Low Precision Floating-point Arithmetic for High Performance FPGA-based CNN Acceleration
FPGA(2020)
摘要
Low precision data representation is important to reduce storage size and memory access for convolutional neural networks (CNNs). Yet, existing methods have two major limitations: (1) requiring re-training to maintain accuracy for deep CNNs, and (2) needing 16-bit floating-point or 8-bit fixed-point for a good accuracy. In this paper, we propose a low precision (8-bit) floating-point (LPFP) quantization method for FPGA-based acceleration to overcome the above limitations. Without any re-training, LPFP finds an optimal 8-bit data representation with negligible top-1/top-5 accuracy loss (within 0.5%/0.3% in our experiments, respectively, and significantly better than existing methods for deep CNNs). Furthermore, we implement one 8-bit LPFP multiplication by one 4-bit multiply-adder (MAC) and one 3-bit adder, and therefore implement four 8-bit LPFP multiplications using one DSP slice of Xilinx Kintex 7 family (KC705 in this paper) while one DSP can implement only two 8-bit fixed-point multiplications. Experiments on six typical CNNs for inference show that on average, we improve throughput by 64.5x over Intel i9 CPU and by 1.5x over existing FPGA accelerators. Particularly for VGG16 and YOLO, compared to six recent FPGA accelerators, we improve average throughput by 3.5x and 27.5x and improve average throughput per DSP by 4.1x and 5x, respectively. To the best of our knowledge, this is the first in-depth study to simplify one multiplication for CNN inference to one 4-bit MAC and implement four multiplications within one DSP while maintaining comparable accuracy without any re-training.
更多查看译文
关键词
Low-precision floating-point,CNN,deep learning,FPGA processor,FPGA acceleration
AI 理解论文
溯源树
样例
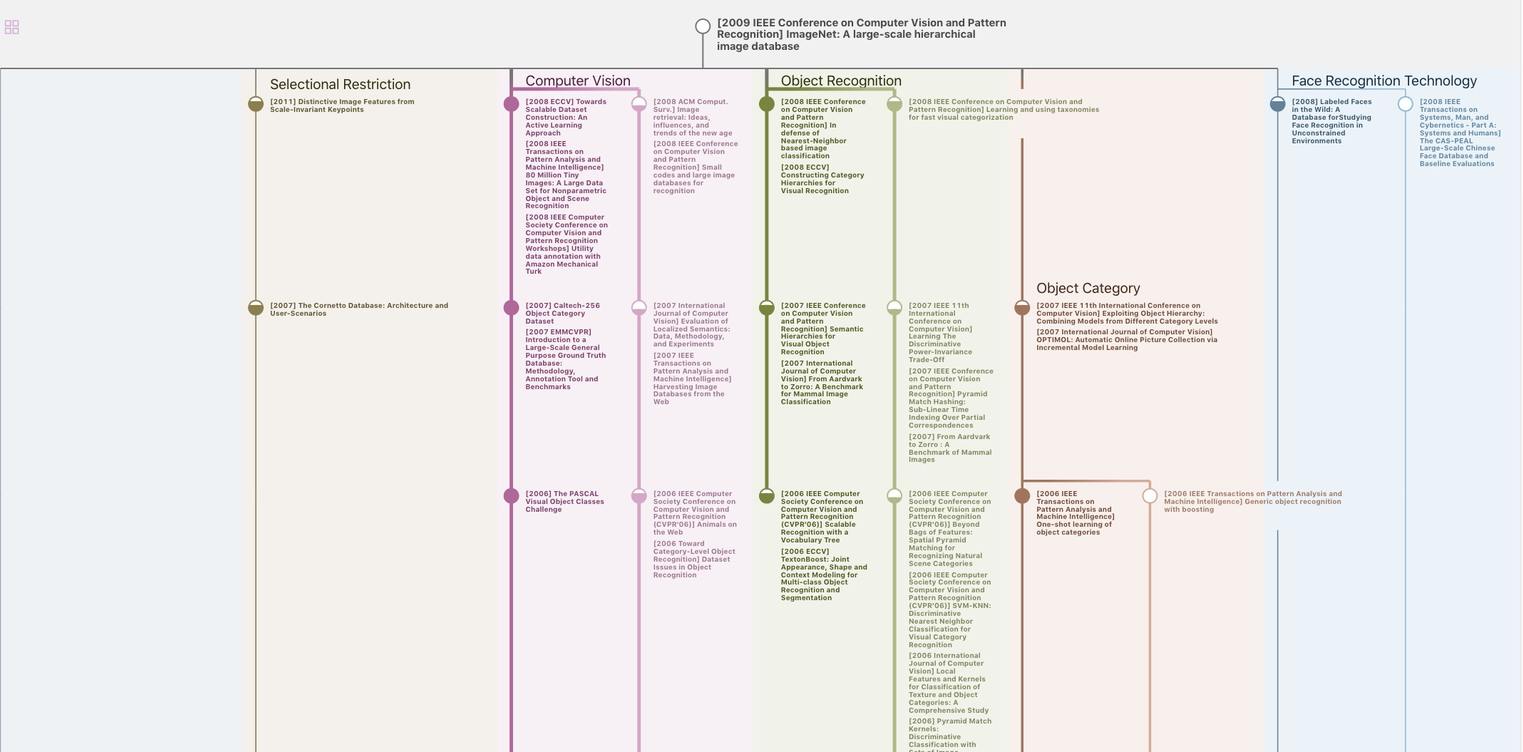
生成溯源树,研究论文发展脉络
Chat Paper
正在生成论文摘要