MV2DFusion: Leveraging Modality-Specific Object Semantics for Multi-Modal 3D Detection
arxiv(2024)
摘要
The rise of autonomous vehicles has significantly increased the demand for robust 3D object detection systems. While cameras and LiDAR sensors each offer unique advantages–cameras provide rich texture information and LiDAR offers precise 3D spatial data–relying on a single modality often leads to performance limitations. This paper introduces MV2DFusion, a multi-modal detection framework that integrates the strengths of both worlds through an advanced query-based fusion mechanism. By introducing an image query generator to align with image-specific attributes and a point cloud query generator, MV2DFusion effectively combines modality-specific object semantics without biasing toward one single modality. Then the sparse fusion process can be accomplished based on the valuable object semantics, ensuring efficient and accurate object detection across various scenarios. Our framework's flexibility allows it to integrate with any image and point cloud-based detectors, showcasing its adaptability and potential for future advancements. Extensive evaluations on the nuScenes and Argoverse2 datasets demonstrate that MV2DFusion achieves state-of-the-art performance, particularly excelling in long-range detection scenarios.
更多查看译文
AI 理解论文
溯源树
样例
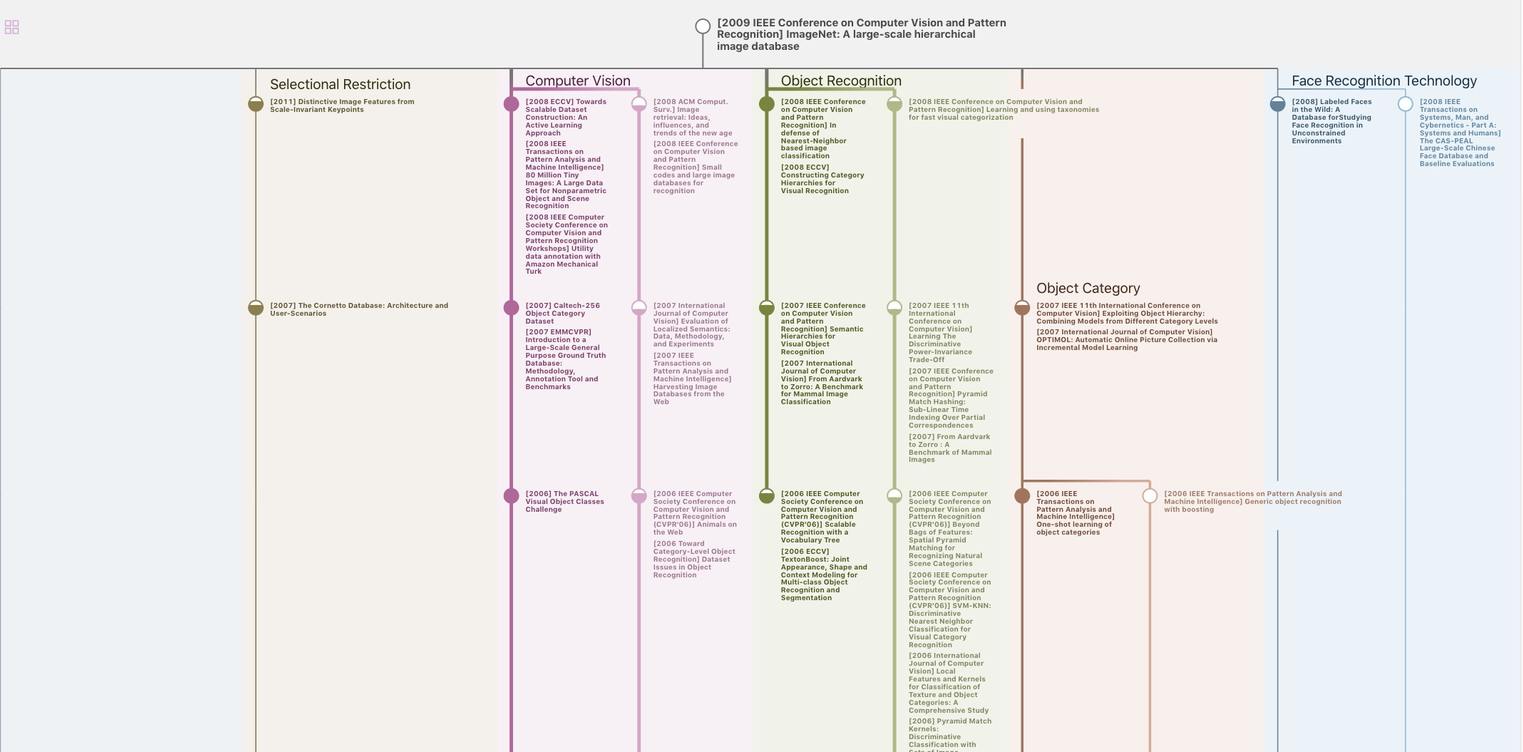
生成溯源树,研究论文发展脉络
Chat Paper
正在生成论文摘要