Recommending Interest Groups to Social Media Users by Incorporating Heterogeneous Resources.
IEA/AIE'13: Proceedings of the 26th International Conference on Industrial, Engineering and Other Applications of Applied Intelligent Systems(2013)
摘要
Due to the advance of social media technologies, it becomes easier for users to gather together to form groups online. Take the Last.fm for example (which is a popular music sharing website), users with common interests can join groups where they can share and discuss their loved songs. However, since the number of groups grows over time, users often need effective group recommendation (also called affiliation or community recommendation) in order to meet like-minded users. In this paper, based on the matrix factorization mechanism, we have investigated how to improve the accuracy of group recommendation by fusing other potentially useful information resources. Particulary, we adopt the collective factorization model to incorporate the user-item preference data, and the similarity-integrated regularization model to fuse the friendship data. The experiment on two real-world datasets (namely Last.fm and Douban) shows the outperforming impact of the chosen models relative to others on addressing the data sparsity problem and enhancing the algorithm's accuracy. Moreover, the experimental results identify that the user-item preference data can be more effective than the friendship in terms of benefiting the group recommendation.
更多查看译文
关键词
Recommending groups, matrix factorization, regularization, user-item preferences, friendship
AI 理解论文
溯源树
样例
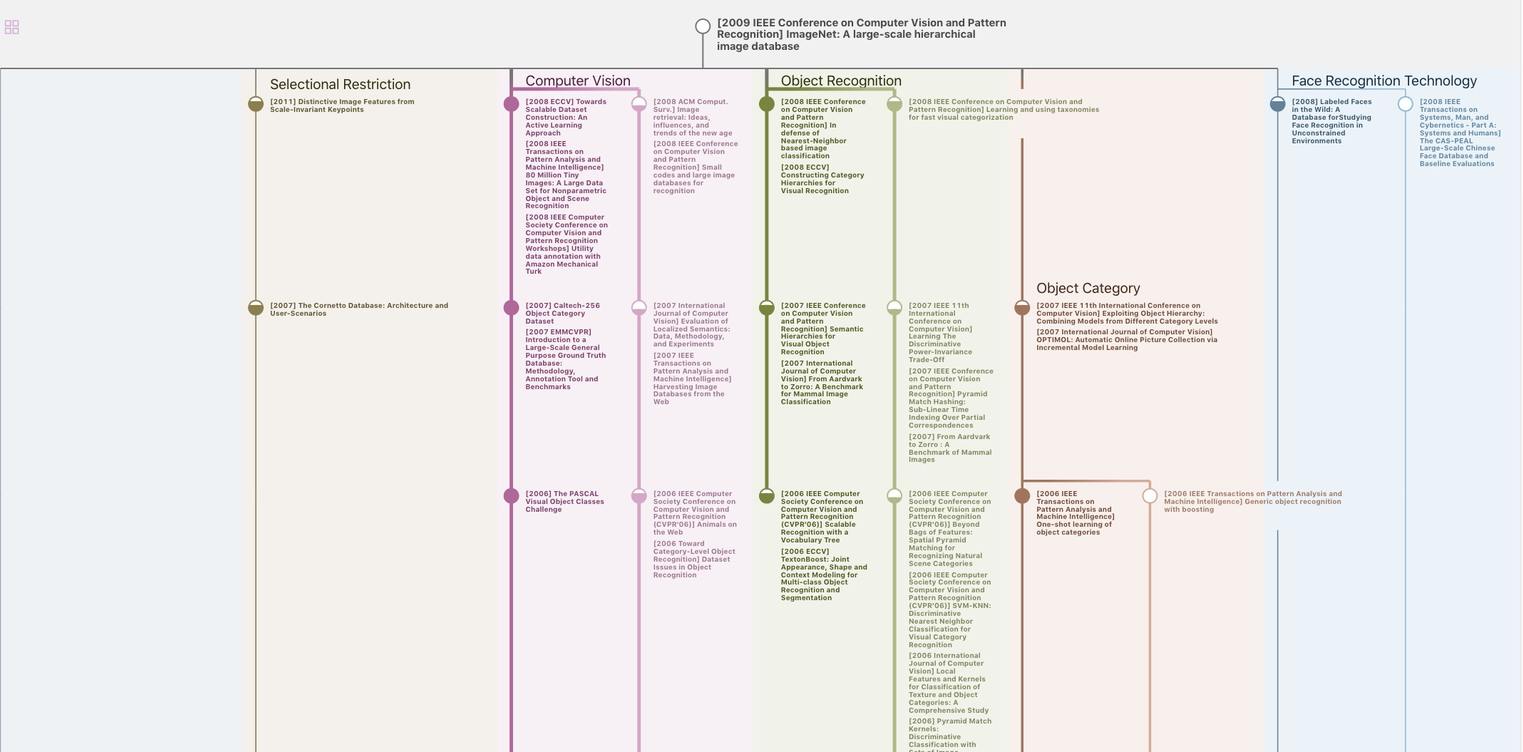
生成溯源树,研究论文发展脉络
Chat Paper
正在生成论文摘要