Invasive Cancer Detection Utilizing Compressed Convolutional Neural Network And Transfer Learning
MEDICAL IMAGE COMPUTING AND COMPUTER ASSISTED INTERVENTION - MICCAI 2018, PT II(2018)
摘要
Identification of invasive cancer in Whole Slide Images (WSIs) is crucial for tumor staging as well as treatment planning. However, the precise manual delineation of tumor regions is challenging, tedious and time-consuming. Thus, automatic invasive cancer detection in WSIs is of significant importance. Recently, Convolutional Neural Network (CNN) based approaches advanced invasive cancer detection. However, computation burdens of these approaches become barriers in clinical applications. In this work, we propose to detect invasive cancer employing a lightweight network in a fully convolution fashion without model ensembles. In order to improve the small network's detection accuracy, we utilized the "soft labels" of a large capacity network to supervise its training process. Additionally, we adopt a teacher guided loss to help the small network better learn from the intermediate layers of the high capacity network. With this suite of approaches, our network is extremely efficient as well as accurate. The proposed method is validated on two large scale WSI datasets. Our approach is performed in an average time of 0.6 and 3.6min per WSI with a single GPU on our gastric cancer dataset and CAMELYON16, respectively, about 5 times faster than Google Inception V3. We achieved an average FROC of 81.1% and 85.6% respectively, which are on par with Google Inception V3. The proposed method requires less high performance computing resources than state-of-the-art methods, which makes the invasive cancer diagnosis more applicable in the clinical usage.
更多查看译文
关键词
Invasive Cancer Detection, Transfer Learning, Large Network Capacity, Whole Slide Images (WSI), Gastric Cancer Dataset
AI 理解论文
溯源树
样例
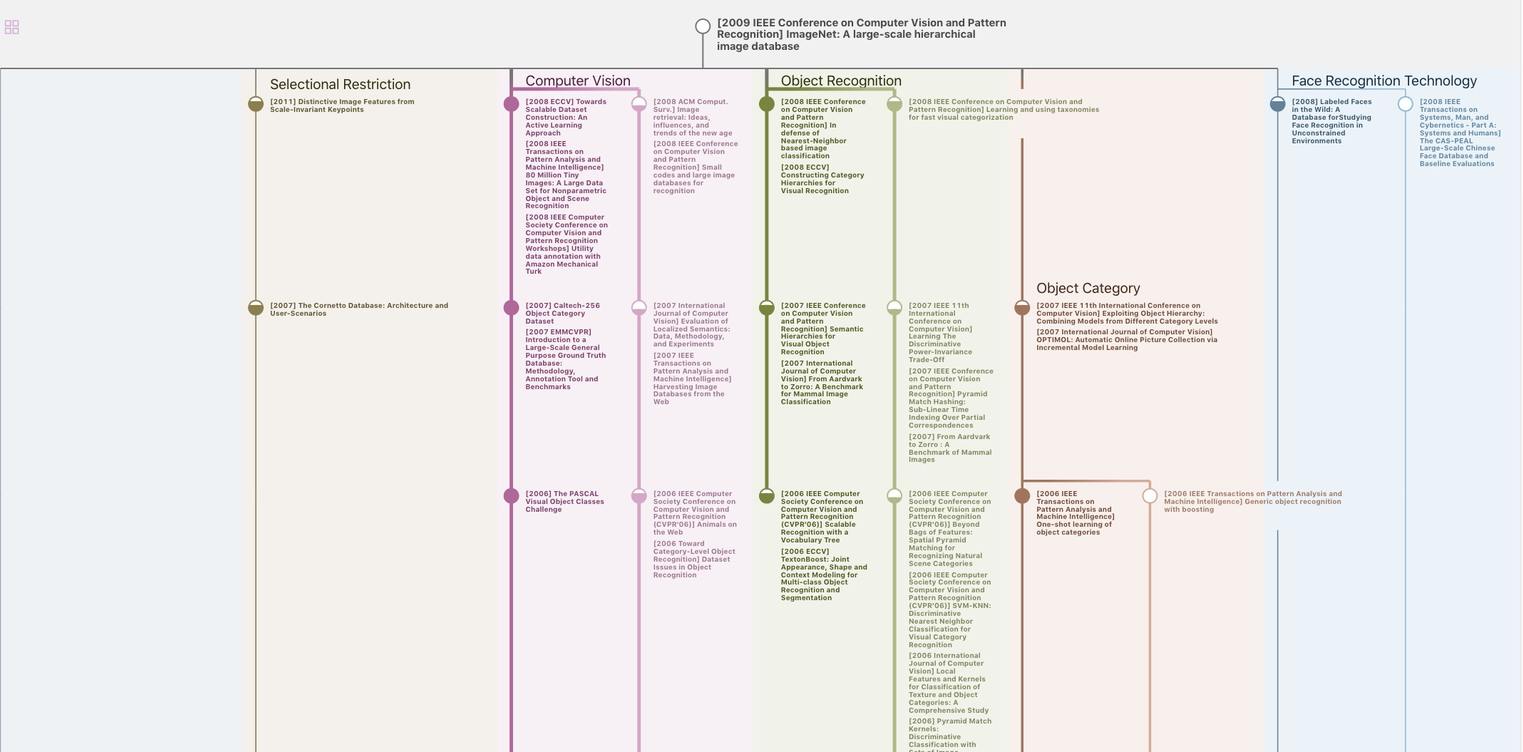
生成溯源树,研究论文发展脉络
Chat Paper
正在生成论文摘要