SleepSatelightFTC: A Lightweight and Interpretable Deep Learning Model for Single-Channel EEG-Based Sleep Stage Classification
biorxiv(2024)
Abstract
Sleep scoring by experts is necessary for diagnosing sleep disorders. To this end, electroencephalography (EEG) is an essential physiological examination. As manual sleep scoring based on EEG signals is time-consuming and labor-intensive, an automated method is highly desired. One promising automation technology is deep learning, which has performed well or better than experts in sleep scoring. However, deep learning lacks adequate interpretability, which is crucial for ensuring safety and accountability, especially for complex inference processes. We propose SleepSatelightFTC, a model that employs self-attention to visualize feature importance for inference and transfer learning on continuous epoch data to reflect the inference context. This model achieves a higher accuracy (84.8%) and kappa coefficient (0.787) with fewer parameters than state-of-the-art models for sleep stage classification on the 2018 version of the Sleep-EDF Database Expanded. The visualization of feature importance obtained from self-attention confirms that the proposed model learns representative waveform features, including K-complexes and sleep spindles.
### Competing Interest Statement
The authors have declared no competing interest.
MoreTranslated text
AI Read Science
Must-Reading Tree
Example
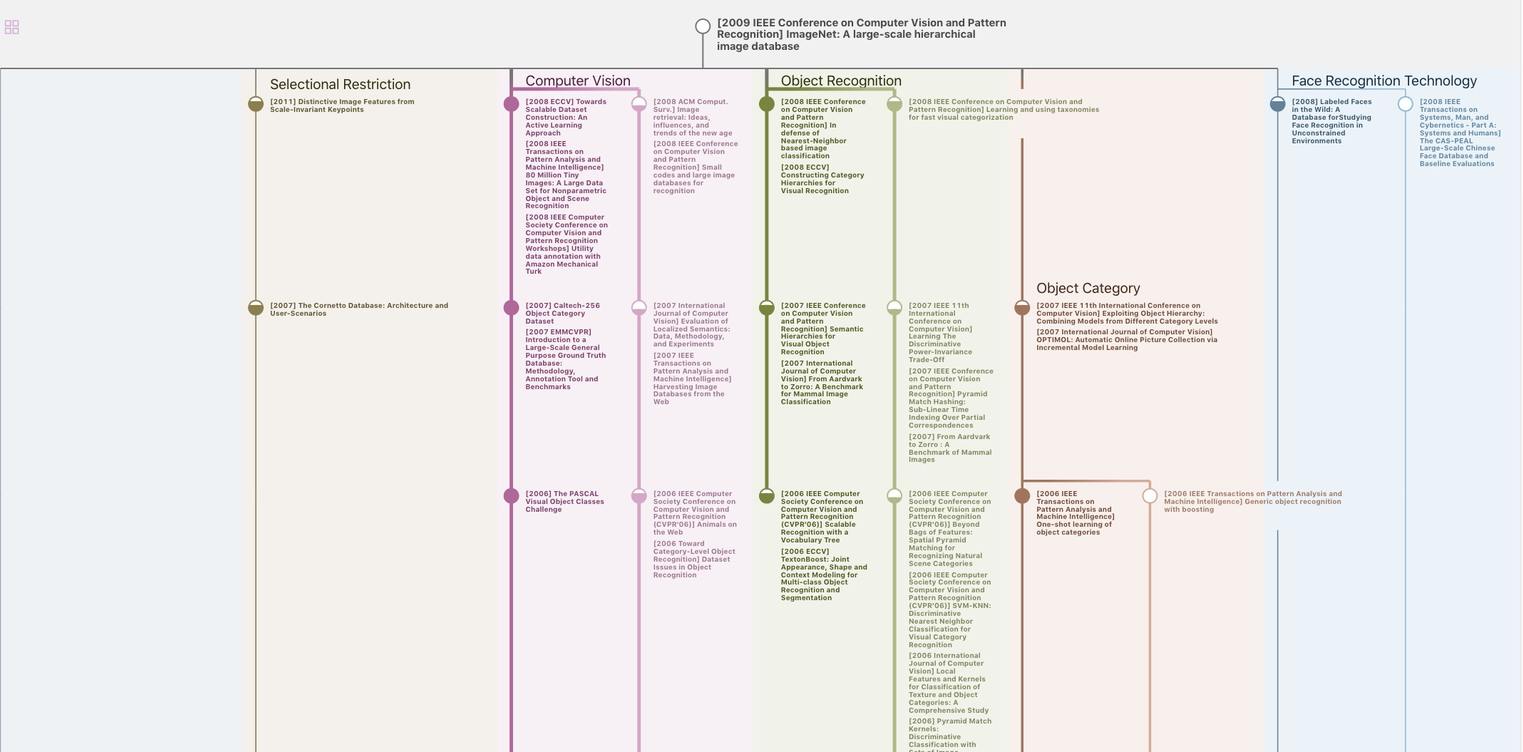
Generate MRT to find the research sequence of this paper
Chat Paper
Summary is being generated by the instructions you defined