Enhancing cardiovascular risk assessment with advanced data balancing and domain knowledge-driven explainability
Expert Systems with Applications(2024)
摘要
In medical risk prediction, such as predicting heart disease, machine learning (ML) classifiers must achieve high accuracy, precision, and recall to minimize the chances of incorrect diagnoses or treatment recommendations. However, real-world datasets often have imbalanced data, which can affect classifier performance. Traditional data balancing methods can lead to overfitting and underfitting, making it difficult to identify potential health risks accurately. Early prediction of heart attacks is of paramount importance, and researchers have developed ML-based systems to address this problem. However, much of the existing ML research is based on a single dataset, often ignoring performance evaluation across multiple datasets. As the demand for interpretable ML models grows, model interpretability becomes central to revealing insights and feature effects within predictive models. To address these challenges, we present a novel data balancing technique that uses a divide-and-conquer strategy with the K-Means clustering algorithm to segment the dataset. The performance of our approach is highlighted through comparisons with established techniques, which demonstrate the superiority of our proposed method. To address the challenge of inter-dataset discrepancies, we use two different datasets. Our holistic pipeline, strengthened by the innovative balancing technique, effectively addresses performance discrepancies, culminating in a significant improvement from 81% to 90%. Furthermore, through advanced statistical analysis, it has been determined that the 95% confidence interval for the AUC metric of our method ranges from 0.8187 to 0.8411. This observation serves to underscore the consistency and reliability of our approach, demonstrating its ability to achieve high performance across a range of scenarios. Incorporating Explainable AI (XAI), we examine the feature rankings and their contributions within the best performing Random Forest model. While the domain expert feedback is consistent with the explanatory power of XAI, some differences remain. Nevertheless, a remarkable convergence in feature ranking and weighting is observed, bridging the insights from XAI tools and domain expert perspectives.
更多查看译文
关键词
Heart disease risk,Data balancing,Performance discrepancy,Explainability,Expert system,Domain knowledge
AI 理解论文
溯源树
样例
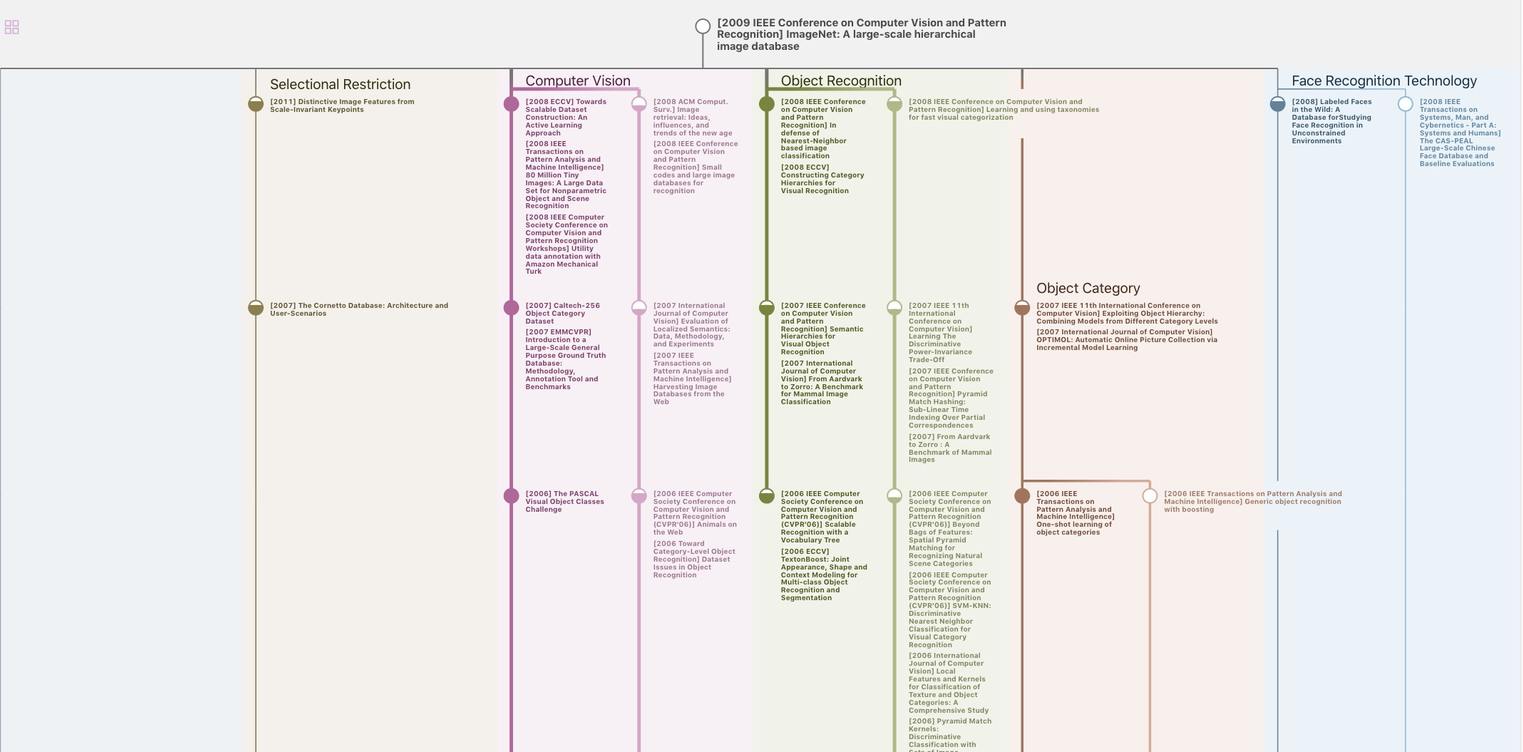
生成溯源树,研究论文发展脉络
Chat Paper
正在生成论文摘要