Hierarchical Federated Learning Across Heterogeneous Cellular Networks
2020 IEEE INTERNATIONAL CONFERENCE ON ACOUSTICS, SPEECH, AND SIGNAL PROCESSING(2020)
摘要
We consider federated edge learning (FEEL), where mobile users (MUs) collaboratively learn a global model by sharing local updates on the model parameters rather than their datasets, with the help of a mobile base station (MBS). We optimize the resource allocation among MUs to reduce the communication latency in learning iterations. Observing that the performance in this centralized setting is limited due to the distance of the cell-edge users to the MBS, we introduce small cell base stations (SBSs) orchestrating FEEL among MUs within their cells, and periodically exchanging model updates with the MBS for global consensus. We show that this hierarchical federated learning (HFL) scheme significantly reduces the communication latency without sacrificing the accuracy.
更多查看译文
关键词
Cellular networks, federated learning, mobile edge processing, resource allocation
AI 理解论文
溯源树
样例
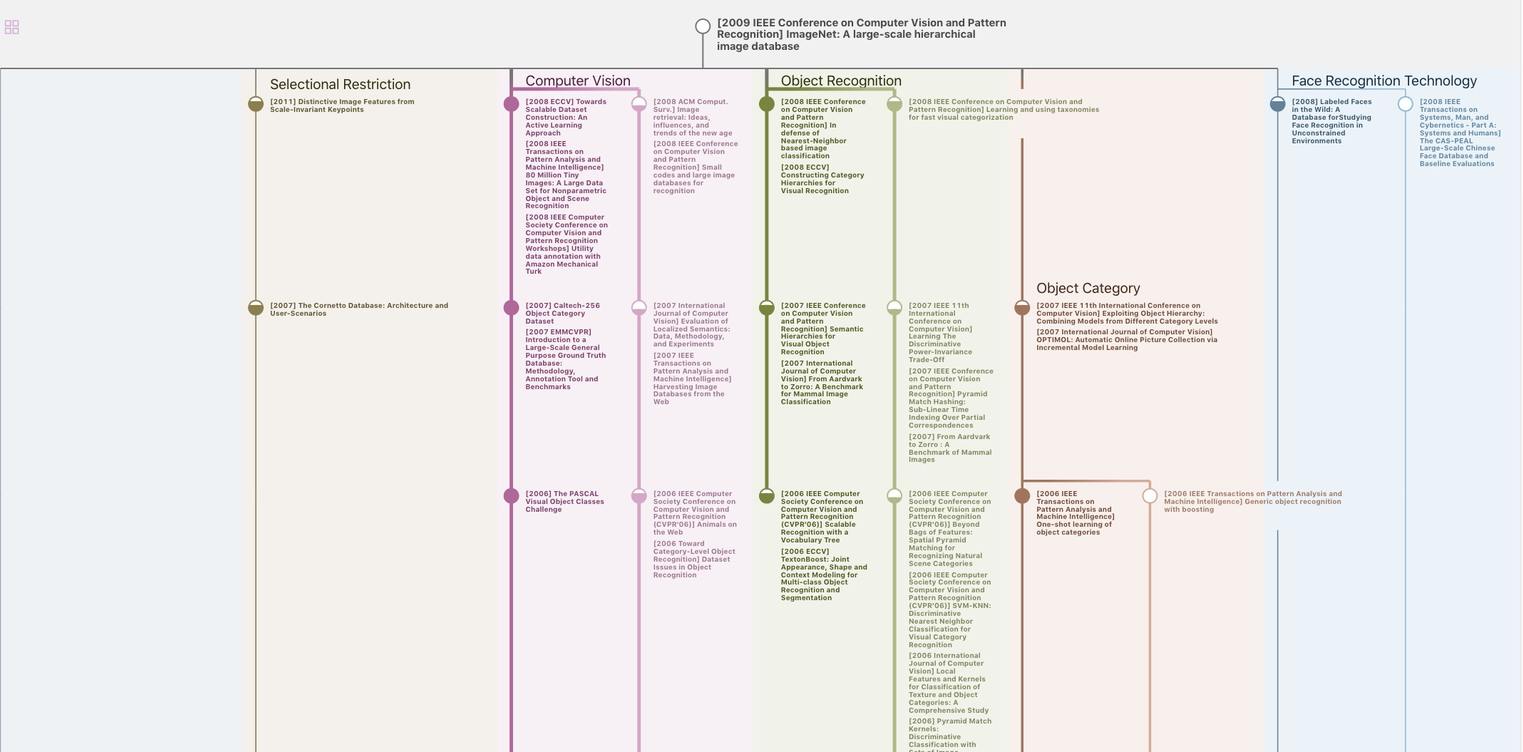
生成溯源树,研究论文发展脉络
Chat Paper
正在生成论文摘要