Quantitative Pixel-Level Segmentation and 3D Reconstruction of Concealed Cracks in Asphalt Pavements
IEEE Transactions on Intelligent Transportation Systems(2024)
Abstract
Detecting concealed cracks before they become reflective cracks is crucial for the proper design and maintenance of roads. However, research on quantitative recognition and intuitive three-dimensional (3D) characterization of concealed cracks is still at an exploratory stage, so this paper proposes a pixel-level segmentation network based on the You Only Look Once for Panoptic (YOLOP) enhancement, which can quantitatively segment the distresses while performing pixel-level detection of concealed cracks in asphalt pavements. Firstly, gprMax was used to numerically simulate and summarize the characteristics of internal cracks in ground-penetrating radar (GPR) images. The relationship between the actual area with crack characteristics and the occupied pixel area in GPR images was then established. The improved YOLOP was utilized to enhance the detection speed and accuracy of concealed cracks, and in terms of a single index, the R-index increased the most by 40%, the P-index increased the least by 26% and the F1-score increased by 37%. Additionally, the segmented crack region was reconstructed in three dimensions using the computed tomography (CT) imaging principle. The vertical height, longitudinal length, cross-sectional area, and volume of the crack region were estimated. This thesis presents important research on the accurate detection and intuitive characterization of concealed cracks in asphalt pavements. It can serve as a theoretical guide for practical engineering applications.
MoreTranslated text
Key words
Ground penetrating radar (GPR),concealed cracks,asphalt pavement,quantitative segmentation,3D reconstruction
AI Read Science
Must-Reading Tree
Example
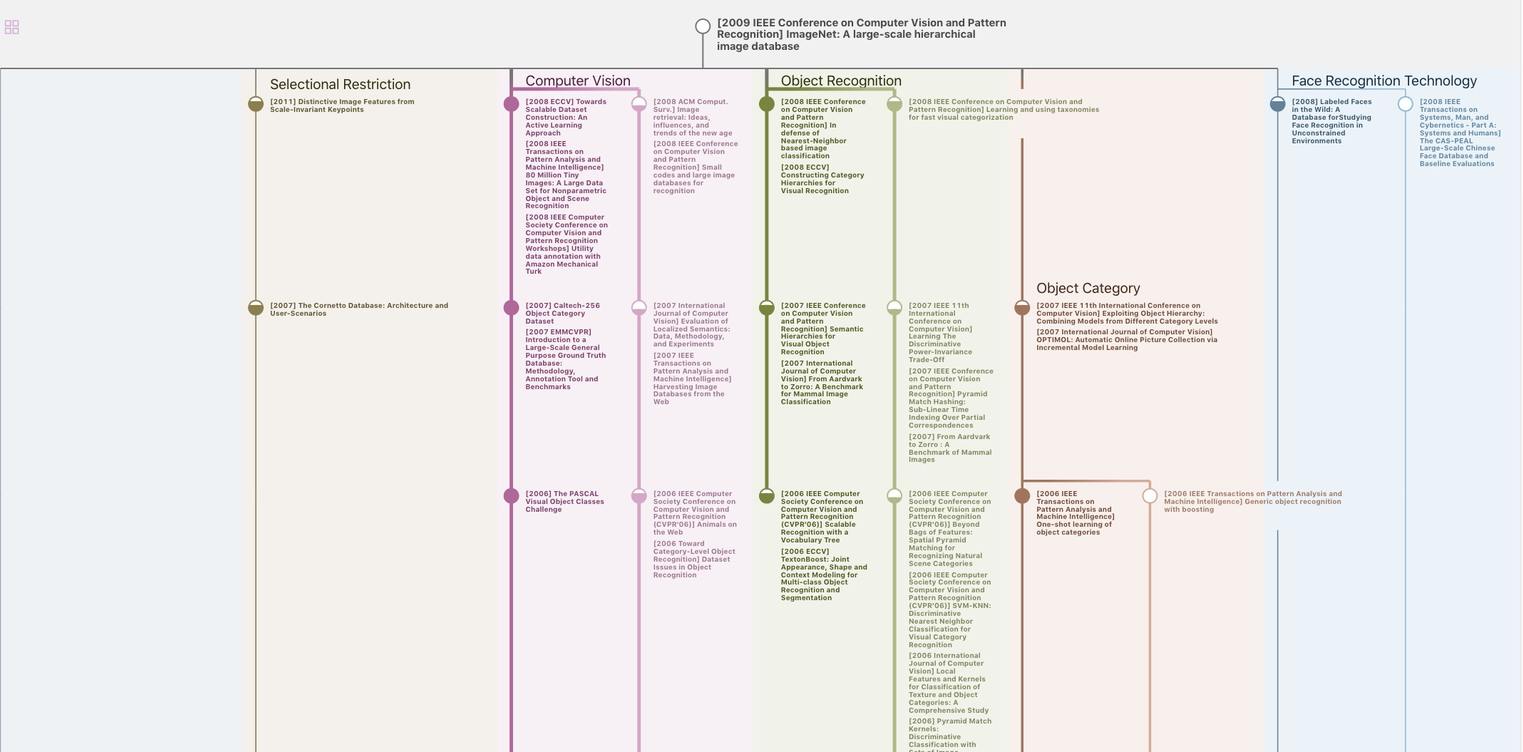
Generate MRT to find the research sequence of this paper
Chat Paper
Summary is being generated by the instructions you defined