Building Change Detection in Earthquake: A Multi-Scale Interaction Network with Offset Calibration and A Dataset
IEEE TRANSACTIONS ON GEOSCIENCE AND REMOTE SENSING(2024)
Key words
Buildings,Earthquakes,Feature extraction,Imaging,Calibration,Attention mechanisms,Remote sensing,CD dataset,change detection (CD),deep learning,offset calibration
AI Read Science
Must-Reading Tree
Example
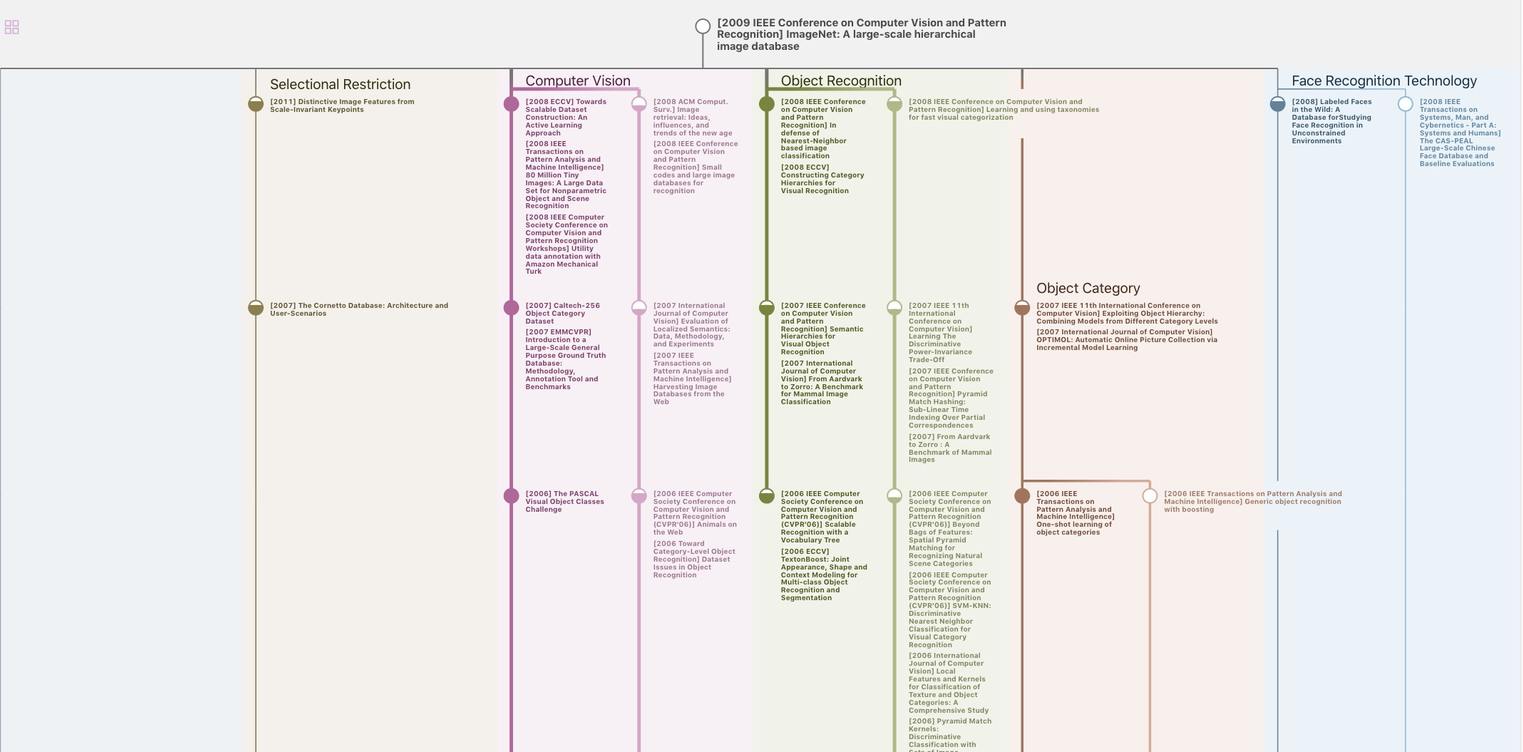
Generate MRT to find the research sequence of this paper
Chat Paper
Summary is being generated by the instructions you defined