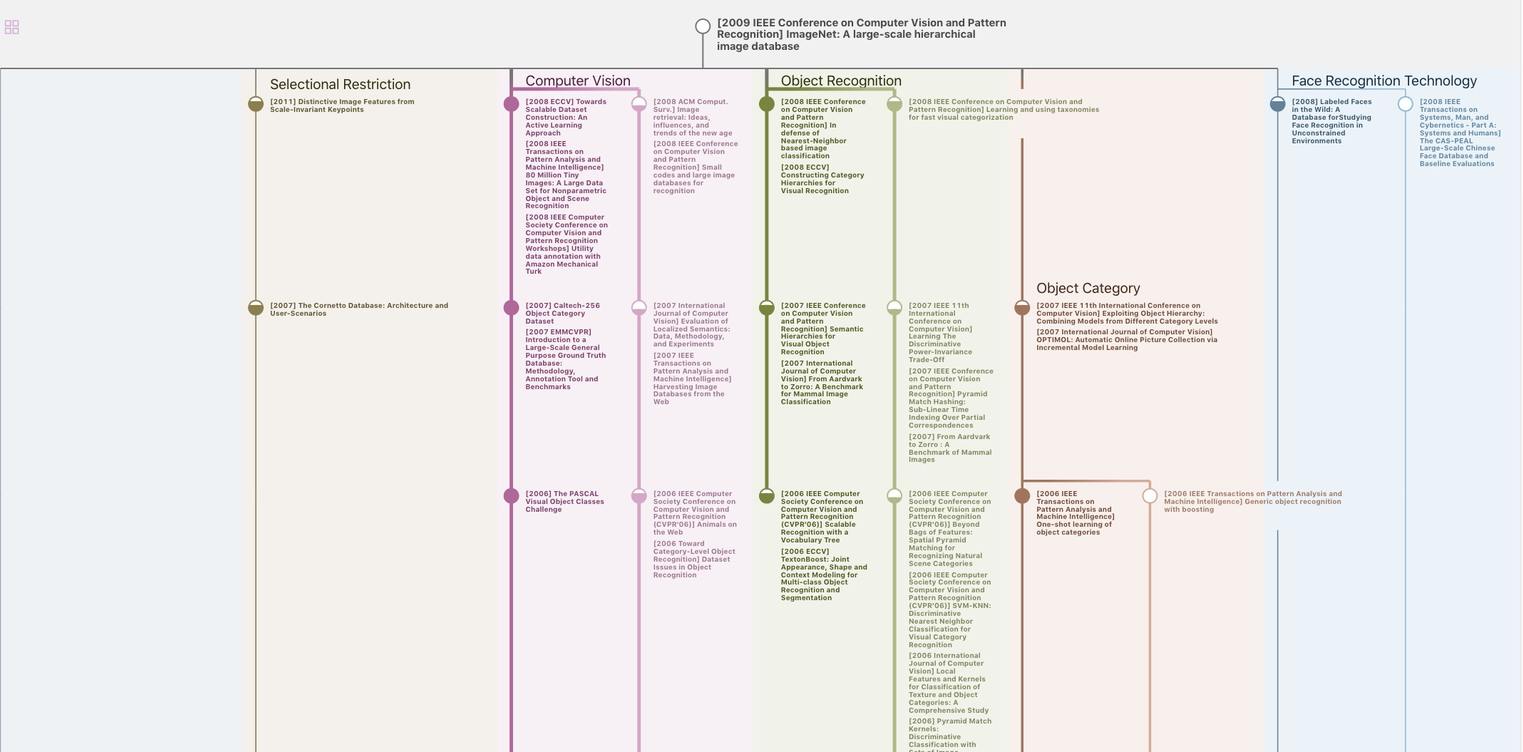
生成溯源树,研究论文发展脉络
Chat Paper
正在生成论文摘要