Mean-square error constrained approach to robust stochastic iterative learning control
IET Control Theory & Applications(2018)
摘要
A Kalman filtering-based robust iterative learning control algorithm is proposed in this study for linear stochastic systems with uncertain dynamics and unknown noise statistics. Firstly, a learning gain matrix is designed for the nominal case by minimising the trace of the mean-square matrix of the input tracking error. Theoretical results show that the proposed algorithm guarantees not only the asymptotic but also monotonic convergence of the input tracking error in the mean-square error sense, especially when random noises are Gaussian distributed the proposed algorithm is further proved to be asymptotically efficient. In addition, a new mean-square error constrained approach is presented in designing the robust learning gain matrix, taking into account model uncertainties. A sufficient condition is provided such that the mean-square matrix of the input tracking error is constrained within a predesigned upper bound which can monotonically converge to zero. Finally, numerical examples considering both structured and unstructured model uncertainties are included to illustrate the effectiveness of the proposed algorithms.
更多查看译文
关键词
Gaussian distribution,iterative learning control,Kalman filters,matrix algebra,mean square error methods,robust control
AI 理解论文
溯源树
样例
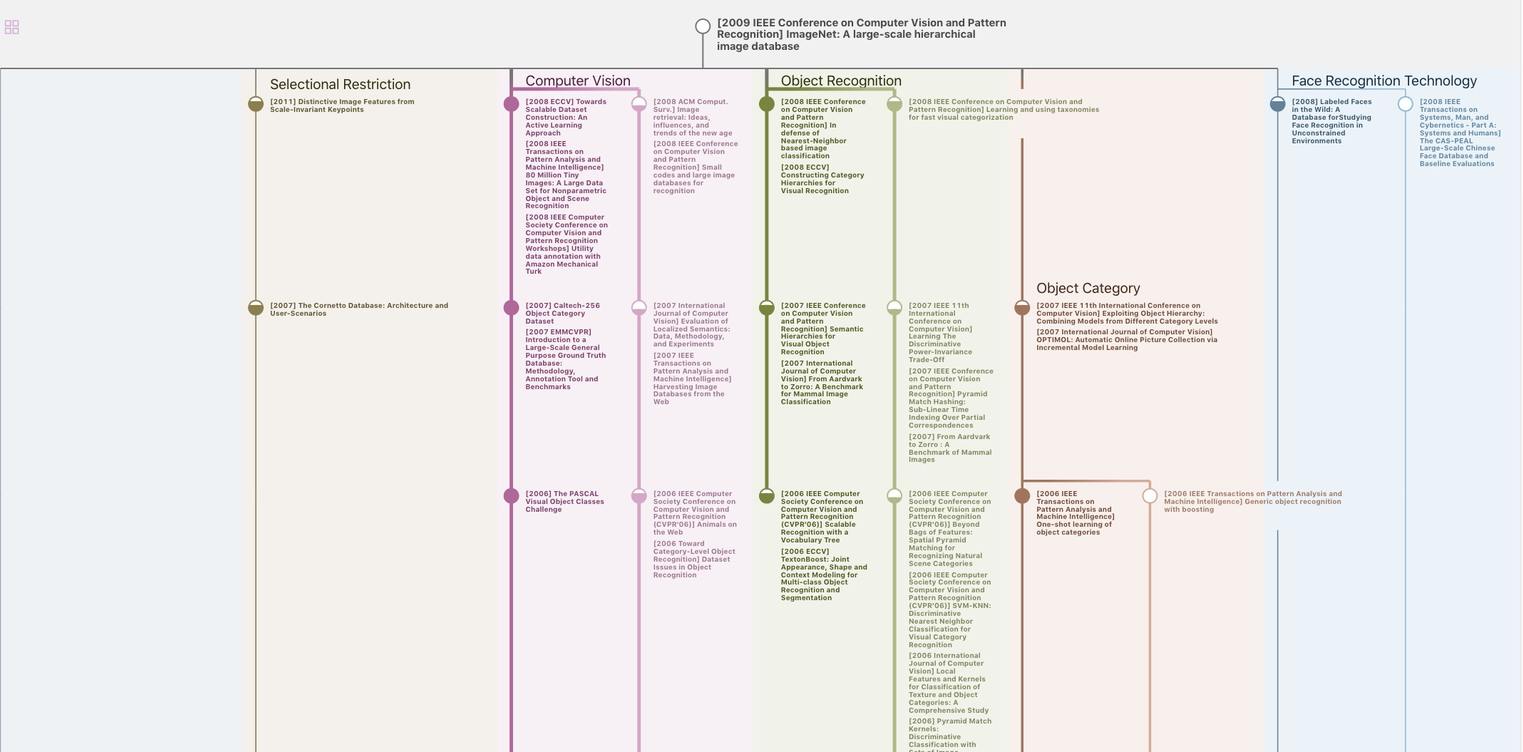
生成溯源树,研究论文发展脉络
Chat Paper
正在生成论文摘要