Automated Detection of Shoulder Rotator Cuff Tendon Tears from Ultrasound Images by CNN-Autoencoder
2024 IEEE International Symposium on Biomedical Imaging (ISBI)(2024)
摘要
Ultrasound can effectively diagnose rotator cuff muscle tears in the shoulder, one of the most common musculoskeletal injuries. Expertise in performing and interpreting these scans is limited, and in some jurisdictions, it involves months-long wait times. This project aims to provide the core AI process for the assessment of shoulder injuries; specifically identifying full-thickness rotator cuff tears within minutes, rather than months. We propose a two-step approach starting with segmentation of the humeral cortex and subacromial bursa followed by the classification of tears based on these regions. Automatic segmentation using ultrasound is challenging due to speckle noise, low contrast, and artifacts. The proposed segmentation method leverages a CNN-autoencoder, that predicts the boundary contour points of the humeral cortex and subacromial bursa directly from raw images. Compared to an end-to-end black box classifier, our two-stage approach is more explainable and focuses on clinically relevant landmarks. The distinctive feature of this segmentation technique is, it predicts the segmentation contour points rather than the popular pixel-wise semantic segmentation. The study was performed on the dataset acquired from 206 patients. All the methods were trained using 10,080 images and evaluated using 2520 images. Our proposed segmentation method achieved an average Dice coefficient (DC) of 94.2% and a Hausdorff Distance (HD) of 2.8 mm, outperforming the U-Net model, which yielded 90.5% for the DC and 6.8 mm for the HD. After the segmentation, a classification network, VGG-16 achieved 81.0% accuracy (sensitivity 78.5%, specificity 76.2%) in classifying rotator cuff tendons as intact or torn from US images. AI-driven ultrasound for rotator cuff tear detection enhances early, accurate diagnosis and improves patient care, and this automated tool could be used by lightly trained users at initial point-of-care facilities like family physician clinics and emergency rooms.
更多查看译文
关键词
Shoulder ultrasound,rotator cuff,CNN,autoencoders,segmentation,classification
AI 理解论文
溯源树
样例
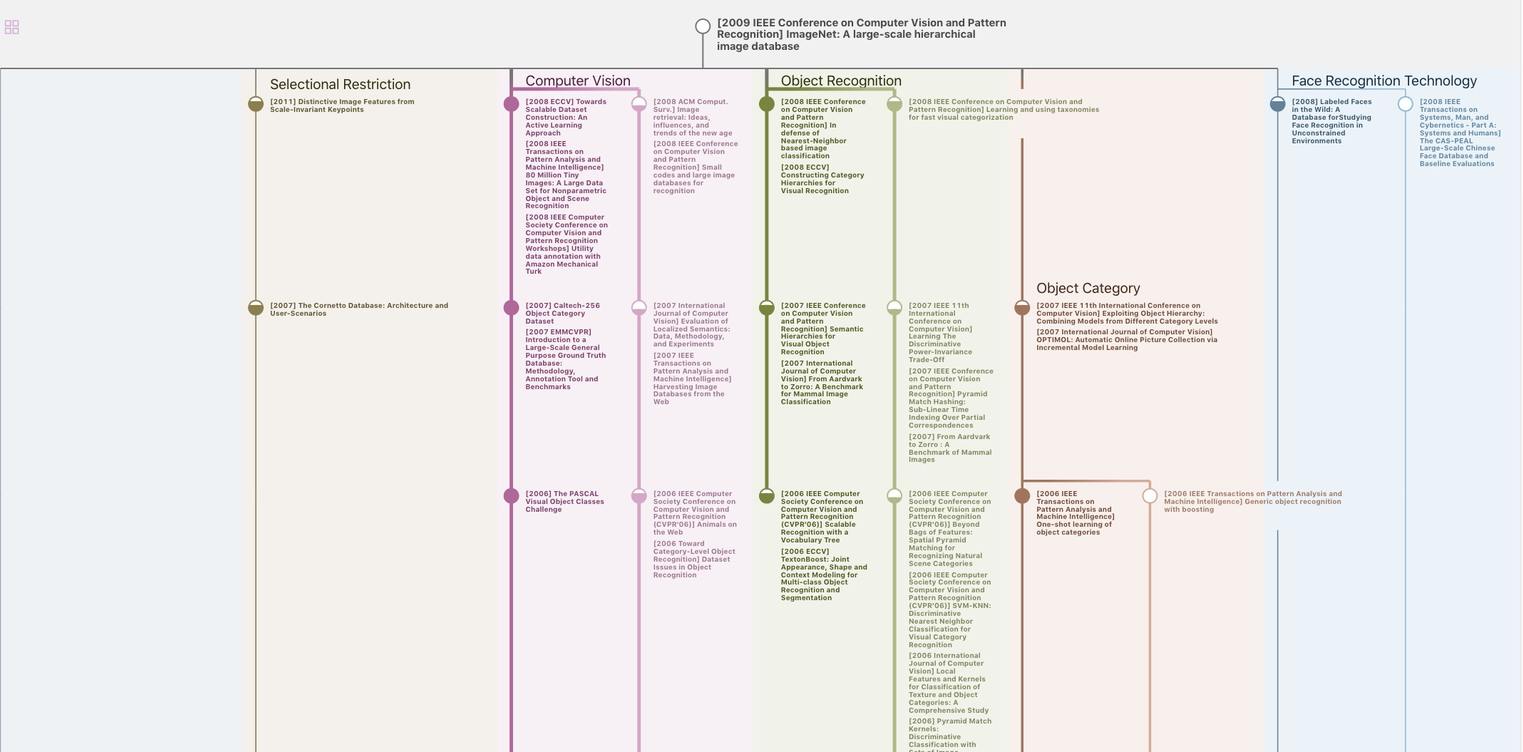
生成溯源树,研究论文发展脉络
Chat Paper
正在生成论文摘要