Automatic Graphic Logo Detection via Fast Region-based Convolutional Networks
2016 International Joint Conference on Neural Networks (IJCNN)(2016)
摘要
Brand recognition is a very challenging topic with many useful applications in localization recognition, advertisement and marketing. In this paper we present an automatic graphic logo detection system that robustly handles unconstrained imaging conditions. Our approach is based on Fast Region-based Convolutional Networks (FRCN) proposed by Ross Girshick, which have shown state-of-the-art performance in several generic object recognition tasks (PASCAL Visual Object Classes challenges). In particular, we use two CNN models pre-trained with the ILSVRC ImageNet dataset and we look at the selective search of windows `proposals' in the pre-processing stage and data augmentation to enhance the logo recognition rate. The novelty lies in the use of transfer learning to leverage powerful Convolutional Neural Network models trained with large-scale datasets and repurpose them in the context of graphic logo detection. Another benefit of this framework is that it allows for multiple detections of graphic logos using regions that are likely to have an object. Experimental results with the FlickrLogos-32 dataset show not only the promising performance of our developed models with respect to noise and other transformations a graphic logo can be subject to, but also its superiority over state-of-the-art systems with hand-crafted models and features.
更多查看译文
关键词
automatic graphic logo detection,fast region-based convolutional networks,brand recognition,unconstrained imaging conditions,FRCN,CNN models,ILSVRC ImageNet dataset,preprocessing stage,data augmentation,transfer learning,FlickrLogos-32 dataset
AI 理解论文
溯源树
样例
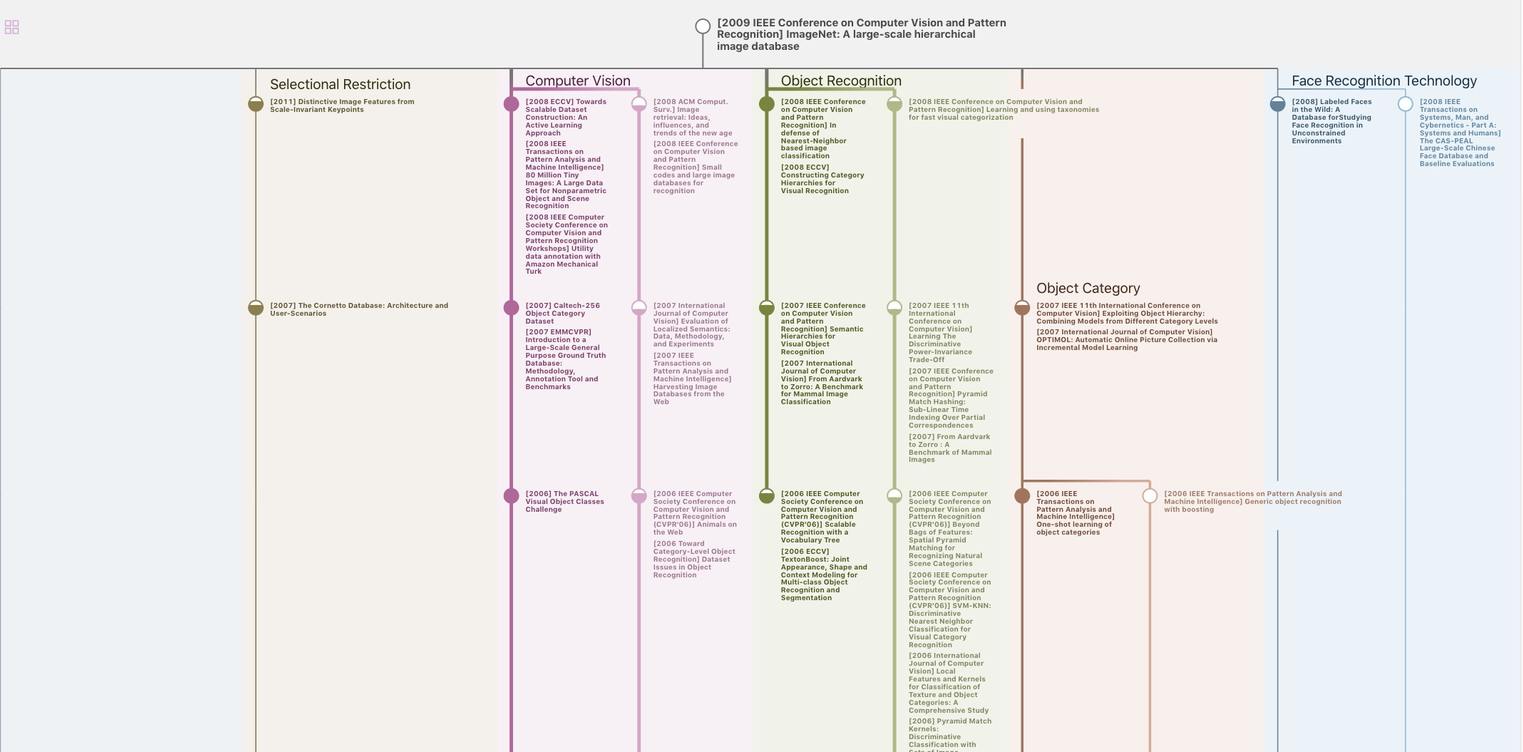
生成溯源树,研究论文发展脉络
Chat Paper
正在生成论文摘要