Recommending Inexperienced Products via Learning from Consumer Reviews
Web Intelligence(2012)
摘要
Most products in e-commerce are with high cost (e.g., digital cameras, computers) and hence less likely experienced by users (so they are called "inexperienced products"). The traditional recommender techniques (such as user-based collaborative filtering and content-based methods) are thus not effectively applicable in this environment, because they largely assume that the users have prior experiences with the items. In this paper, we have particularly incorporated product reviews to solve the recommendation problem. We first studied how to utilize the reviewer-level weighted feature preferences (as learnt from their written product reviews) to generate recommendations to the current buyer, followed by exploring the impact of Latent Class Regression Models (LCRM) based cluster-level feature preferences (that represent the common preferences of a group of reviewers). Motivated by their respective advantages, a hybrid method that combines both reviewer-level and cluster-level preferences is introduced and experimentally compared to the other methods. The results reveal that the hybrid method is superior to the other variations in terms of recommendation accuracy, especially when the current buyer states incomplete feature preferences.
更多查看译文
关键词
purchasing,hybrid method,pattern clustering,written product reviews,consumer reviews,lcrm based cluster-level feature preference,regression analysis,incomplete feature preferences,information retrieval,e-commerce,recommender systems,recommender system,product reviews,latent class regression model,recommendation problem,reviewer-level preferences,inexperienced products,electronic commerce,reviewer-level weighted feature preferences,recommendation accuracy,inexperienced product recommendation,weighted feature preferences
AI 理解论文
溯源树
样例
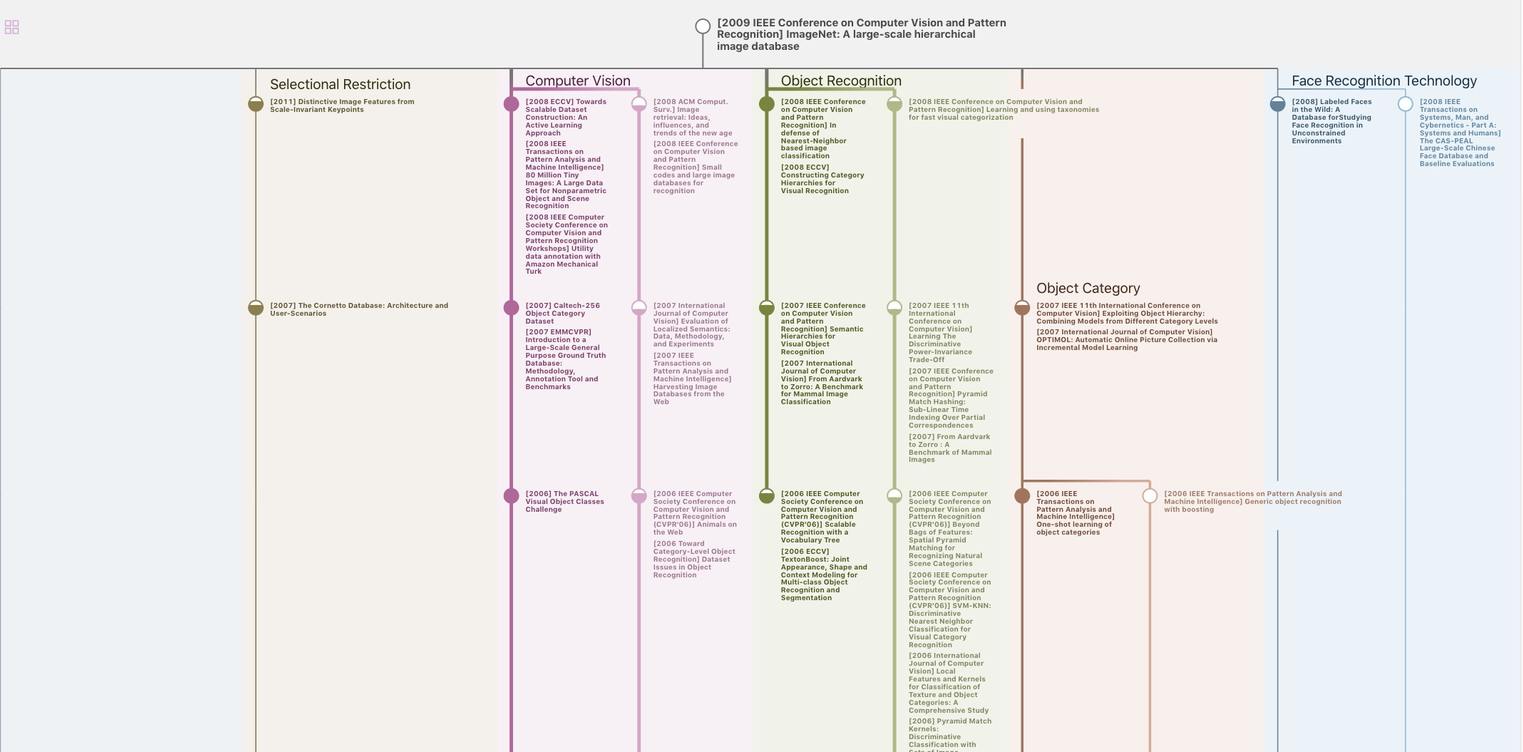
生成溯源树,研究论文发展脉络
Chat Paper
正在生成论文摘要