Lightweight Refresh Method for PCM-based Neuromorphic Circuits
2018 IEEE 18th International Conference on Nanotechnology (IEEE-NANO)(2018)
摘要
Phase change memory (PCM) is being explored as a synaptic nanodevice for scalable and low-power neuromorphic circuits. We present a novel and lightweight method to refresh PCM cells after they saturate at their maximum conductance during the learning process. Our learning system is an event-based Restricted Boltzmann Machine with Spike Time Dependent Plasticity update rule using a modified contrastive divergence algorithm. By using our event-based neuromorphic circuit simulator and the MNIST handwritten digit dataset, we show that our refresh method reduces power consumption by decreasing the number of SET and RESET programming pulses while maintaining high learning accuracy.
更多查看译文
关键词
Neurons,Phase change materials,Synapses,Neuromorphics,Integrated circuit modeling,Timing,Power demand
AI 理解论文
溯源树
样例
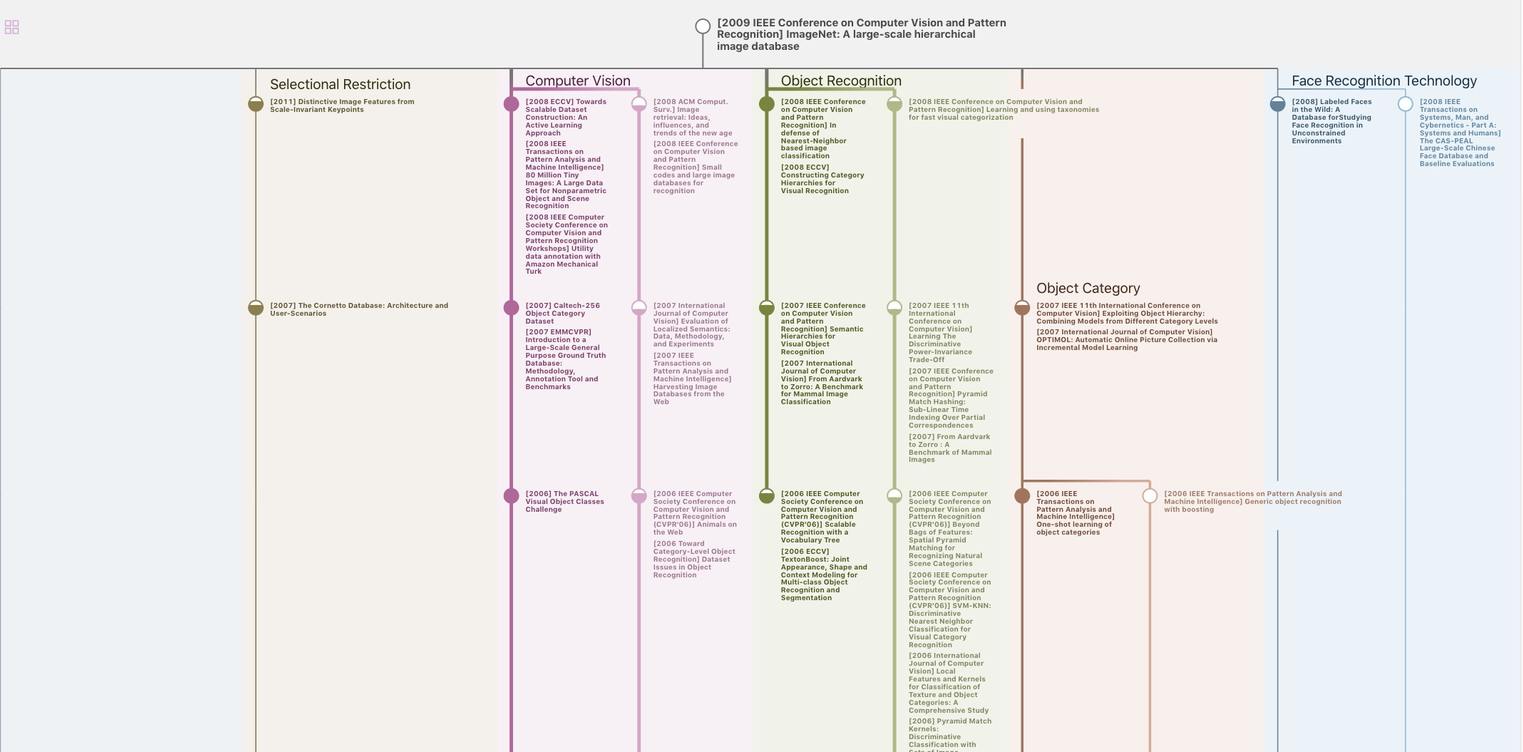
生成溯源树,研究论文发展脉络
Chat Paper
正在生成论文摘要