Optimization Of Selective Ensemble For Cost-Sensitive Classification: An Empirical Study
INTELLIGENT DECISION TECHNOLOGIES-NETHERLANDS(2018)
摘要
Cost-sensitive classification is broadly investigated in many real-life decision-making applications where the different misclassification errors may cause asymmetric costs. However, the cost values are usually hardly specified in practice for decision makers especially when they are faced with the complex multi-class decision problems. In this paper we attempt to take advantage of the pairwise comparisons originally proposed in Analytic Hierarchy Process (AHP) and offer decision makers a flexible, qualitative manner for cost specification rather than the definite, quantitative cost assignment. The cost ratios associated with the classes are then derived from the comparison matrix and used as the parameter of the subsequent cost-sensitive classifiers. To promote the performance of single classifier we construct the ensembles of multiple cost-sensitive Learning Vector Quantization Neural Networks (LVQ-NNs) trained independently and then combined together by various weighted voting approaches. Empirical study on some real-world databases reveal that the well optimized cost-sensitive ensembles based on evolutionary computing approaches perform significantly better than the best single classifier within the ensemble in terms of generalization ability and stability.
更多查看译文
关键词
Cost-sensitive classification, selective ensemble learning, evolutionary computing, pairwise comparison matrix, learning vector quantization neural network
AI 理解论文
溯源树
样例
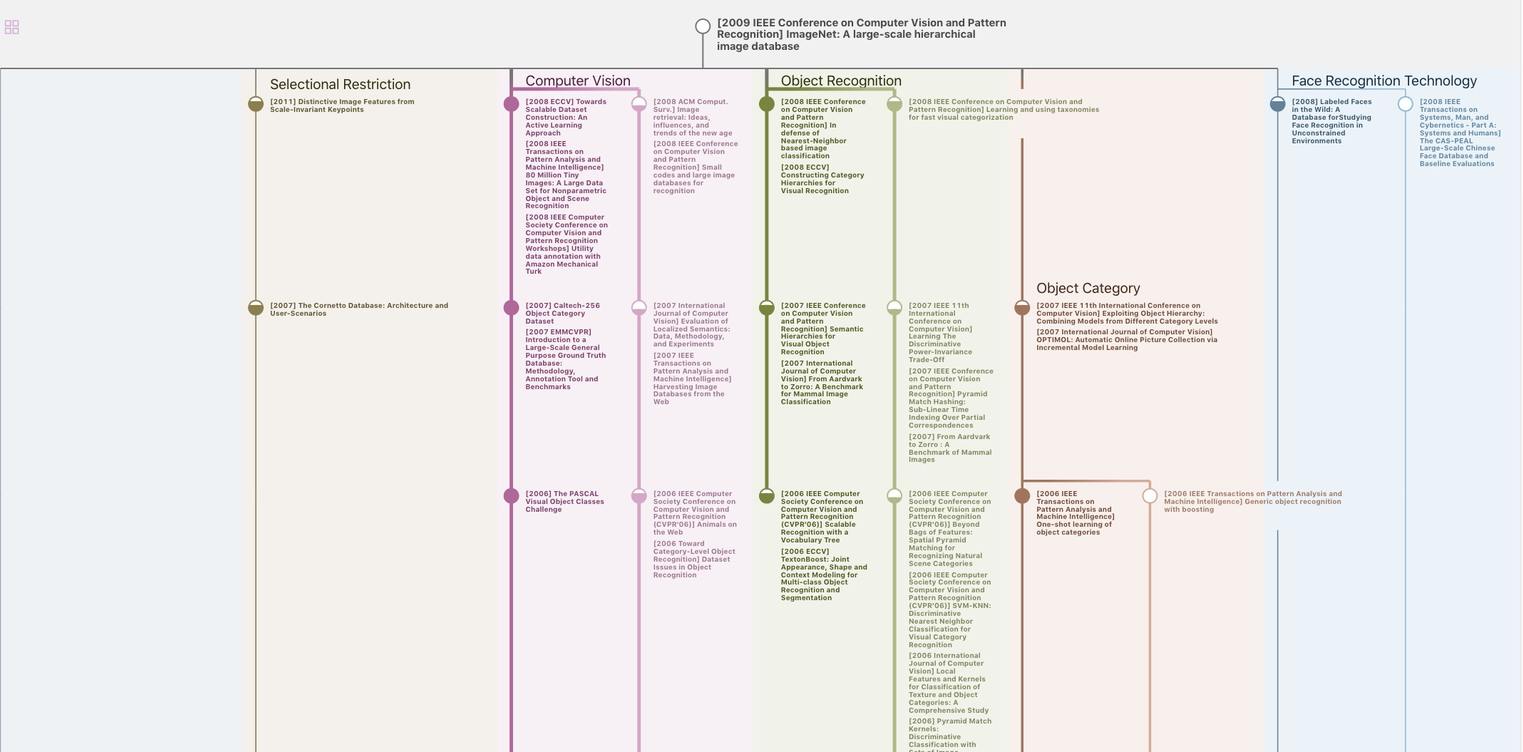
生成溯源树,研究论文发展脉络
Chat Paper
正在生成论文摘要