A Linear-Chain Crf-Based Learning Approach For Web Opinion Mining
WISE'10 Proceedings of the 11th international conference on Web information systems engineering(2010)
摘要
The task of opinion mining from product reviews is to extract the product entities and determine whether the opinions on the entities are positive, negative or neutral. Reasonable performance on this task has been achieved by employing rule-based, statistical approaches or generative learning models such as hidden Markov model (HMMs). In this paper, we proposed a discriminative model using linear-chain Conditional Random Field (CRFs) for opinion mining. CRFs can naturally incorporate arbitrary, non-independent features of the input without making conditional independence assumptions among the features. This can be particularly important for opinion mining on product reviews. We evaluated our approach base on three criteria: recall, precision and F-score for extracted entities, opinions and their polarities. Compared to other methods, our approach was proven more effective for accomplishing opinion mining tasks.
更多查看译文
关键词
Conditional Random Field (CRFs),Web Opinion Mining,Feature Function
AI 理解论文
溯源树
样例
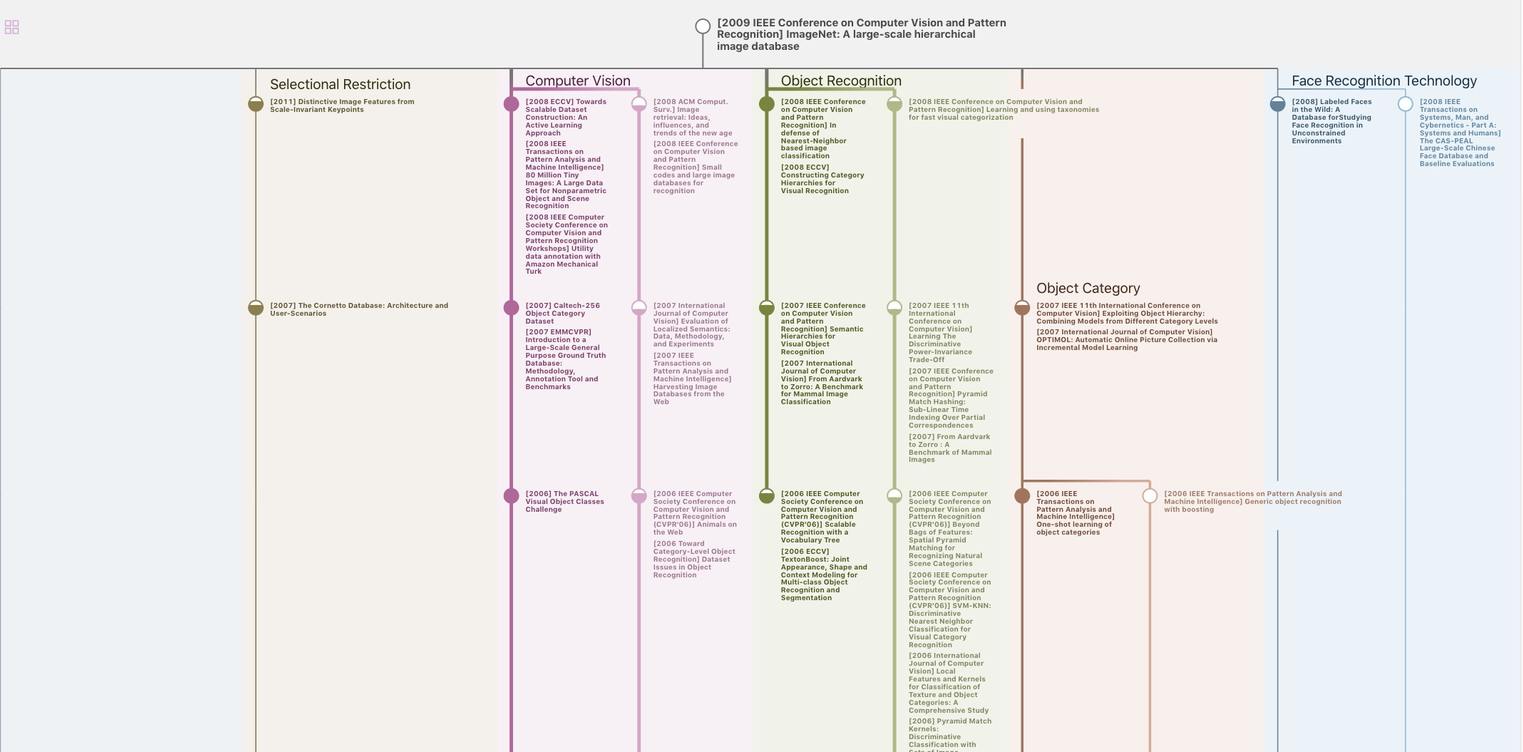
生成溯源树,研究论文发展脉络
Chat Paper
正在生成论文摘要