Money Never Sleeps: Maximizing Liquidity Mining Yields in Decentralized Finance
KDD 2024(2024)
Abstract
The popularity of decentralized finance has drawn attention to liquidity mining (LM). In LM, a user deposits her cryptocurrencies into liquidity pools to provide liquidity for exchanges and earn yields. Different liquidity pools offer varying yields and require different pairs of cryptocurrencies. A user can exchange a cryptocurrency for another with some exchange costs. Thus, an LM solution consists of exchange transactions and deposit transactions, guaranteeing (1) each exchange transaction must exchange one cryptocurrency for another at a specific rate (i.e., the exchange constraint); (2) the amounts of cryptocurrencies deposited in a liquidity pool must exceed the required threshold (i.e., the minimum constraint); (3) each deposit transaction must deposit a specific pair of cryptocurrencies at a certain rate in a liquidity pool (i.e., the deposit constraint); and (4) the cryptocurrencies used in the solution do not exceed the cryptocurrencies that the user has (i.e., the budget constraint). Selecting the most profitable LM solution is challenging due to the vast number of candidate solutions. To address this challenge, we define the yield maximization liquidity mining (YMLM) problem. Given a set of liquidity pools, a set of the user's cryptocurrencies, a set of exchange rates, and an evaluation function, YMLM aims to find an LM solution with maximal yields, satisfying the minimum, exchange, deposit, and budget constraints. We prove that YMLM is NP-hard and cannot be solved by algorithms with constant approximation ratios. To tackle YMLM, we propose two algorithms, namely YMLM\_GD and YMLM\_SK, with parameterized approximation ratios. Extensive experiments on both real and synthetic datasets show that our approaches outperform the baselines in yields.
MoreTranslated text
Key words
Decentralized Finance,Liquidity Mining,Yield Maximization,NP-hard Problem,Approximation Algorithms
AI Read Science
Must-Reading Tree
Example
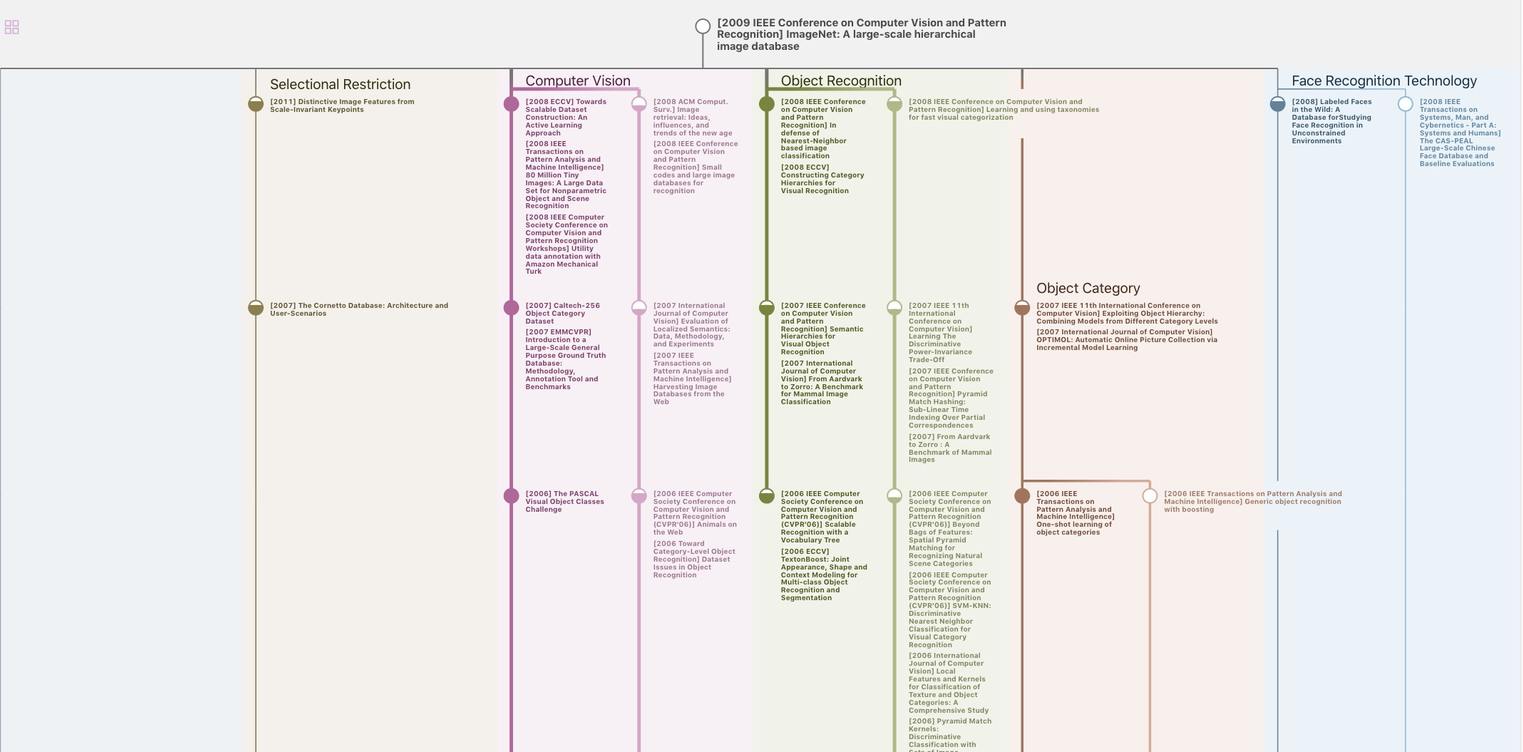
Generate MRT to find the research sequence of this paper
Chat Paper
Summary is being generated by the instructions you defined