Optimal Trade-offs in Multi-Processor Approximate Message Passing
arXiv: Information Theory(2016)
Abstract
We consider large-scale linear inverse problems in Bayesian settings. We follow a recent line of work that applies the approximate message passing (AMP) framework to multi-processor (MP) computational systems, where each processor node stores and processes a subset of rows of the measurement matrix along with corresponding measurements. In each MP-AMP iteration, nodes of the MP system and its fusion center exchange lossily compressed messages pertaining to their estimates of the input. In this setup, we derive the optimal per-iteration coding rates using dynamic programming. We analyze the excess mean squared error (EMSE) beyond the minimum mean squared error (MMSE), and prove that, in the limit of low EMSE, the optimal coding rates increase approximately linearly per iteration. Additionally, we obtain that the combined cost of computation and communication scales with the desired estimation quality according to $O(log^2(1/text{EMSE}))$. Finally, we study trade-offs between the physical costs of the estimation process including computation time, communication loads, and the estimation quality as a multi-objective optimization problem, and characterize the properties of the Pareto optimal surfaces.
MoreTranslated text
AI Read Science
Must-Reading Tree
Example
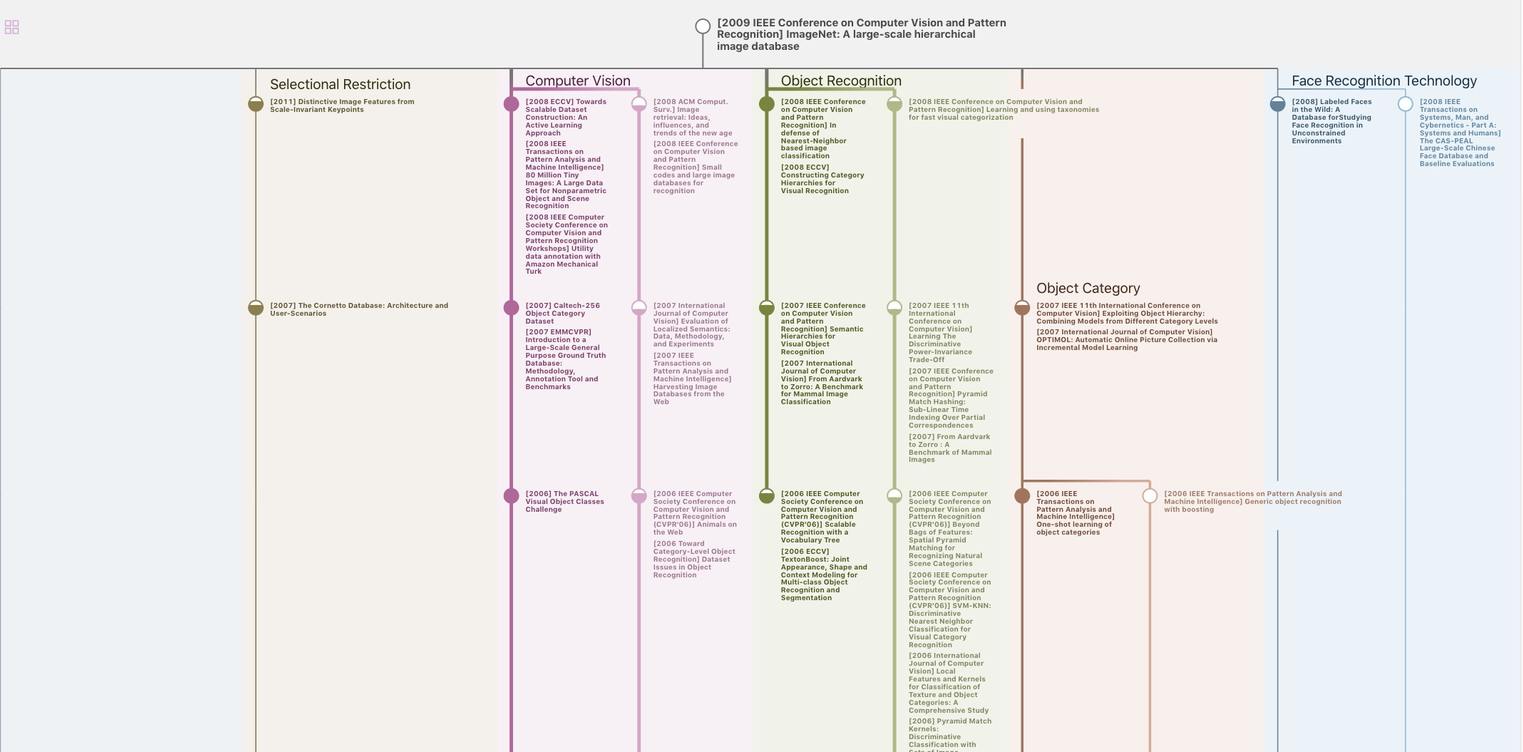
Generate MRT to find the research sequence of this paper
Chat Paper
Summary is being generated by the instructions you defined