Identifying digital biomarkers of illness activity and treatment response in bipolar disorder with a novel wearable device (TIMEBASE): protocol for a pragmatic observational clinical study
BJPsych Open(2024)
Abstract
Background Bipolar disorder is highly prevalent and consists of biphasic recurrent mood episodes of mania and depression, which translate into altered mood, sleep and activity alongside their physiological expressions. Aims The IdenTifying dIgital bioMarkers of illnEss activity and treatment response in BipolAr diSordEr with a novel wearable device (TIMEBASE) project aims to identify digital biomarkers of illness activity and treatment response in bipolar disorder. Method We designed a longitudinal observational study including 84 individuals. Group A comprises people with acute episode of mania (n = 12), depression (n = 12 with bipolar disorder and n = 12 with major depressive disorder (MDD)) and bipolar disorder with mixed features (n = 12). Physiological data will be recorded during 48 h with a research-grade wearable (Empatica E4) across four consecutive time points (acute, response, remission and episode recovery). Group B comprises 12 people with euthymic bipolar disorder and 12 with MDD, and group C comprises 12 healthy controls who will be recorded cross-sectionally. Psychopathological symptoms, disease severity, functioning and physical activity will be assessed with standardised psychometric scales. Physiological data will include acceleration, temperature, blood volume pulse, heart rate and electrodermal activity. Machine learning models will be developed to link physiological data to illness activity and treatment response. Generalisation performance will be tested in data from unseen patients. Results Recruitment is ongoing. Conclusions This project should contribute to understanding the pathophysiology of affective disorders. The potential digital biomarkers of illness activity and treatment response in bipolar disorder could be implemented in a real-world clinical setting for clinical monitoring and identification of prodromal symptoms. This would allow early intervention and prevention of affective relapses, as well as personalisation of treatment.
MoreTranslated text
Key words
Bipolar disorder,mania,depression,physiological data,digital biomarkers
AI Read Science
Must-Reading Tree
Example
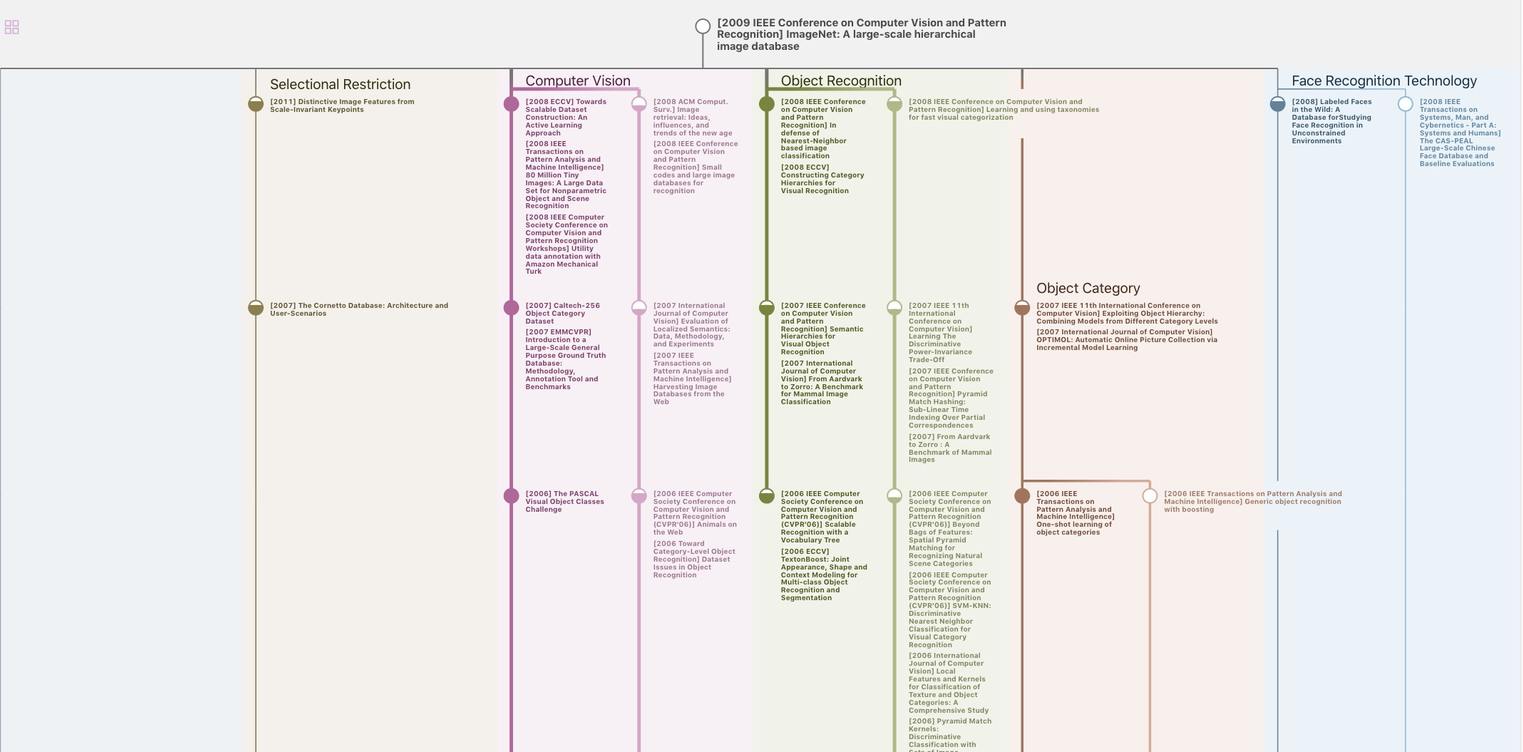
Generate MRT to find the research sequence of this paper
Chat Paper
Summary is being generated by the instructions you defined