An overview of multi-processor approximate message passing
2017 51ST ANNUAL CONFERENCE ON INFORMATION SCIENCES AND SYSTEMS (CISS)(2017)
摘要
Approximate message passing (AMP) is an algorithmic framework for solving linear inverse problems from noisy measurements, with exciting applications such as reconstructing images, audio, hyper spectral images, and various other signals, including those acquired in compressive signal acquisition systems. The growing prevalence of big data systems has increased interest in large-scale problems, which may involve huge measurement matrices that are unsuitable for conventional computing systems. To address the challenge of large-scale processing, multi-processor (MP) versions of AMP have been developed. We provide an overview of two such MP-AMP variants. In row-MP-AMP, each computing node stores a subset of the rows of the matrix and processes corresponding measurements. In column-MP-AMP, each node stores a subset of columns, and is solely responsible for reconstructing a portion of the signal. We will discuss pros and cons of both approaches, summarize recent research results for each, and explain when each one may be a viable approach. Aspects that are highlighted include some recent results on state evolution for both MP-AMP algorithms, and the use of data compression to reduce communication in the MP network.
更多查看译文
关键词
Approximate message passing, compressed sensing, distributed linear systems, inverse problems, lossy compression, optimization
AI 理解论文
溯源树
样例
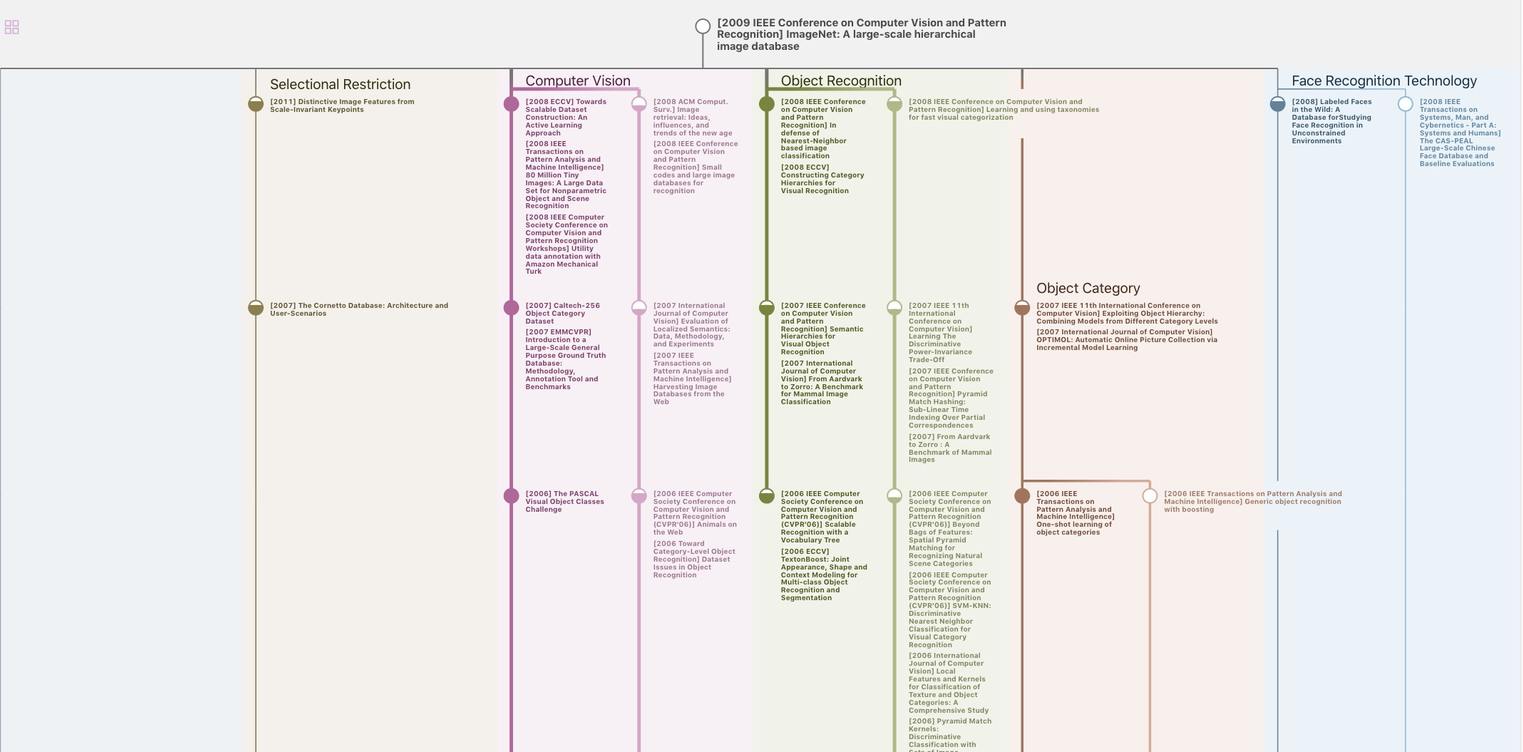
生成溯源树,研究论文发展脉络
Chat Paper
正在生成论文摘要