Variably Scaled Kernels Improve Classification of Hormonally-Treated Patient-Derived Xenografts
2020 IEEE Conference on Evolving and Adaptive Intelligent Systems (EAIS)(2020)
摘要
Little is known about the biological functions which are exerted by hormone receptors in physiological conditions. Here, we made use of the Mouse INtraDuctal (MIND) model, an innovative patient-derived xenograft (PDX) model, to characterize global gene expression changes, which are triggered by stimulation of dihydrotestosterone (DHT) and progesterone (P4) in vivo. Fast and clever mathematical tools are needed to analyze increasing numbers of complex datasets. We generated hormone receptor-specific list of genes which were then used to test the classification performance obtained by different machine learning algorithms in the frame of our labelled PDXs RNAseq dataset. Next to other standard techniques, we consider the variably scaled kernel (VSK) setting in the framework of support vector machines. Our results show that mixed schemes obtained via VSKs can outperform standard classification methods in the considered task.
更多查看译文
关键词
Variably scaled kernels,patient-derived xenografts,hormones
AI 理解论文
溯源树
样例
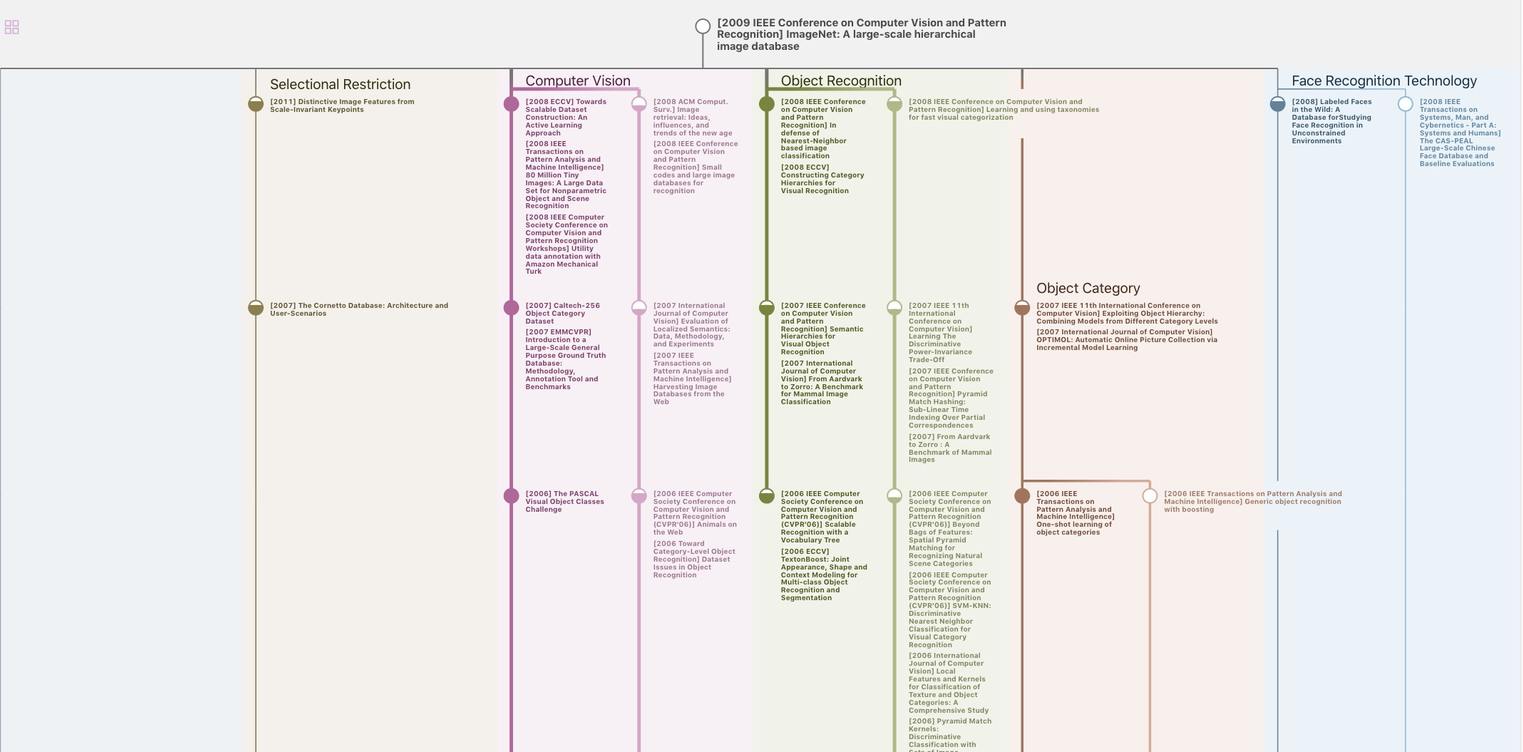
生成溯源树,研究论文发展脉络
Chat Paper
正在生成论文摘要