Minimized models and grammar-informed initialization for supertagging with highly ambiguous lexicons
ACL(2010)
摘要
We combine two complementary ideas for learning supertaggers from highly ambiguous lexicons: grammar-informed tag transitions and models minimized via integer programming. Each strategy on its own greatly improves performance over basic expectation-maximization training with a bitag Hidden Markov Model, which we show on the CCGbank and CCG-TUT corpora. The strategies provide further error reductions when combined. We describe a new two-stage integer programming strategy that efficiently deals with the high degree of ambiguity on these datasets while obtaining the full effect of model minimization.
更多查看译文
关键词
grammar-informed tag transition,error reduction,integer programming,complementary idea,ambiguous lexicon,ccg-tut corpus,grammar-informed initialization,basic expectation-maximization training,full effect,new two-stage integer programming,minimized model,bitag hidden markov model,hidden markov model,expectation maximization
AI 理解论文
溯源树
样例
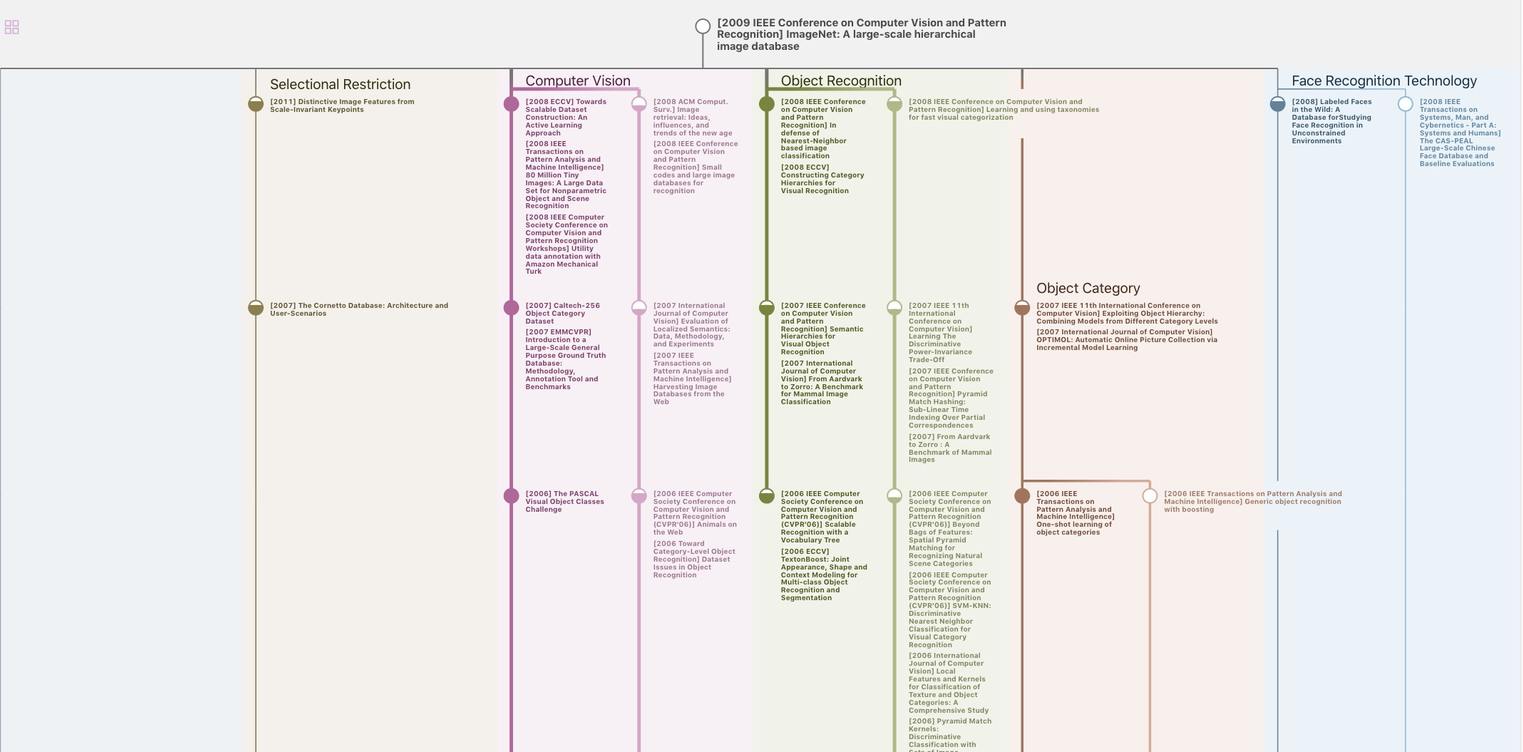
生成溯源树,研究论文发展脉络
Chat Paper
正在生成论文摘要