Generalized Geometric Programming for Rate Allocation in Consensus.
2017 55TH ANNUAL ALLERTON CONFERENCE ON COMMUNICATION, CONTROL, AND COMPUTING (ALLERTON)(2017)
摘要
Distributed averaging, or distributed average consensus, is a common method for computing the sample mean of the data dispersed among the nodes of a network in a decentralized manner. By iteratively exchanging messages with neighbors, the nodes of the network can converge to an agreement on the sample mean of their initial states. In real-world scenarios, these messages are subject to bandwidth and power constraints, which motivates the design of a lossy compression strategy. Few prior works consider the rate allocation problem from the perspective of constrained optimization, which provides a principled method for the design of lossy compression schemes, allows for the relaxation of certain assumptions, and offers performance guarantees. We show for Gaussian-distributed initial states with entropy-coded scalar quantization and vector quantization that the coding rates for distributed averaging can be optimized through generalized geometric programming. In the absence of side information from past states, this approach finds a rate allocation over nodes and iterations that minimizes the aggregate coding rate required to achieve a target mean square error within a finite run time. Our rate allocation is compared to some of the prior art through numerical simulations. The results motivate the incorporation of side-information through differential or predictive coding to improve rate-distortion performance.
更多查看译文
关键词
Compression,consensus,geometric programming,optimization,source coding
AI 理解论文
溯源树
样例
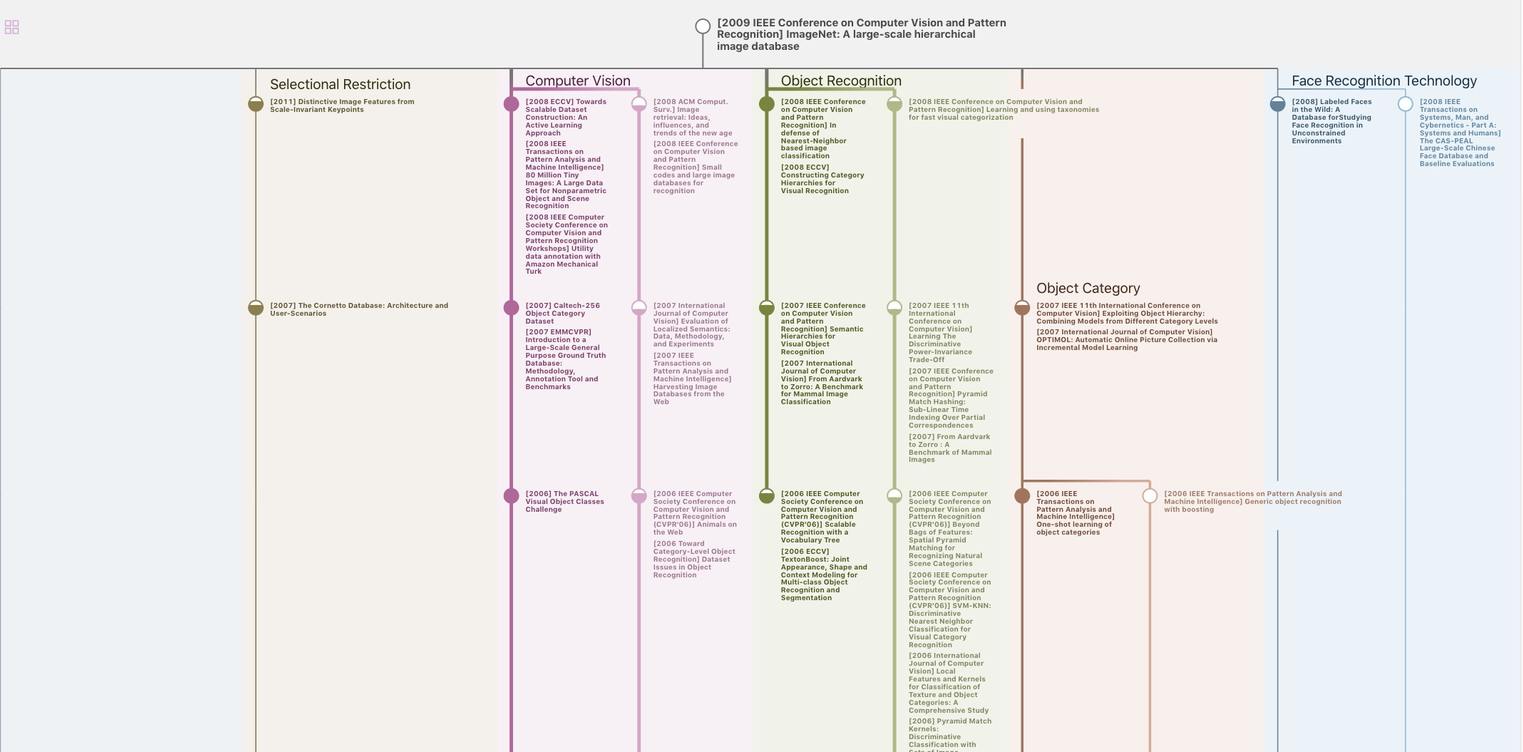
生成溯源树,研究论文发展脉络
Chat Paper
正在生成论文摘要