Machine learning-accelerated inverse design of programmable bi-functional metamaterials
Composite Structures(2024)
摘要
Bi-functional metamaterials with programmable coefficients of thermal expansion (CTEs) and Poisson’s ratios (PRs) have garnered significant attention among researchers due to the ability to manifest desired deformations under thermal and mechanical loads. Nevertheless, a current challenge lies in efficiently achieving the inverse design of these metamaterials to meet diverse application requirements. This paper presents a machine learning (ML) model that can establish a logical mapping relationship between geometric/material parameters and mechanical properties, and it is applied to the inverse design of bi-functional metamaterials with desired CTEs and PRs. Furthermore, the inverse design capability of the ML model was validated by the finite element analysis and experimental test. The results demonstrate that the geometric models obtained from the inverse prediction can effectively exhibit the desired deformation behavior under thermal and mechanical loads. And the ML model proves to be a valuable tool, offering effective guidance for the design of bi-functional metamaterials with specific CTEs and PRs.
更多查看译文
关键词
Bi-functional metamaterials,Machine learning,Thermal expansion,Poisson’s ratio,Inverse design
AI 理解论文
溯源树
样例
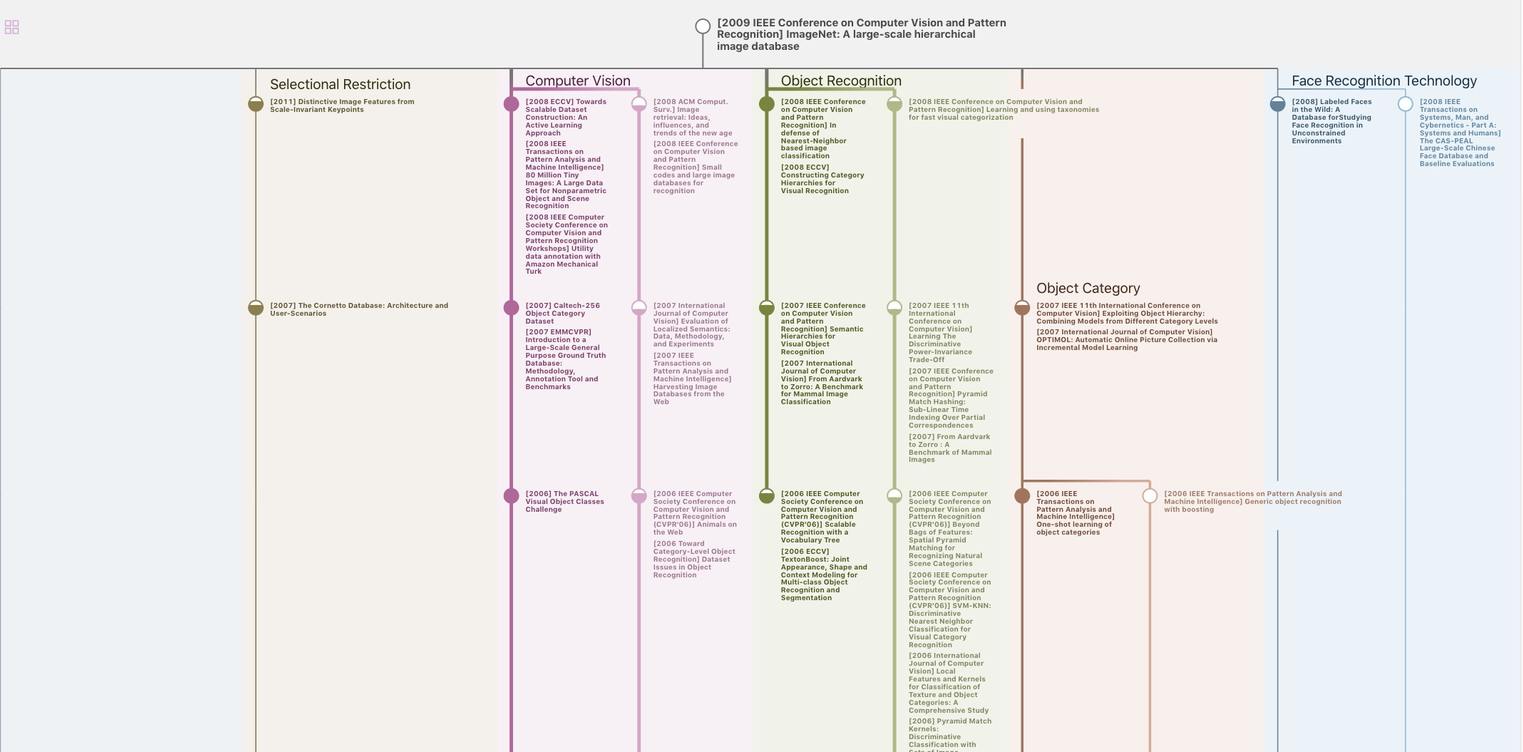
生成溯源树,研究论文发展脉络
Chat Paper
正在生成论文摘要