Fast And Accurate Ranking Regression
INTERNATIONAL CONFERENCE ON ARTIFICIAL INTELLIGENCE AND STATISTICS, VOL 108(2020)
Abstract
We consider a ranking regression problem in which we use a dataset of ranked choices to learn Plackett-Luce scores as functions of sample features. We solve the maximum likelihood estimation problem by using the Alternating Directions Method of Multipliers (ADMM), effectively separating the learning of scores and model parameters. This separation allows us to express scores as the stationary distribution of a continuous-time Markov Chain. Using this equivalence, we propose two spectral algorithms for ranking regression that learn model parameters up to 579 times faster than the Newton's method.
MoreTranslated text
Key words
accurate ranking regression
AI Read Science
Must-Reading Tree
Example
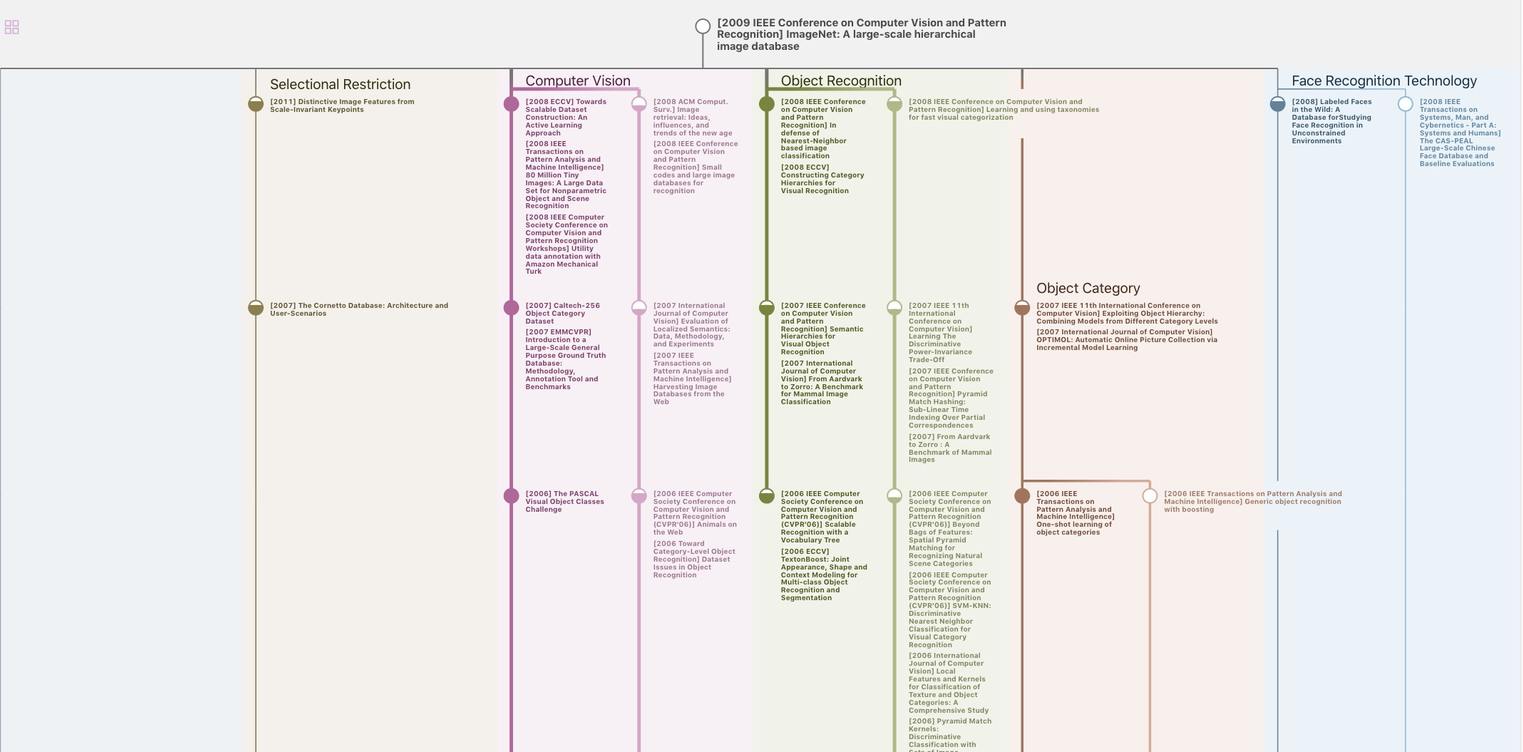
Generate MRT to find the research sequence of this paper
Chat Paper
Summary is being generated by the instructions you defined