Prediction and identification of the effectors of heterotrimeric G proteins in rice (Oryza sativa L.).
Briefings in bioinformatics(2017)
Abstract
Heterotrimeric G protein signaling cascades are one of the primary metazoan sensing mechanisms linking a cell to environment. However, the number of experimentally identified effectors of G protein in plant is limited. We have therefore studied which tools are best suited for predicting G protein effectors in rice. Here, we compared the predicting performance of four classifiers with eight different encoding schemes on the effectors of G proteins by using 10-fold cross-validation. Four methods were evaluated: random forest, naive Bayes, K-nearest neighbors and support vector machine. We applied these methods to experimentally identified effectors of G proteins and randomly selected non-effector proteins, and tested their sensitivity and specificity. The result showed that random forest classifier with composition of K-spaced amino acid pairs and composition of motif or domain (CKSAAP_PROSITE_200) combination method yielded the best performance, with accuracy and the Mathew's correlation coefficient reaching 74.62% and 0.49, respectively. We have developed G-Effector, an online predictor, which outperforms BLAST, PSI-BLAST and HMMER on predicting the effectors of G proteins. This provided valuable guidance for the researchers to select classifiers combined with different feature selection encoding schemes. We used G-Effector to screen the effectors of G protein in rice, and confirmed the candidate effectors by gene co-expression data. Interestingly, one of the top 15 candidates, which did not appear in the training data set, was validated in a previous research work. Therefore, the candidate effectors list in this article provides both a clue for researchers as to their function and a framework of validation for future experimental work. It is accessible at http://bioinformatics.fafu.edu.cn/geffector.
MoreTranslated text
AI Read Science
Must-Reading Tree
Example
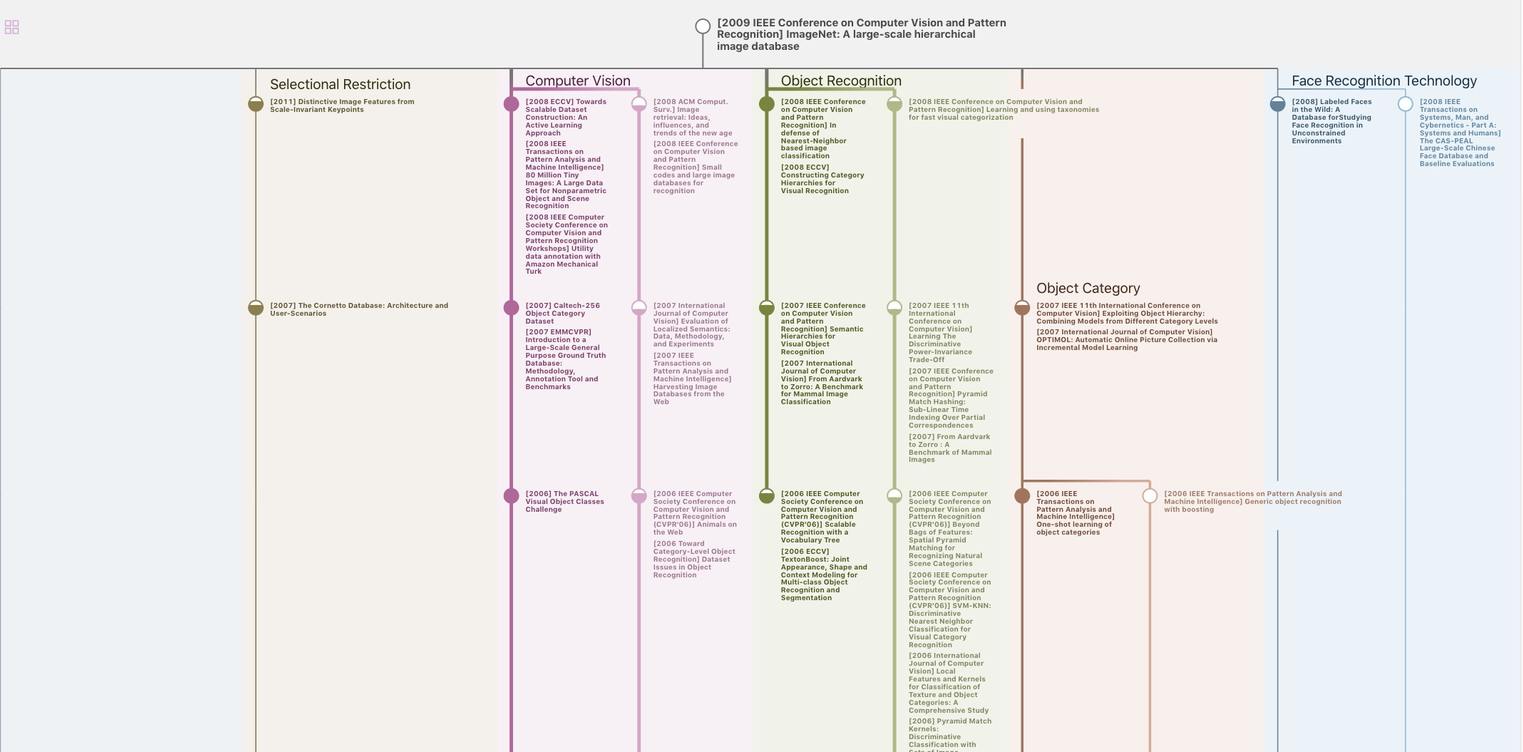
Generate MRT to find the research sequence of this paper
Chat Paper
Summary is being generated by the instructions you defined