Interpretable machine learning models for predicting the bond strength between UHPC and normal-strength concrete
Materials Today Communications(2024)
摘要
Ultra-high-performance concrete (UHPC) boasts superior mechanical properties and durability, rendering it a viable material to repair and reinforce pre-existing concrete structures. The complexity of the bond behavior between UHPC and normal-strength concrete (NC) presents a challenge to make precise predictions of the interfacial bond strength. This study employed four machine learning algorithms, namely artificial neural network, random forest, adaptive boosting, and categorical gradient boosting, to predict the interfacial bond strength of UHPC-NC. A database of 95 samples of slant shear tests was collected from existing literature. The input variables for modeling include joint angle, compressive strength of NC and UHPC, surface treatment, interfacial moisture, curing age, and curing method. The impact of each feature on the slant shear bond strength was assessed using SHapley Additive exPlanations and Partial Dependence Plots. Results show that the categorical gradient boosting model performed the best on the testing set, achieving an R2 of 0.948, RMSE of 1.408, and MAE of 1.028. The accuracy of all four machine learning models surpassed that of two empirical models (AASHTO LRFD 2014 and Eurocode 2). The top three features influencing the interfacial bond strength were identified as the surface treatment, joint angle, and compressive strength of NC, while the impact of the curing method and the interfacial moisture were comparatively limited. The proposed machine learning model exhibits potential as a valuable tool for assisting structural retrofitting and rehabilitation with UHPC.
更多查看译文
关键词
Interface bond strength,Ultra-high-performance concrete,Normal-strength concrete,Machine learning,Model interpretability
AI 理解论文
溯源树
样例
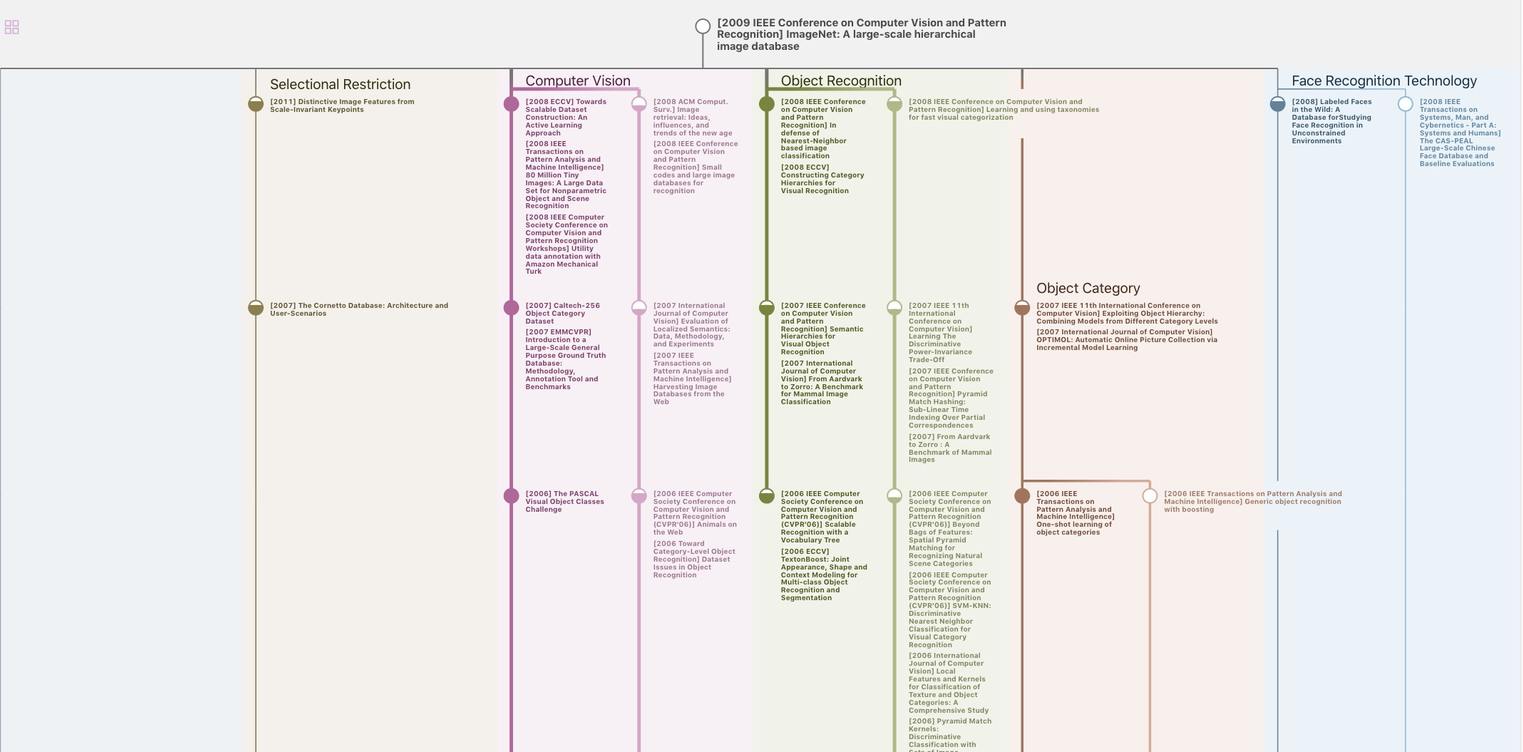
生成溯源树,研究论文发展脉络
Chat Paper
正在生成论文摘要