Machine Learning Approaches to Predict Functional Upper Extremity Use in Individuals with Stroke
2018 IEEE INTERNATIONAL CONFERENCE ON BIG DATA (BIG DATA)(2018)
摘要
The majority of stroke survivors suffer from residual functional deficits in the Upper Extremity (UE) that limits the patients' ability to incorporate the hemiparetic UE into daily function such as reaching and grasping objects. Correctly assessing the degree to which individuals with stroke use their UE would be important for rehabilitation. However, previous work shows that many stroke patients, when observed in the laboratory, can appear to use the paretic arm with adequate ability, yet do not use the arm at home with the expected regularity. The main purposes of this study are to use various machine learning techniques to examine associations between laboratory-based measures of limb functioning and actual home use. The UE kinematics during reaching and grasping tasks were measured in the laboratory, while the use of the paretic arm in the home environment was assessed with portable accelerometry and the Motor Activity Log (MAL). The study identified a subset of the biomechanical features that predicted well the paretic arm use at home. Arm use was also well predicted with clinical evaluations of impairment using Fugl-Meyer and Action Research Arm Test (ARAT) scores. However, combining the clinical and a subset of biomechanical features yielded the best model to predict UE use in individuals with stroke.
更多查看译文
关键词
machine learning, upper extremity, stroke, kinematics, accelerometry
AI 理解论文
溯源树
样例
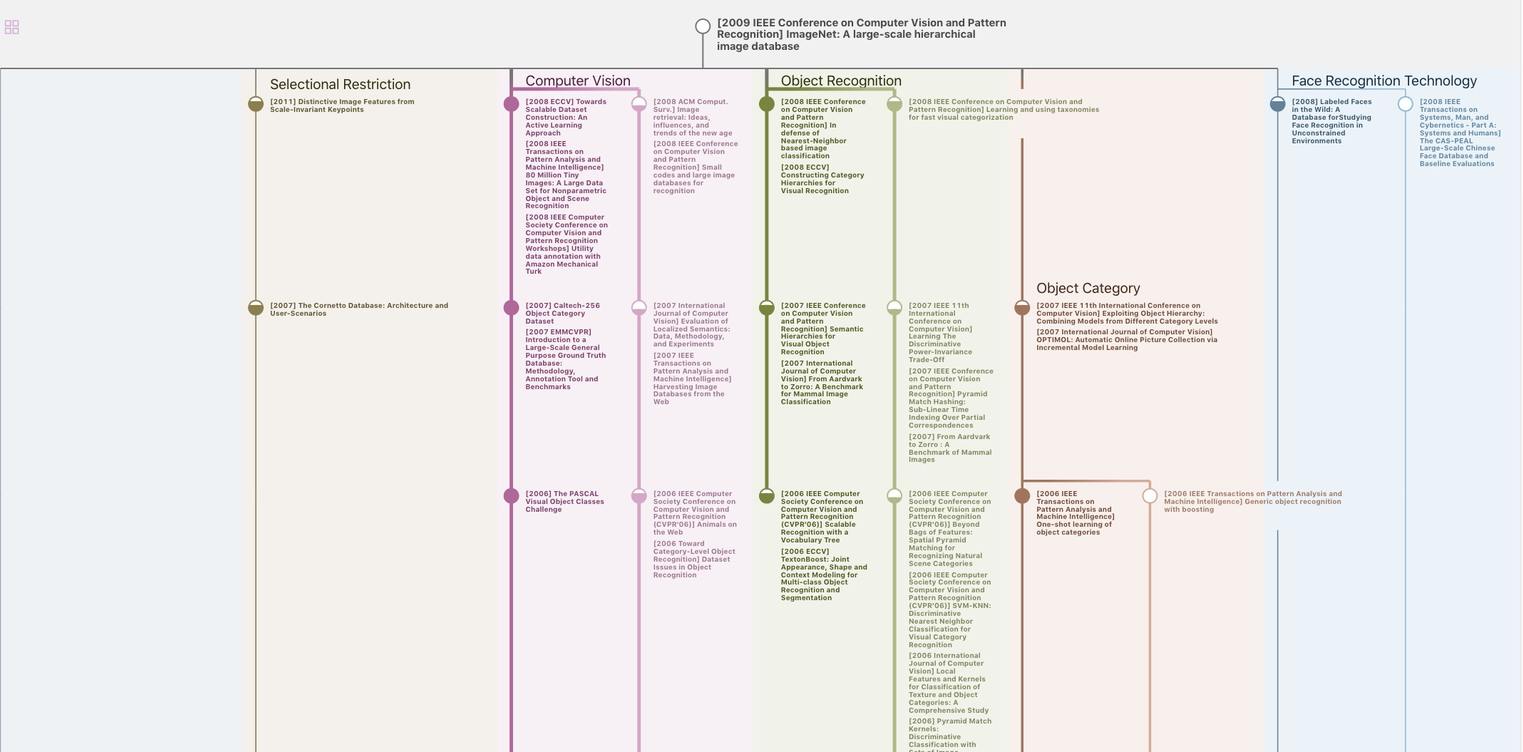
生成溯源树,研究论文发展脉络
Chat Paper
正在生成论文摘要