Measuring generalized divergence for multiple distributions with application to deep clustering
Pattern Recognition(2025)
摘要
In machine learning scenarios involving multiple sources (distributions) of data, such as multi-view learning, domain adaptation or generalization, and clustering, it is crucial to efficiently handle such multi-source data simultaneously while effectively identifying their dissimilarity. Traditional approaches for measuring the overall divergence among multiple distributions involve calculating the divergence between each pair of distributions using a two-distribution-compare measure and then quantifying their average. However, this approach risks disregarding the collective synergy or overlap that may exist among more than two distributions and also incurs substantial computational costs. To address these limitations, we propose the use of the generalized Jensen–Rényi divergence (GJRD), to facilitate the simultaneous handling of multiple distributions. We derive a closed-form non-parametric empirical estimator for the GJRD based on kernel density estimation, making it convenient for data-driven machine learning applications. Further, we develop the GJRD-based deep clustering framework (GJRD-DC) and the corresponding algorithms, leveraging the derived estimator. Experimental results on various benchmarks demonstrate that the proposed GJRD-DC method achieves state-of-the-art performance on challenging datasets and comparable results on others. Code is available at https://github.com/LMFLRB/GJRD.git
更多查看译文
关键词
Generalized divergence measures,Sample-based estimation,Jensen–Rényi divergence,Deep clustering
AI 理解论文
溯源树
样例
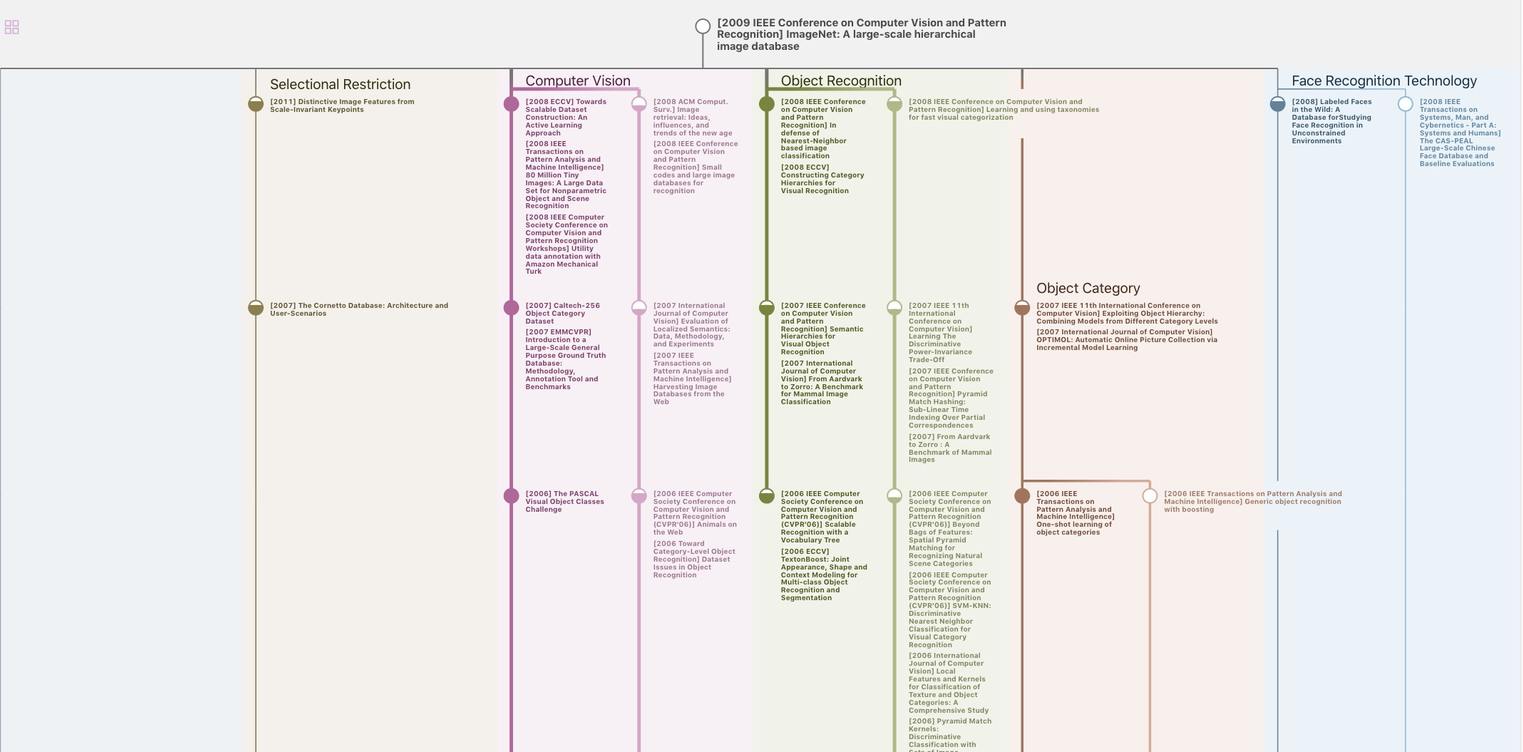
生成溯源树,研究论文发展脉络
Chat Paper
正在生成论文摘要