Multi-Label Learning Vector Quantization For Semi-Supervised Classification
INTELLIGENT DATA ANALYSIS(2019)
摘要
In the context of expensive and time-consuming acquisition of reliably labeled data, how to utilize the unlabeled instances that can potentially improve the classification accuracy becomes an attractive problem with significant importance in practice. Semi-supervised classification that fills the gap between supervised learning and unsupervised learning is designed to take advantage of the unlabeled data in regular supervised learning procedure for classification tasks. In this paper we proposed a self-learning framework, that firstly pre-learns a classification model using the labeled data, then makes the prediction of unlabeled instances in the form of soft class labels, and re-learned a model based on the enlarged training data. Two multi-label Learning Vector Quantization Neural Networks (LVQ-NNs) are proposed, namely multi-label online LVQ-NN (mLVQo) and multi-label batch LVQ-NN (mLVQb), to work with the soft labels of training instances. The experiments demonstrate that the semi-supervised models using multi-label LVQ-NN as the base classifier can produce better generalization accuracy than the supervised counterpart.
更多查看译文
关键词
Semi-supervised classification, self-training, soft label, entropy, multi-label learning vector quantization
AI 理解论文
溯源树
样例
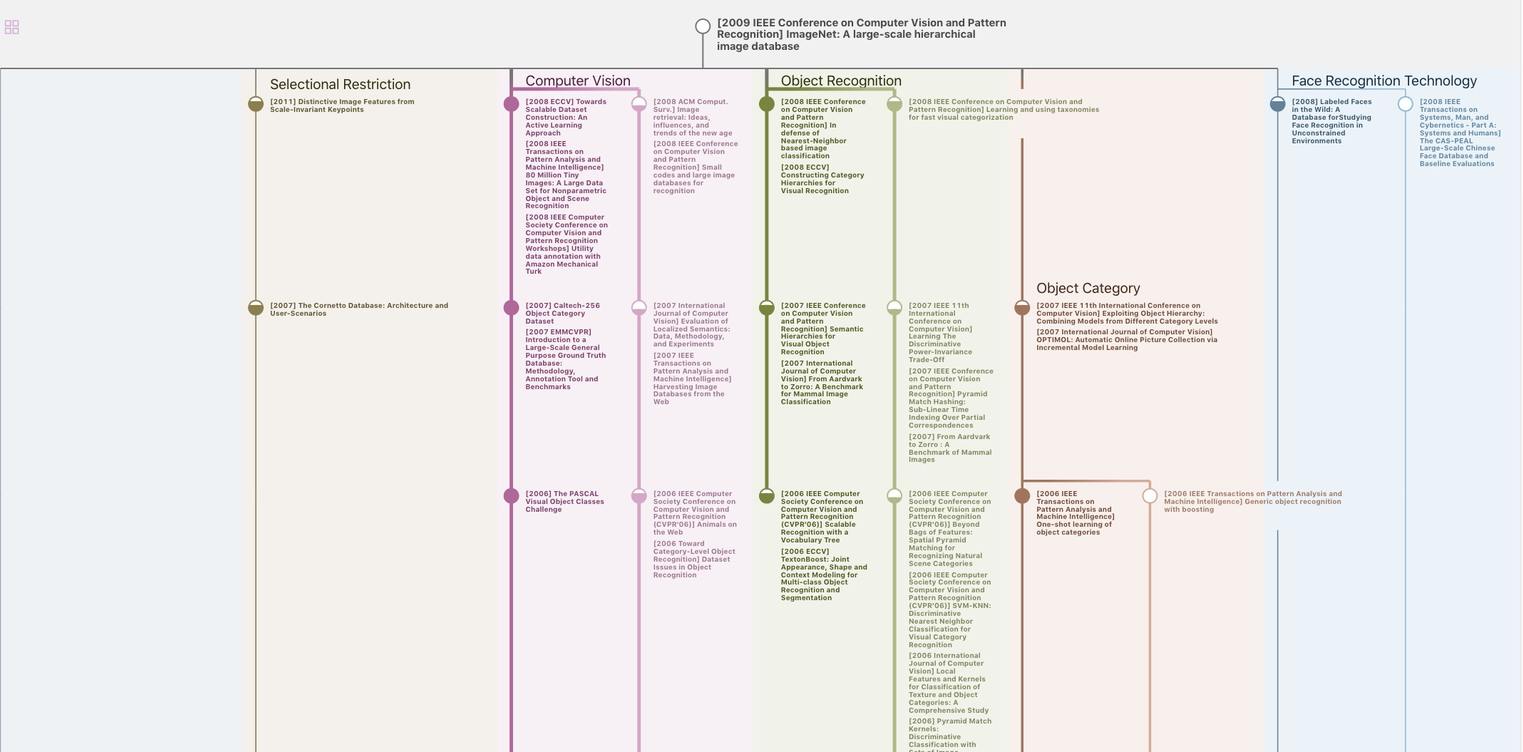
生成溯源树,研究论文发展脉络
Chat Paper
正在生成论文摘要